COMET: A Neural Framework for MT Evaluation
Ricardo Rei, Craig Stewart, Ana C Farinha, Alon Lavie
Machine Translation and Multilinguality Long Paper
You can open the pre-recorded video in a separate window.
Abstract:
We present COMET, a neural framework for training multilingual machine translation evaluation models which obtains new state-of-the-art levels of correlation with human judgements. Our framework leverages recent breakthroughs in cross-lingual pretrained language modeling resulting in highly multilingual and adaptable MT evaluation models that exploit information from both the source input and a target-language reference translation in order to more accurately predict MT quality. To showcase our framework, we train three models with different types of human judgements: Direct Assessments, Human-mediated Translation Edit Rate and Multidimensional Quality Metric. Our models achieve new state-of-the-art performance on the WMT 2019 Metrics shared task and demonstrate robustness to high-performing systems.
NOTE: Video may display a random order of authors.
Correct author list is at the top of this page.
Connected Papers in EMNLP2020
Similar Papers
On the Sparsity of Neural Machine Translation Models
Yong Wang, Longyue Wang, Victor Li, Zhaopeng Tu,

MAD-X: An Adapter-Based Framework for Multi-Task Cross-Lingual Transfer
Jonas Pfeiffer, Ivan Vulić, Iryna Gurevych, Sebastian Ruder,

XL-WiC: A Multilingual Benchmark for Evaluating Semantic Contextualization
Alessandro Raganato, Tommaso Pasini, Jose Camacho-Collados, Mohammad Taher Pilehvar,
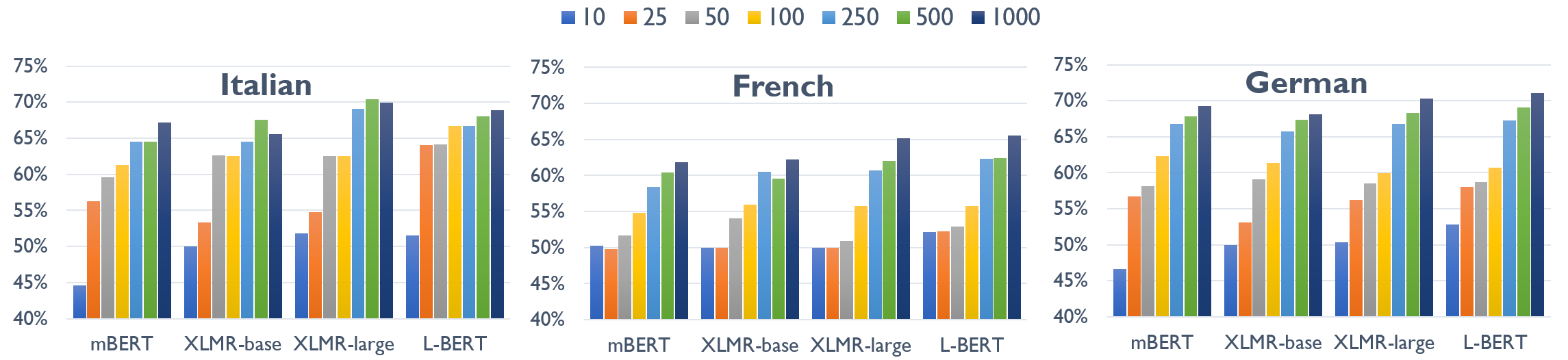