Multilingual AMR-to-Text Generation
Angela Fan, Claire Gardent
Language Generation Long Paper
You can open the pre-recorded video in a separate window.
Abstract:
Generating text from structured data is challenging because it requires bridging the gap between (i) structure and natural language (NL) and (ii) semantically underspecified input and fully specified NL output. Multilingual generation brings in an additional challenge: that of generating into languages with varied word order and morphological properties. In this work, we focus on Abstract Meaning Representations (AMRs) as structured input, where previous research has overwhelmingly focused on generating only into English. We leverage advances in cross-lingual embeddings, pretraining, and multilingual models to create multilingual AMR-to-text models that generate in twenty one different languages. Our multilingual models surpass baselines that generate into one language in eighteen languages, based on automatic metrics. We analyze the ability of our multilingual models to accurately capture morphology and word order using human evaluation, and find that native speakers judge our generations to be fluent.
NOTE: Video may display a random order of authors.
Correct author list is at the top of this page.
Connected Papers in EMNLP2020
Similar Papers
Making Monolingual Sentence Embeddings Multilingual using Knowledge Distillation
Nils Reimers, Iryna Gurevych,
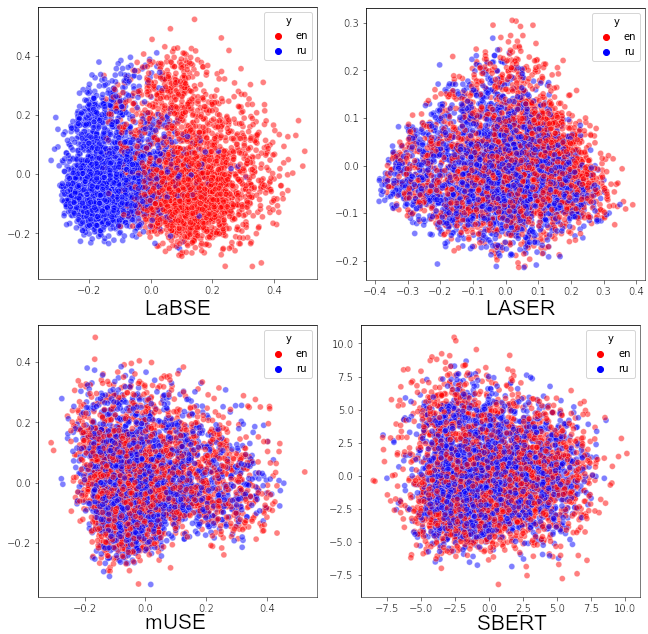
End-to-End Slot Alignment and Recognition for Cross-Lingual NLU
Weijia Xu, Batool Haider, Saab Mansour,

XL-AMR: Enabling Cross-Lingual AMR Parsing with Transfer Learning Techniques
Rexhina Blloshmi, Rocco Tripodi, Roberto Navigli,
