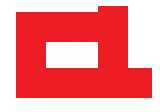
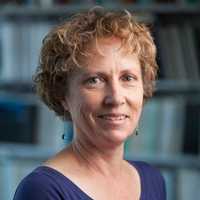
In this talk, I'll examine the state of the NLP subfield of information extraction from its inception almost 30 years ago to its current realization in neural network models. Which aspects of the original formulation of the task are more or less solved? In what ways are current state-of-the-art methods still falling short? What's up next for information extraction?
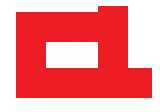
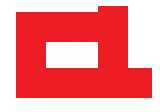
Publishing in an era of Responsible AI: How can NLP be proactive? Considerations and Implications. Moderated by Mona Diab.
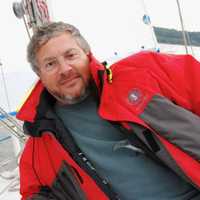
In machine learning sometimes tradeoffs must be made between accuracy and intelligibility: the most accurate models usually are not very intelligible, and the most intelligible models usually are less accurate. This can limit the accuracy of models that can safely be deployed in mission-critical applications where being able to understand, validate, edit, and ultimately trust a model is important. We have been working on a learning method to escape this tradeoff that is as accurate as full complexity models such as boosted trees and random forests, but more intelligible than linear models. This makes it easy to understand what the model has learned and to edit the model when it learns inappropriate things. Making it possible for humans to understand and repair a model is critical because most training data has unexpected problems. I’ll present several case studies where these high-accuracy GAMs discover surprising patterns in the data that would have made deploying a black-box model inappropriate. I’ll also show how these models can be used to detect and correct bias. And if there’s time, I’ll briefly discuss using intelligible GAM models to predict COVID-19 mortality.
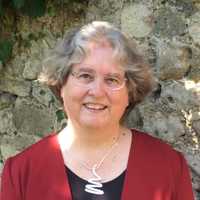
To evaluate the performance of NLP systems, the standard is to use held-out test data. When the systems are deployed in real-world applications, they will only be successful if they perform well on examples that their architects never saw before. Many of these will be examples that nobody ever saw before; the central observation of generative linguistics, going back to von Humboldt, is that human language involves "The infinite use of finite means". Predicting the real-world success of NLP systems thus comes down to predicting future human linguistic behaviour. In this talk, I will discuss some general characteristics of human linguistic behaviour, and the extent to which they are, or are not addressed in current NLP methodology. The topics I will address include: look-ahead and prediction; the role of categorization in building abstractions; effects of context; and variability across individuals.
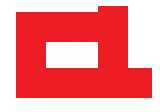
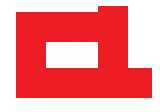