On the Sparsity of Neural Machine Translation Models
Yong Wang, Longyue Wang, Victor Li, Zhaopeng Tu
Machine Translation and Multilinguality Short Paper
You can open the pre-recorded video in a separate window.
Abstract:
Modern neural machine translation (NMT) models employ a large number of parameters, which leads to serious over-parameterization and typically causes the underutilization of computational resources. In response to this problem, we empirically investigate whether the redundant parameters can be reused to achieve better performance. Experiments and analyses are systematically conducted on different datasets and NMT architectures. We show that: 1) the pruned parameters can be rejuvenated to improve the baseline model by up to +0.8 BLEU points; 2) the rejuvenated parameters are reallocated to enhance the ability of modeling low-level lexical information.
NOTE: Video may display a random order of authors.
Correct author list is at the top of this page.
Connected Papers in EMNLP2020
Similar Papers
Language Model Prior for Low-Resource Neural Machine Translation
Christos Baziotis, Barry Haddow, Alexandra Birch,
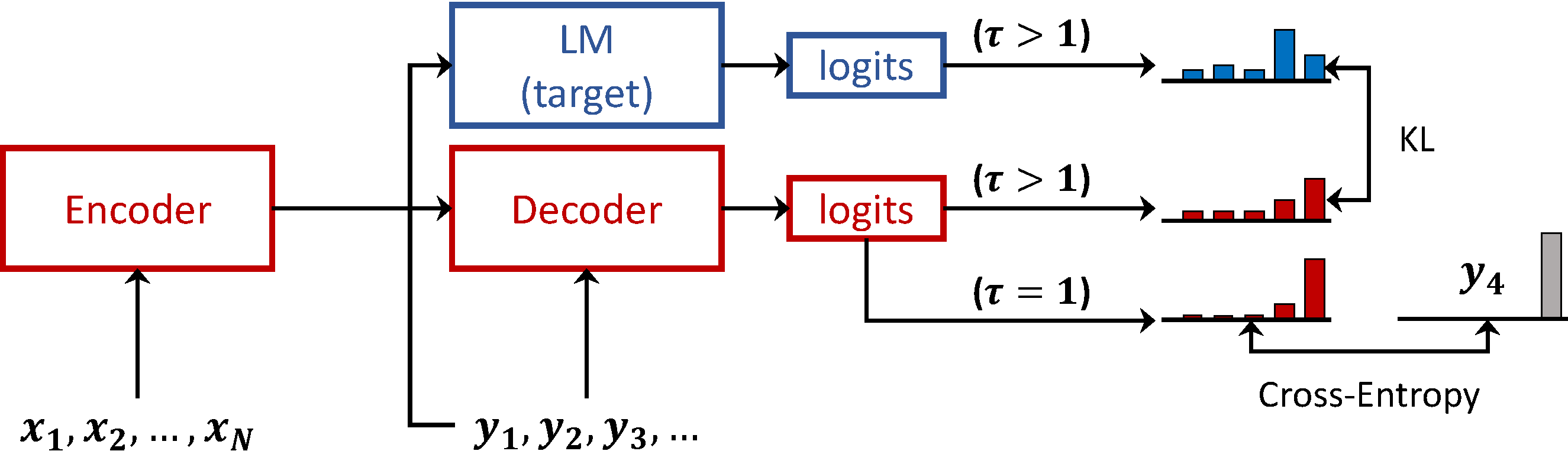
On the importance of pre-training data volume for compact language models
Vincent Micheli, Martin d'Hoffschmidt, François Fleuret,

Distilling Multiple Domains for Neural Machine Translation
Anna Currey, Prashant Mathur, Georgiana Dinu,
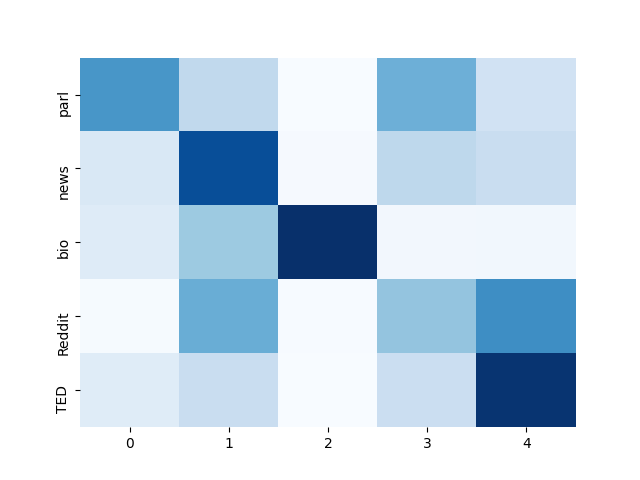