A Simple and Effective Model for Answering Multi-span Questions
Elad Segal, Avia Efrat, Mor Shoham, Amir Globerson, Jonathan Berant
Question Answering Short Paper
Abstract:
Models for reading comprehension (RC) commonly restrict their output space to the set of all single contiguous spans from the input, in order to alleviate the learning problem and avoid the need for a model that generates text explicitly. However, forcing an answer to be a single span can be restrictive, and some recent datasets also include multi-span questions, i.e., questions whose answer is a set of non-contiguous spans in the text. Naturally, models that return single spans cannot answer these questions. In this work, we propose a simple architecture for answering multi-span questions by casting the task as a sequence tagging problem, namely, predicting for each input token whether it should be part of the output or not. Our model substantially improves performance on span extraction questions from DROP and Quoref by 9.9 and 5.5 EM points respectively.
Connected Papers in EMNLP2020
Similar Papers
IIRC: A Dataset of Incomplete Information Reading Comprehension Questions
James Ferguson, Matt Gardner, Hannaneh Hajishirzi, Tushar Khot, Pradeep Dasigi,
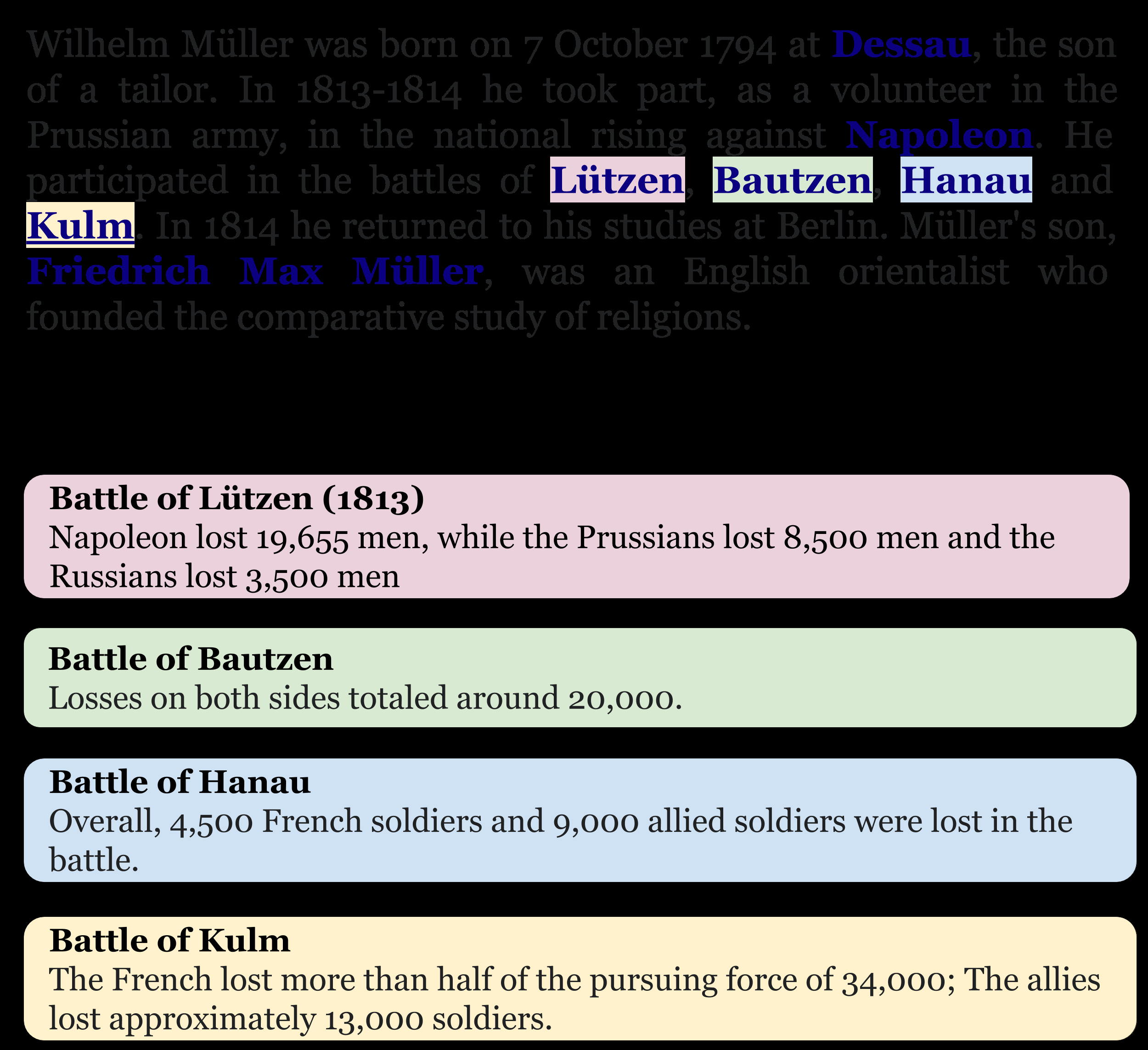
Context-Aware Answer Extraction in Question Answering
Yeon Seonwoo, Ji-Hoon Kim, Jung-Woo Ha, Alice Oh,
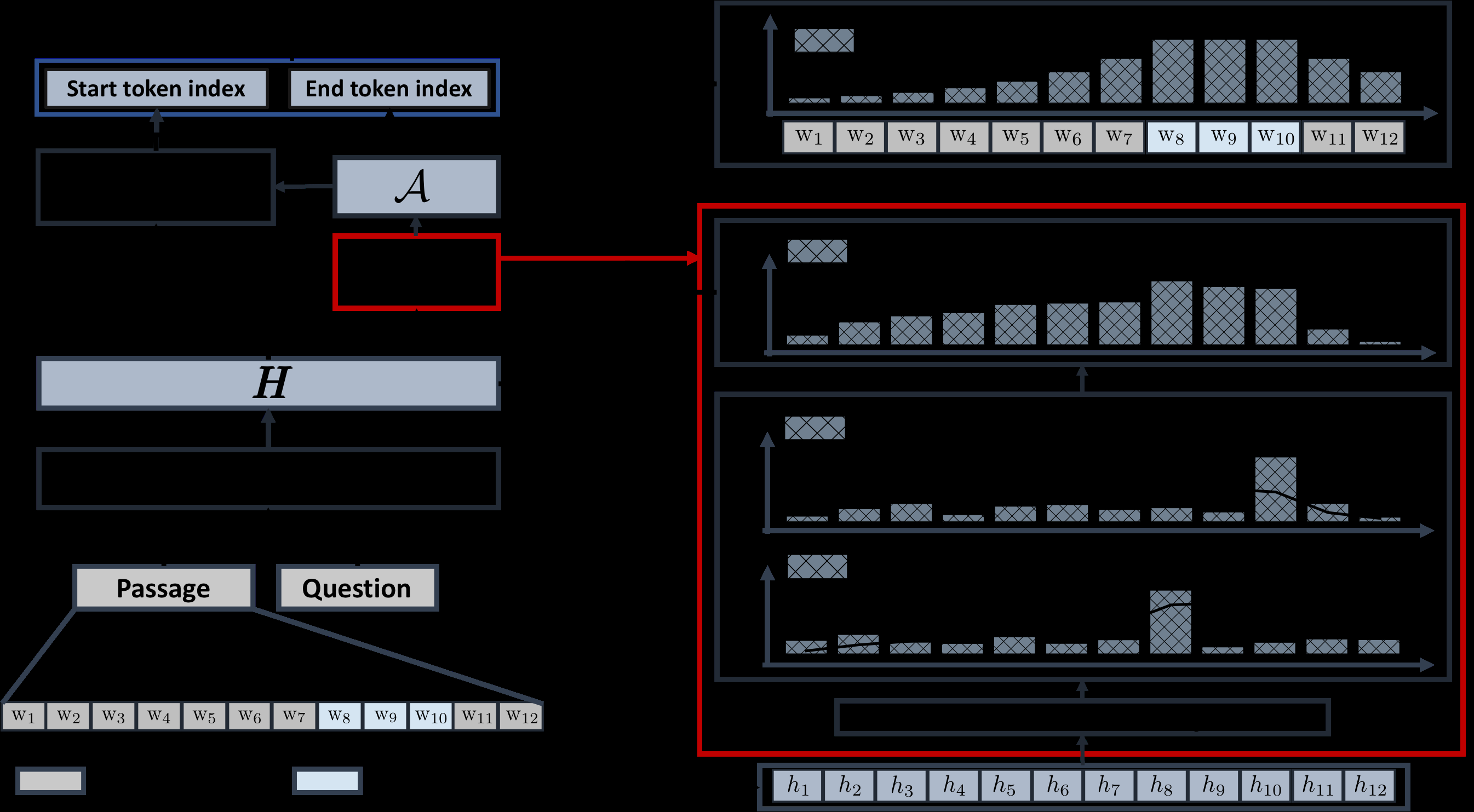
Training Question Answering Models From Synthetic Data
Raul Puri, Ryan Spring, Mohammad Shoeybi, Mostofa Patwary, Bryan Catanzaro,
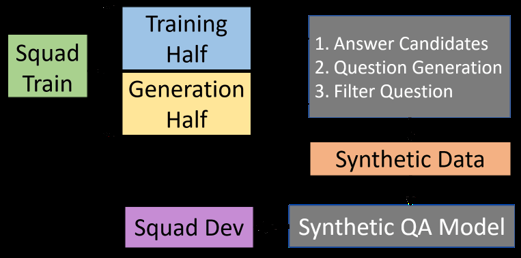