Training Question Answering Models From Synthetic Data
Raul Puri, Ryan Spring, Mohammad Shoeybi, Mostofa Patwary, Bryan Catanzaro
Question Answering Long Paper
You can open the pre-recorded video in a separate window.
Abstract:
Question and answer generation is a data augmentation method that aims to improve question answering (QA) models given the limited amount of human labeled data. However, a considerable gap remains between synthetic and human-generated question-answer pairs. This work aims to narrow this gap by taking advantage of large language models and explores several factors such as model size, quality of pretrained models, scale of data synthesized, and algorithmic choices. On the SQuAD1.1 question answering task, we achieve higher accuracy using solely synthetic questions and answers than when using the SQuAD1.1 training set questions alone. Removing access to real Wikipedia data, we synthesize questions and answers from a synthetic text corpus generated by an 8.3 billion parameter GPT-2 model and achieve 88.4 Exact Match (EM) and 93.9 F1 score on the SQuAD1.1 dev set. We further apply our methodology to SQuAD2.0 and show a 2.8 absolute gain on EM score compared to prior work using synthetic data.
NOTE: Video may display a random order of authors.
Correct author list is at the top of this page.
Connected Papers in EMNLP2020
Similar Papers
Unsupervised Question Decomposition for Question Answering
Ethan Perez, Patrick Lewis, Wen-tau Yih, Kyunghyun Cho, Douwe Kiela,

Inquisitive Question Generation for High Level Text Comprehension
Wei-Jen Ko, Te-yuan Chen, Yiyan Huang, Greg Durrett, Junyi Jessy Li,
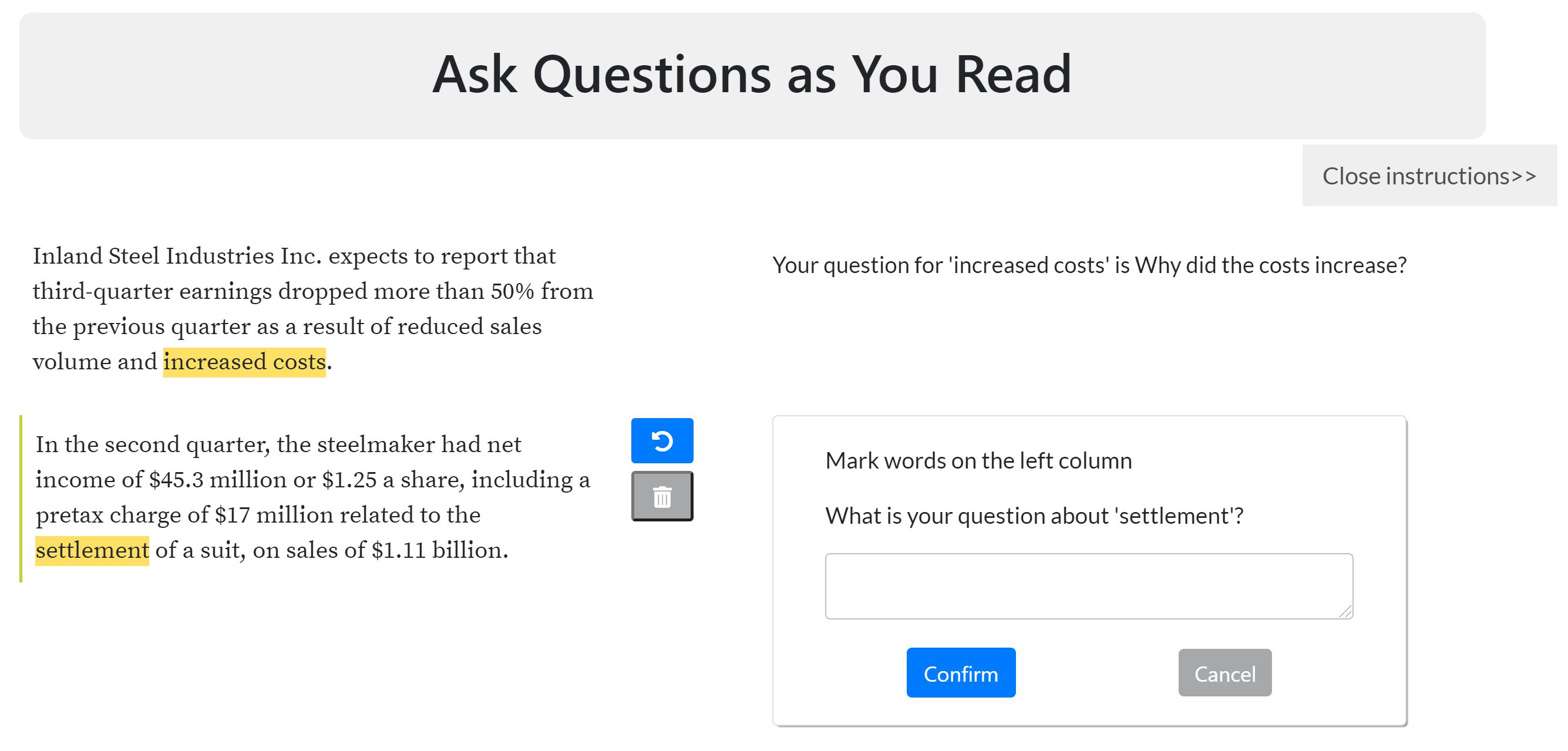
More Bang for Your Buck: Natural Perturbation for Robust Question Answering
Daniel Khashabi, Tushar Khot, Ashish Sabharwal,
