IIRC: A Dataset of Incomplete Information Reading Comprehension Questions
James Ferguson, Matt Gardner, Hannaneh Hajishirzi, Tushar Khot, Pradeep Dasigi
Question Answering Long Paper
You can open the pre-recorded video in a separate window.
Abstract:
Humans often have to read multiple documents to address their information needs. However, most existing reading comprehension (RC) tasks only focus on questions for which the contexts provide all the information required to answer them, thus not evaluating a system's performance at identifying a potential lack of sufficient information and locating sources for that information. To fill this gap, we present a dataset, IIRC, with more than 13K questions over paragraphs from English Wikipedia that provide only partial information to answer them, with the missing information occurring in one or more linked documents. The questions were written by crowd workers who did not have access to any of the linked documents, leading to questions that have little lexical overlap with the contexts where the answers appear. This process also gave many questions without answers, and those that require discrete reasoning, increasing the difficulty of the task. We follow recent modeling work on various reading comprehension datasets to construct a baseline model for this dataset, finding that it achieves 31.1% F1 on this task, while estimated human performance is 88.4%. The dataset, code for the baseline system, and a leaderboard can be found at https://allennlp.org/iirc.
NOTE: Video may display a random order of authors.
Correct author list is at the top of this page.
Connected Papers in EMNLP2020
Similar Papers
Inquisitive Question Generation for High Level Text Comprehension
Wei-Jen Ko, Te-yuan Chen, Yiyan Huang, Greg Durrett, Junyi Jessy Li,
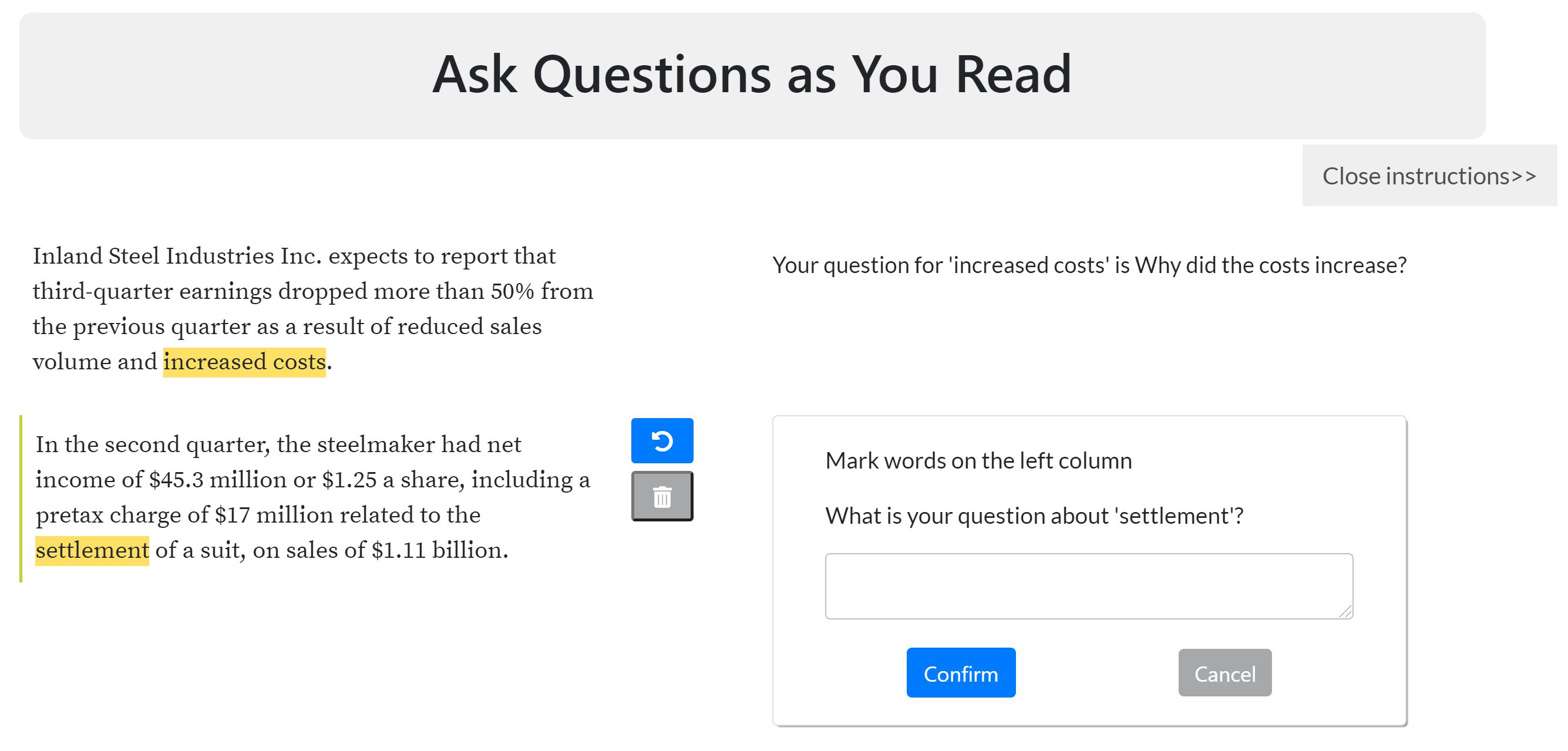
TORQUE: A Reading Comprehension Dataset of Temporal Ordering Questions
Qiang Ning, Hao Wu, Rujun Han, Nanyun Peng, Matt Gardner, Dan Roth,
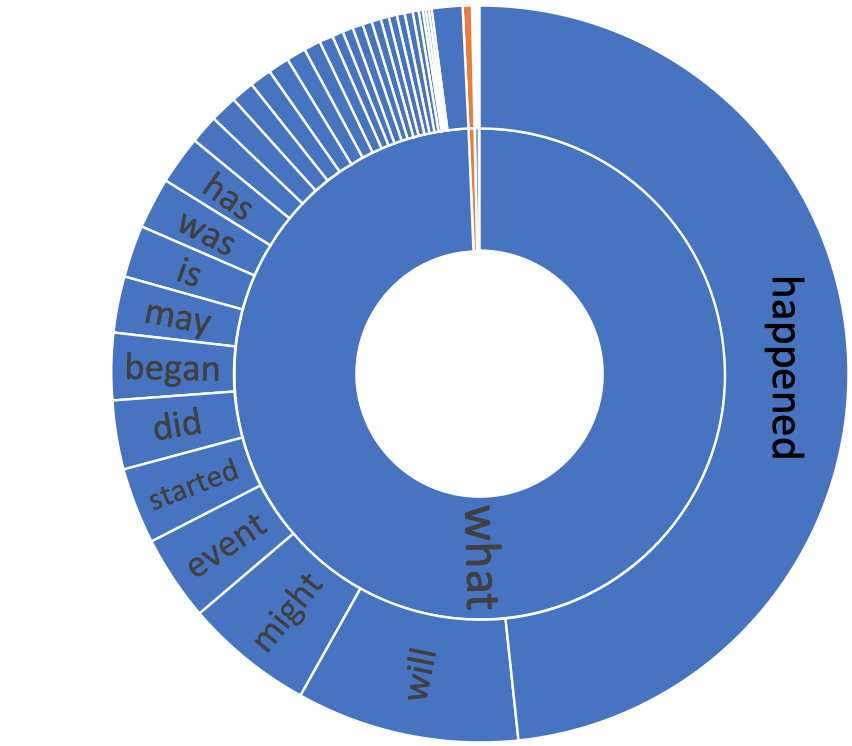
MOCHA: A Dataset for Training and Evaluating Generative Reading Comprehension Metrics
Anthony Chen, Gabriel Stanovsky, Sameer Singh, Matt Gardner,
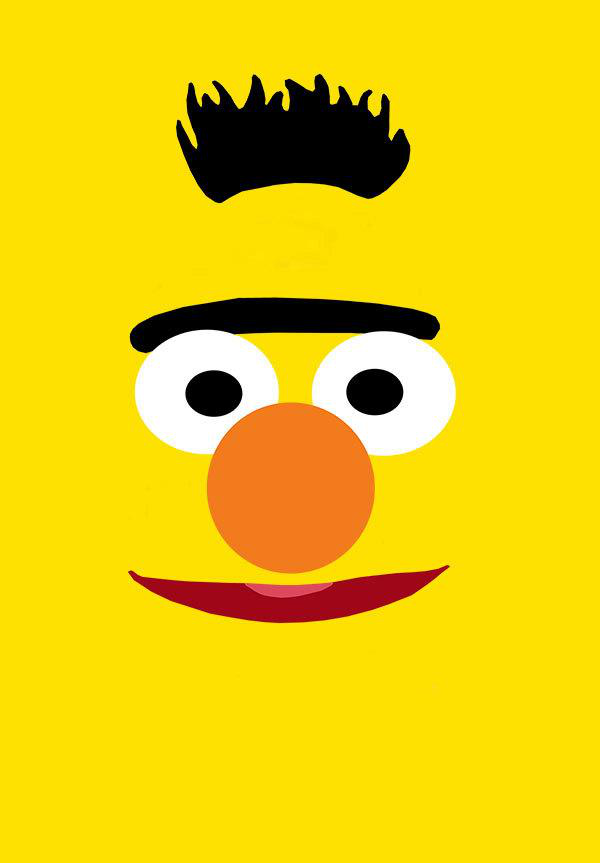
Towards Interpreting BERT for Reading Comprehension Based QA
Sahana Ramnath, Preksha Nema, Deep Sahni, Mitesh M. Khapra,
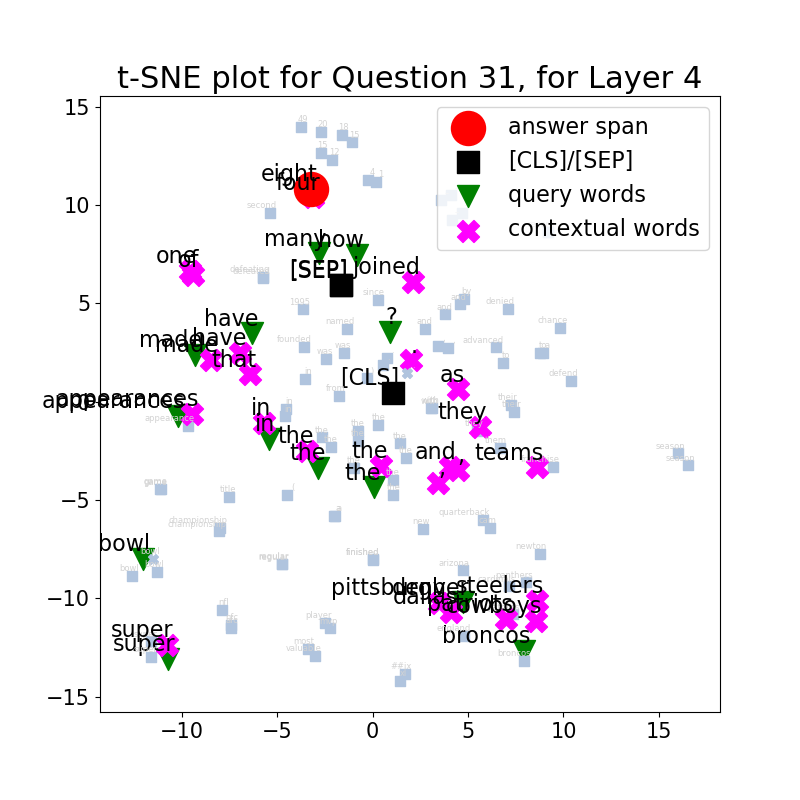