Context-Aware Answer Extraction in Question Answering
Yeon Seonwoo, Ji-Hoon Kim, Jung-Woo Ha, Alice Oh
Question Answering Long Paper
You can open the pre-recorded video in a separate window.
Abstract:
Extractive QA models have shown very promising performance in predicting the correct answer to a question for a given passage. However, they sometimes result in predicting the correct answer text but in a context irrelevant to the given question. This discrepancy becomes especially important as the number of occurrences of the answer text in a passage increases. To resolve this issue, we propose BLANC (BLock AttentioN for Context prediction) based on two main ideas: context prediction as an auxiliary task in multi-task learning manner, and a block attention method that learns the context prediction task. With experiments on reading comprehension, we show that BLANC outperforms the state-of-the-art QA models, and the performance gap increases as the number of answer text occurrences increases. We also conduct an experiment of training the models using SQuAD and predicting the supporting facts on HotpotQA and show that BLANC outperforms all baseline models in this zero-shot setting.
NOTE: Video may display a random order of authors.
Correct author list is at the top of this page.
Connected Papers in EMNLP2020
Similar Papers
Training Question Answering Models From Synthetic Data
Raul Puri, Ryan Spring, Mohammad Shoeybi, Mostofa Patwary, Bryan Catanzaro,
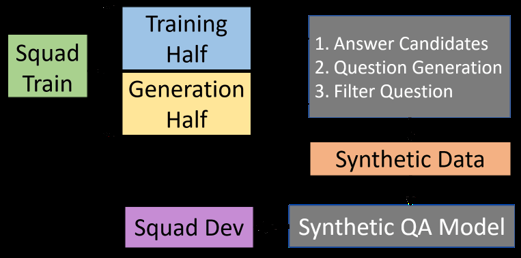
Look at the First Sentence: Position Bias in Question Answering
Miyoung Ko, Jinhyuk Lee, Hyunjae Kim, Gangwoo Kim, Jaewoo Kang,
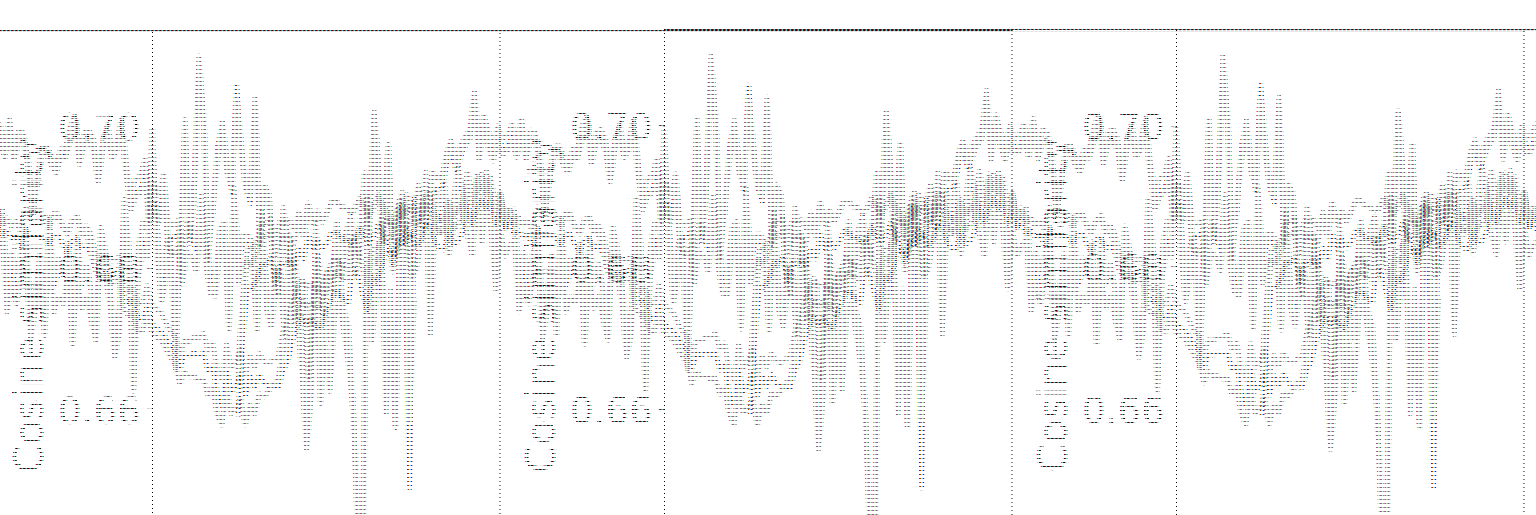
Inquisitive Question Generation for High Level Text Comprehension
Wei-Jen Ko, Te-yuan Chen, Yiyan Huang, Greg Durrett, Junyi Jessy Li,
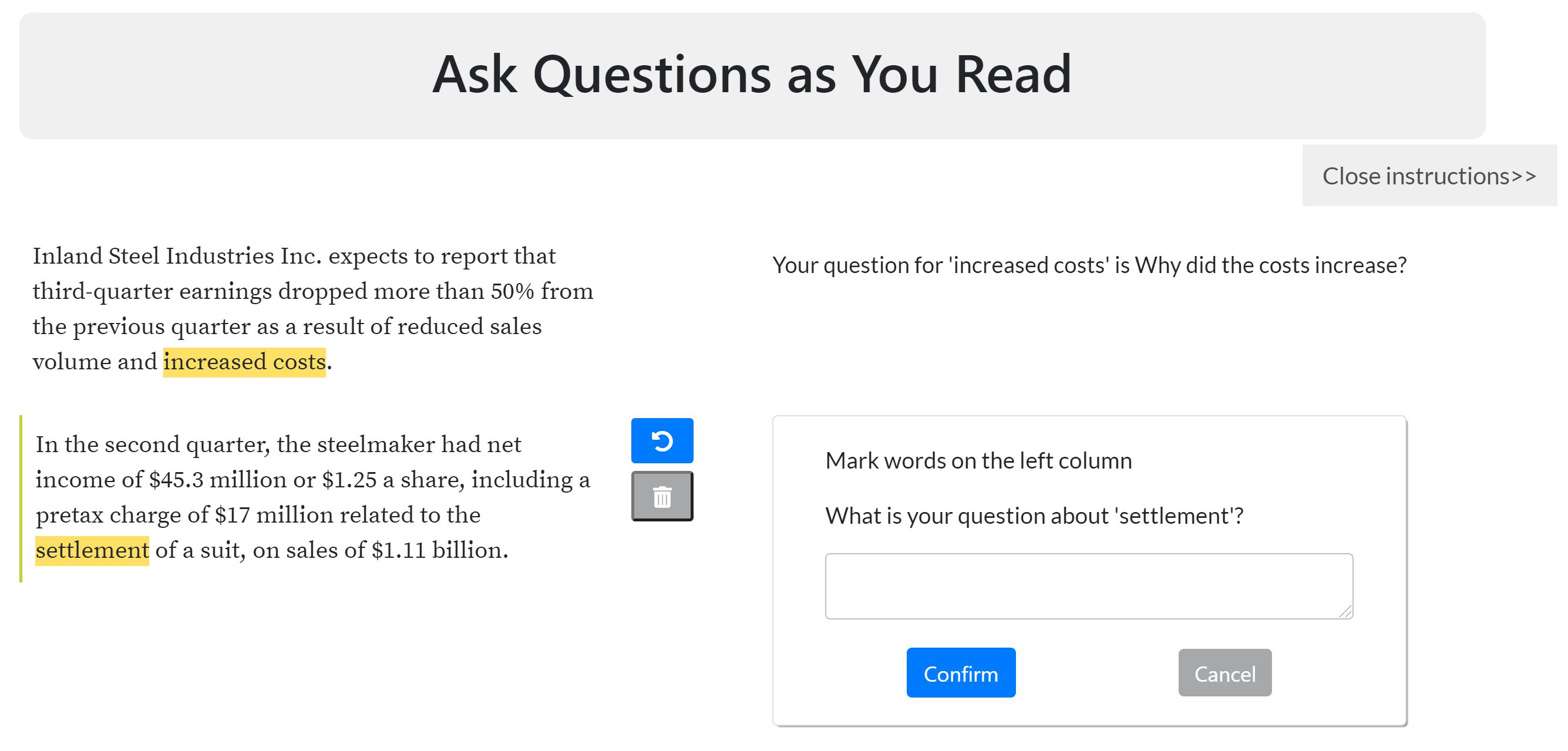
Don't Read Too Much Into It: Adaptive Computation for Open-Domain Question Answering
Yuxiang Wu, Sebastian Riedel, Pasquale Minervini, Pontus Stenetorp,
