PathQG: Neural Question Generation from Facts
Siyuan Wang, Zhongyu Wei, Zhihao Fan, Zengfeng Huang, Weijian Sun, Qi Zhang, Xuanjing Huang
NLP Applications Long Paper
You can open the pre-recorded video in a separate window.
Abstract:
Existing research for question generation encodes the input text as a sequence of tokens without explicitly modeling fact information. These models tend to generate irrelevant and uninformative questions. In this paper, we explore to incorporate facts in the text for question generation in a comprehensive way. We present a novel task of question generation given a query path in the knowledge graph constructed from the input text. We divide the task into two steps, namely, query representation learning and query-based question generation. We formulate query representation learning as a sequence labeling problem for identifying the involved facts to form a query and employ an RNN-based generator for question generation. We first train the two modules jointly in an end-to-end fashion, and further enforce the interaction between these two modules in a variational framework. We construct the experimental datasets on top of SQuAD and results show that our model outperforms other state-of-the-art approaches, and the performance margin is larger when target questions are complex. Human evaluation also proves that our model is able to generate relevant and informative questions.\footnote{Our code is available at \url{https://github.com/WangsyGit/PathQG}.}
NOTE: Video may display a random order of authors.
Correct author list is at the top of this page.
Connected Papers in EMNLP2020
Similar Papers
Understanding Neural Abstractive Summarization Models via Uncertainty
Jiacheng Xu, Shrey Desai, Greg Durrett,
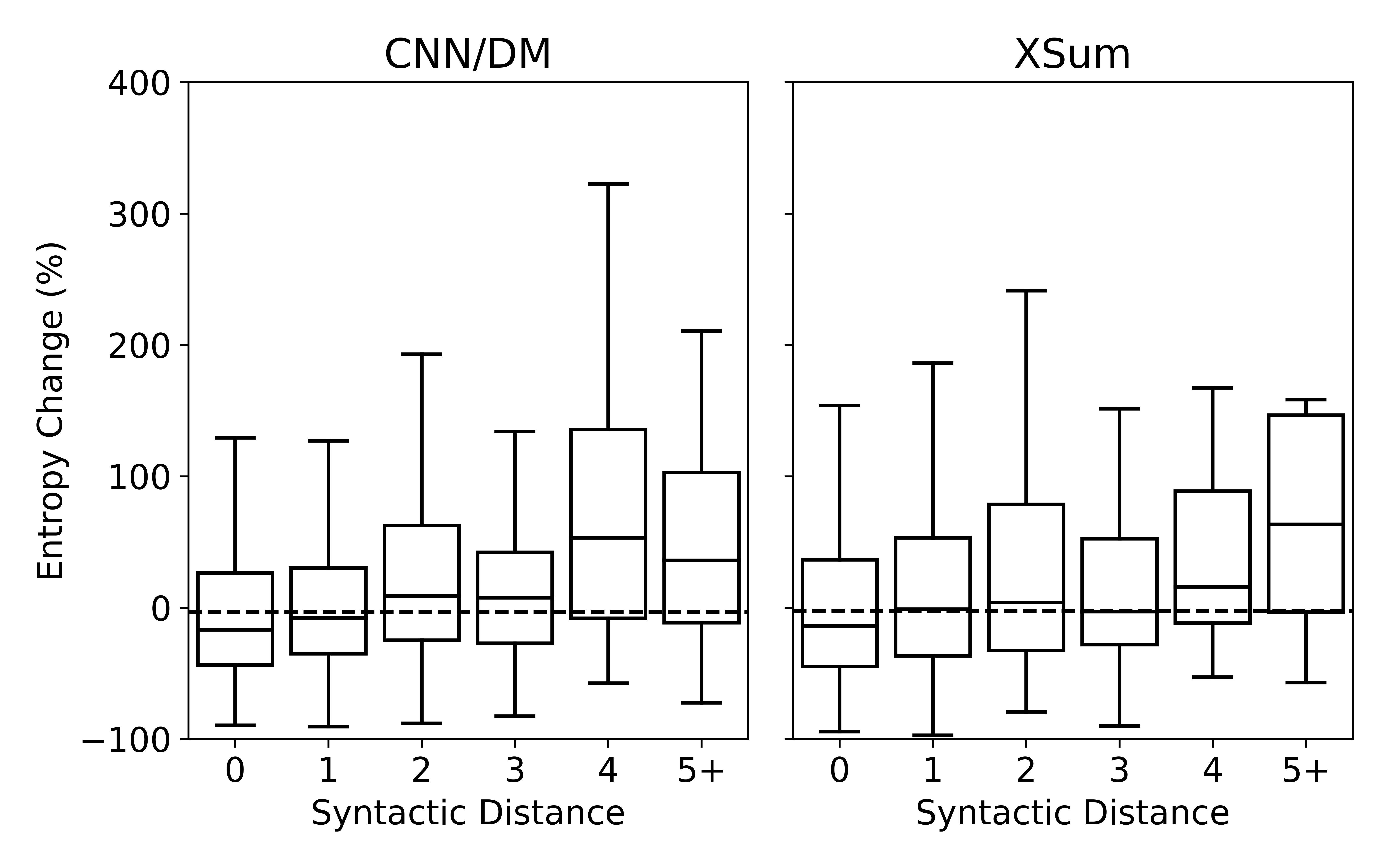
Training Question Answering Models From Synthetic Data
Raul Puri, Ryan Spring, Mohammad Shoeybi, Mostofa Patwary, Bryan Catanzaro,
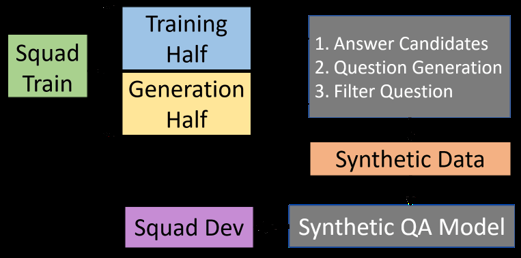
Context-Aware Answer Extraction in Question Answering
Yeon Seonwoo, Ji-Hoon Kim, Jung-Woo Ha, Alice Oh,
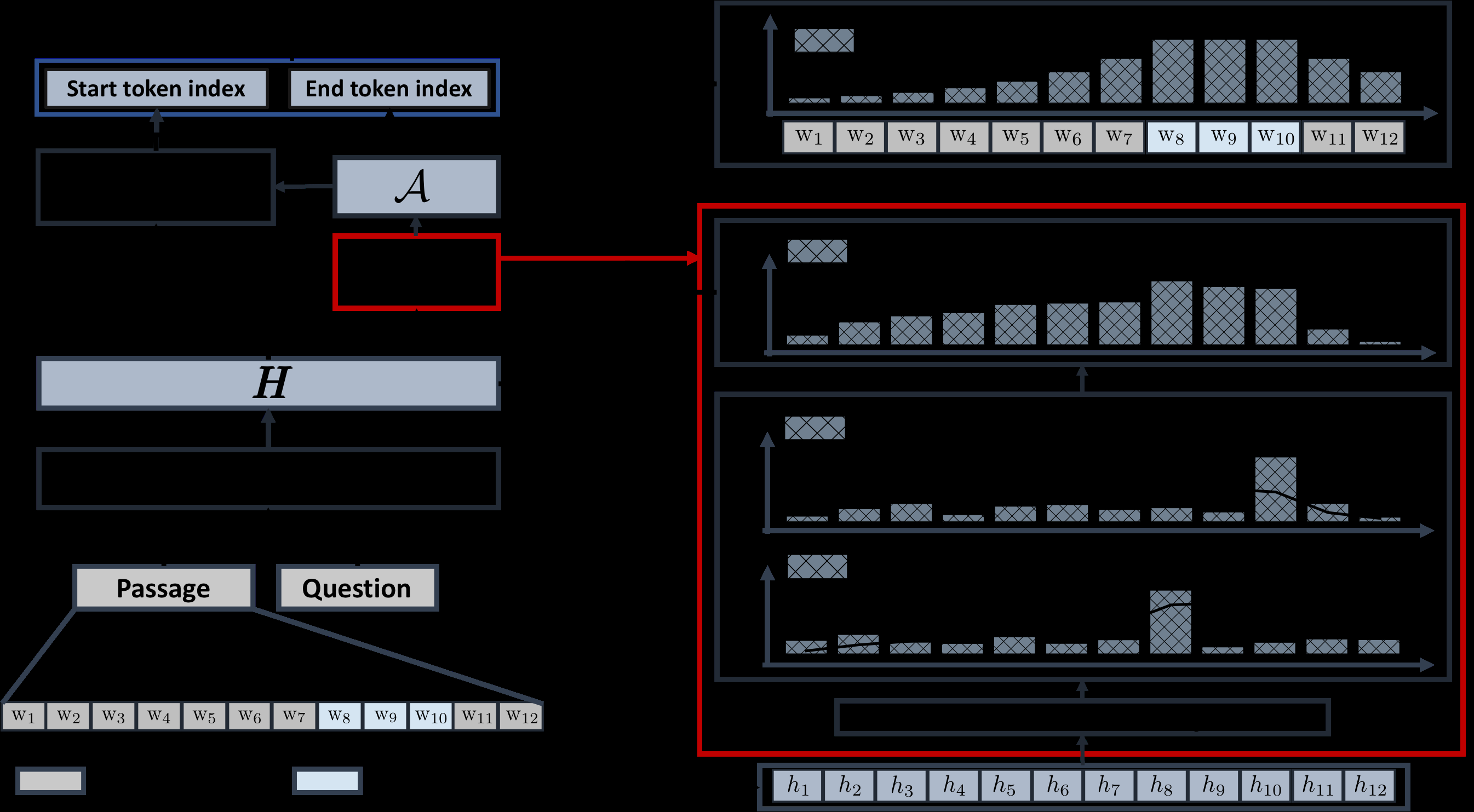