Data and Representation for Turkish Natural Language Inference
Emrah Budur, Rıza Özçelik, Tunga Gungor, Christopher Potts
Semantics: Sentence-level Semantics, Textual Inference and Other areas Long Paper
You can open the pre-recorded video in a separate window.
Abstract:
Large annotated datasets in NLP are overwhelmingly in English. This is an obstacle to progress in other languages. Unfortunately, obtaining new annotated resources for each task in each language would be prohibitively expensive. At the same time, commercial machine translation systems are now robust. Can we leverage these systems to translate English-language datasets automatically? In this paper, we offer a positive response for natural language inference (NLI) in Turkish. We translated two large English NLI datasets into Turkish and had a team of experts validate their translation quality and fidelity to the original labels. Using these datasets, we address core issues of representation for Turkish NLI. We find that in-language embeddings are essential and that morphological parsing can be avoided where the training set is large. Finally, we show that models trained on our machine-translated datasets are successful on human-translated evaluation sets. We share all code, models, and data publicly.
NOTE: Video may display a random order of authors.
Correct author list is at the top of this page.
Connected Papers in EMNLP2020
Similar Papers
Localizing Open-Ontology QA Semantic Parsers in a Day Using Machine Translation
Mehrad Moradshahi, Giovanni Campagna, Sina Semnani, Silei Xu, Monica Lam,

Language Model Prior for Low-Resource Neural Machine Translation
Christos Baziotis, Barry Haddow, Alexandra Birch,
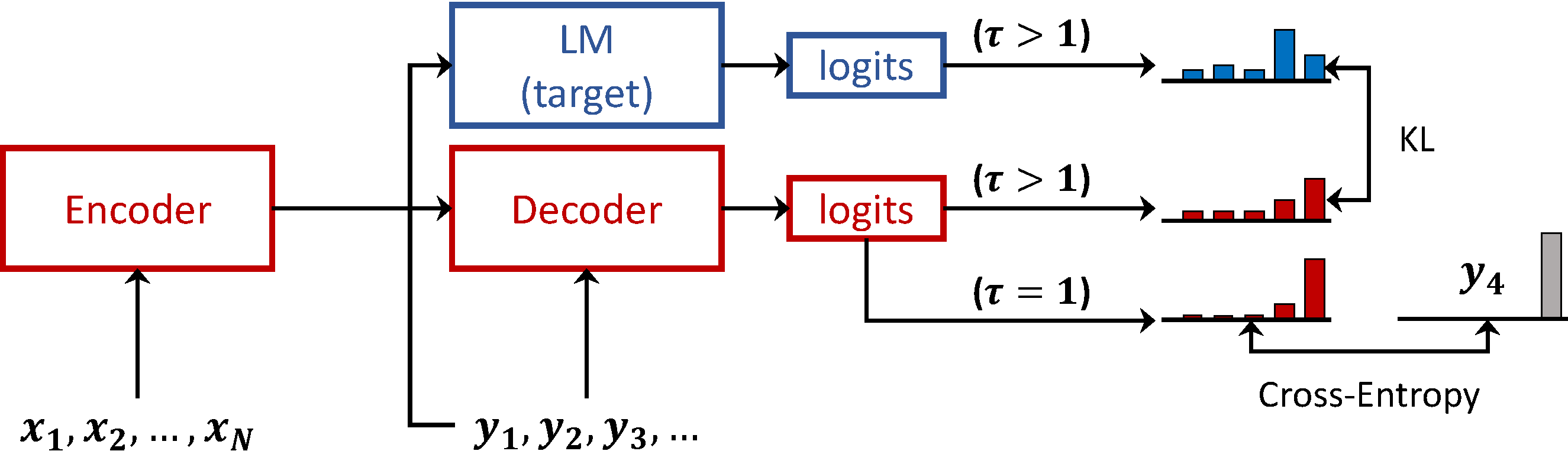