Localizing Open-Ontology QA Semantic Parsers in a Day Using Machine Translation
Mehrad Moradshahi, Giovanni Campagna, Sina Semnani, Silei Xu, Monica Lam
Machine Translation and Multilinguality Long Paper
You can open the pre-recorded video in a separate window.
Abstract:
We propose Semantic Parser Localizer (SPL), a toolkit that leverages Neural Machine Translation (NMT) systems to localize a semantic parser for a new language. Our methodology is to (1) generate training data automatically in the target language by augmenting machine-translated datasets with local entities scraped from public websites, (2) add a few-shot boost of human-translated sentences and train a novel XLMR-LSTM semantic parser, and (3) test the model on natural utterances curated using human translators. We assess the effectiveness of our approach by extending the current capabilities of Schema2QA, a system for English Question Answering (QA) on the open web, to 10 new languages for the restaurants and hotels domains. Our model achieves an overall test accuracy ranging between 61% and 69% for the hotels domain and between 64% and 78% for restaurants domain, which compares favorably to 69% and 80% obtained for English parser trained on gold English data and a few examples from validation set. We show our approach outperforms the previous state-of-the-art methodology by more than 30% for hotels and 40% for restaurants with localized ontologies for the subset of languages tested. Our methodology enables any software developer to add a new language capability to a QA system for a new domain, leveraging machine translation, in less than 24 hours. Our code is released open-source.
NOTE: Video may display a random order of authors.
Correct author list is at the top of this page.
Connected Papers in EMNLP2020
Similar Papers
AutoQA: From Databases To QA Semantic Parsers With Only Synthetic Training Data
Silei Xu, Sina Semnani, Giovanni Campagna, Monica Lam,

XL-WiC: A Multilingual Benchmark for Evaluating Semantic Contextualization
Alessandro Raganato, Tommaso Pasini, Jose Camacho-Collados, Mohammad Taher Pilehvar,
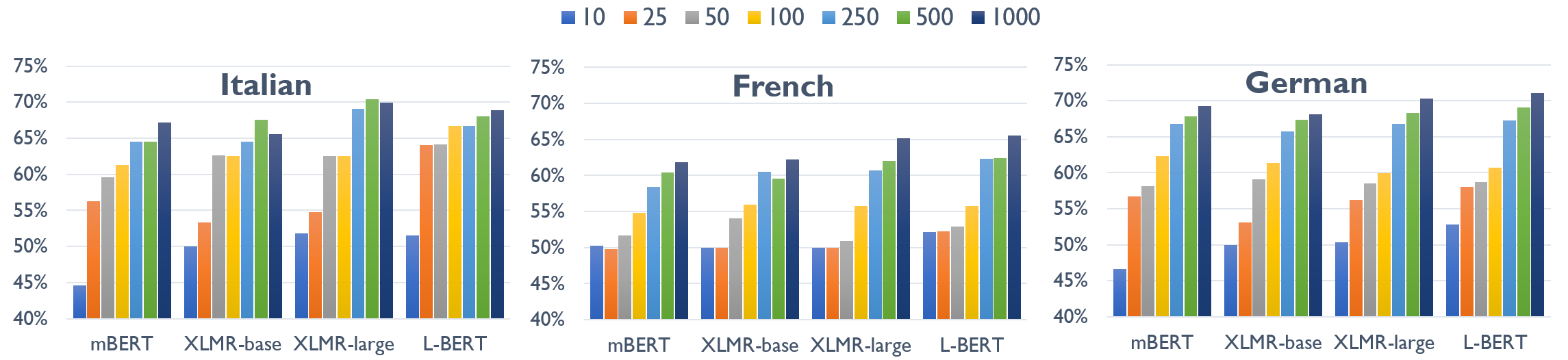
Reusing a Pretrained Language Model on Languages with Limited Corpora for Unsupervised NMT
Alexandra Chronopoulou, Dario Stojanovski, Alexander Fraser,
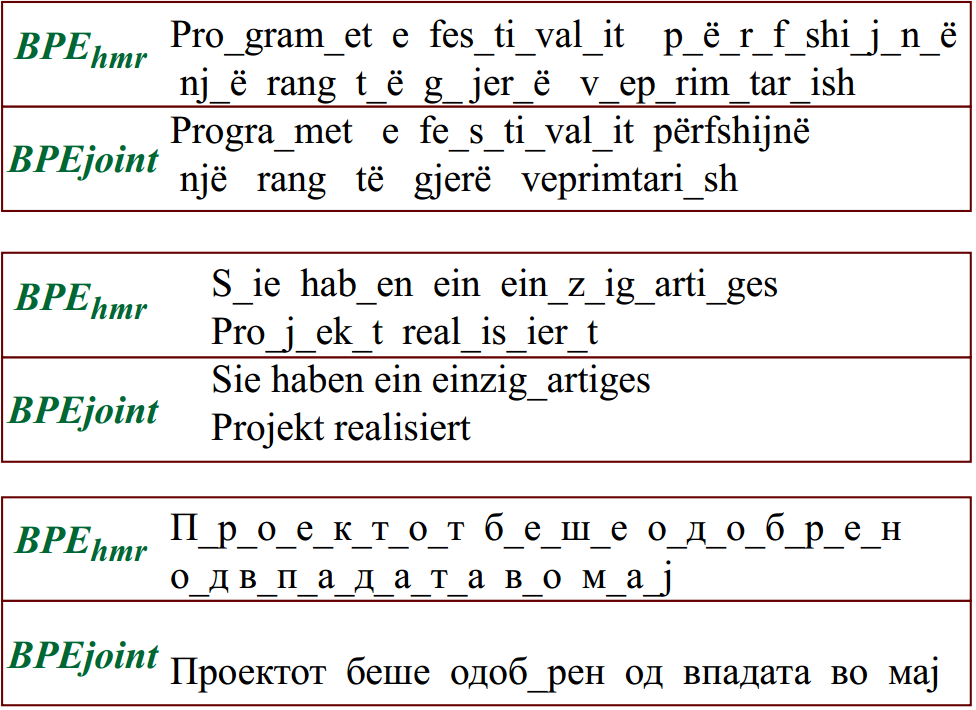
Data and Representation for Turkish Natural Language Inference
Emrah Budur, Rıza Özçelik, Tunga Gungor, Christopher Potts,
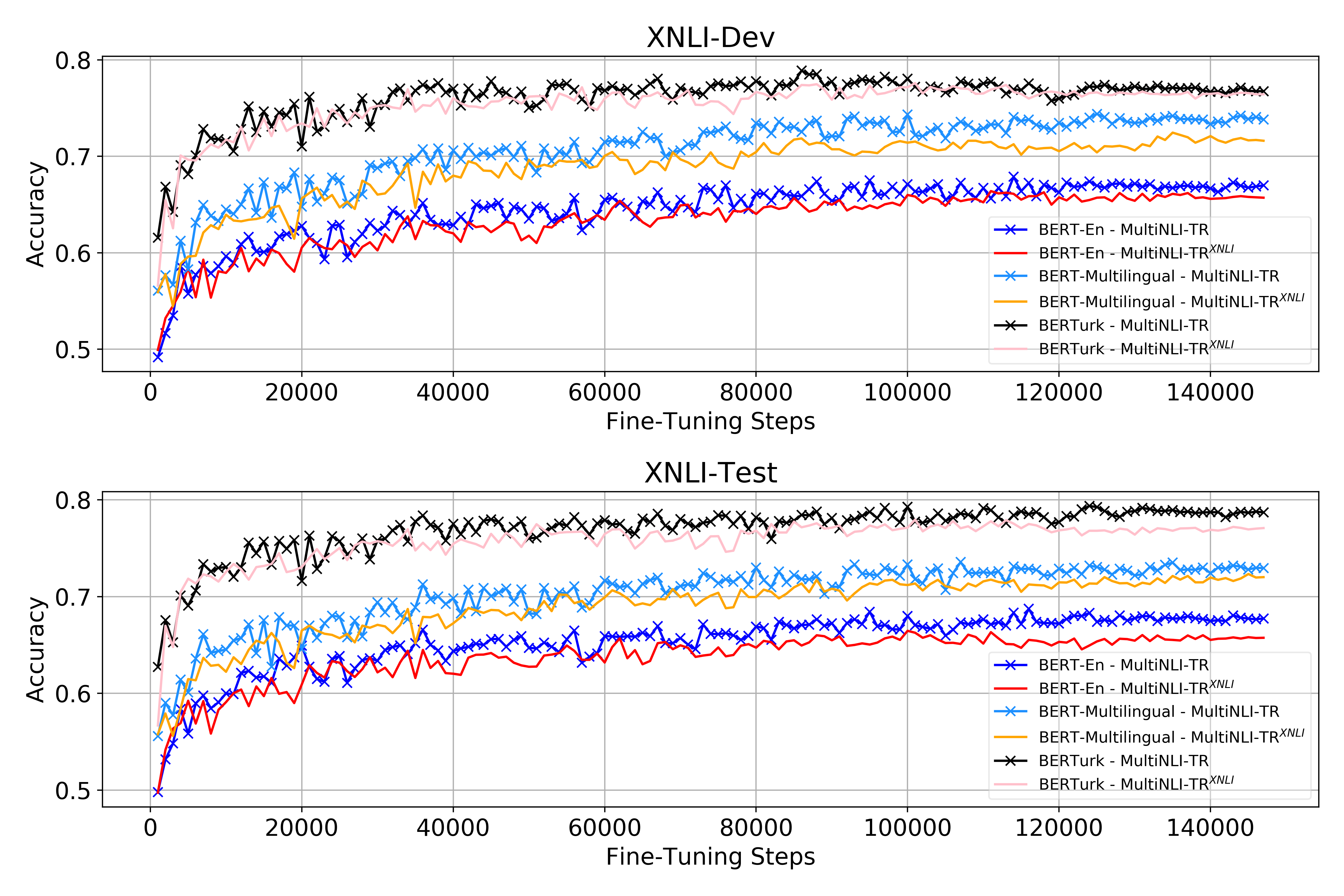