Alignment-free Cross-lingual Semantic Role Labeling
Rui Cai, Mirella Lapata
Semantics: Sentence-level Semantics, Textual Inference and Other areas Long Paper
You can open the pre-recorded video in a separate window.
Abstract:
Cross-lingual semantic role labeling (SRL) aims at leveraging resources in a source language to minimize the effort required to construct annotations or models for a new target language. Recent approaches rely on word alignments, machine translation engines, or preprocessing tools such as parsers or taggers. We propose a cross-lingual SRL model which only requires annotations in a source language and access to raw text in the form of a parallel corpus. The backbone of our model is an LSTM-based semantic role labeler jointly trained with a semantic role compressor and multilingual word embeddings. The compressor collects useful information from the output of the semantic role labeler, filtering noisy and conflicting evidence. It lives in a multilingual embedding space and provides direct supervision for predicting semantic roles in the target language. Results on the Universal Proposition Bank and manually annotated datasets show that our method is highly effective, even against systems utilizing supervised features.
NOTE: Video may display a random order of authors.
Correct author list is at the top of this page.
Connected Papers in EMNLP2020
Similar Papers
Localizing Open-Ontology QA Semantic Parsers in a Day Using Machine Translation
Mehrad Moradshahi, Giovanni Campagna, Sina Semnani, Silei Xu, Monica Lam,

XL-WiC: A Multilingual Benchmark for Evaluating Semantic Contextualization
Alessandro Raganato, Tommaso Pasini, Jose Camacho-Collados, Mohammad Taher Pilehvar,
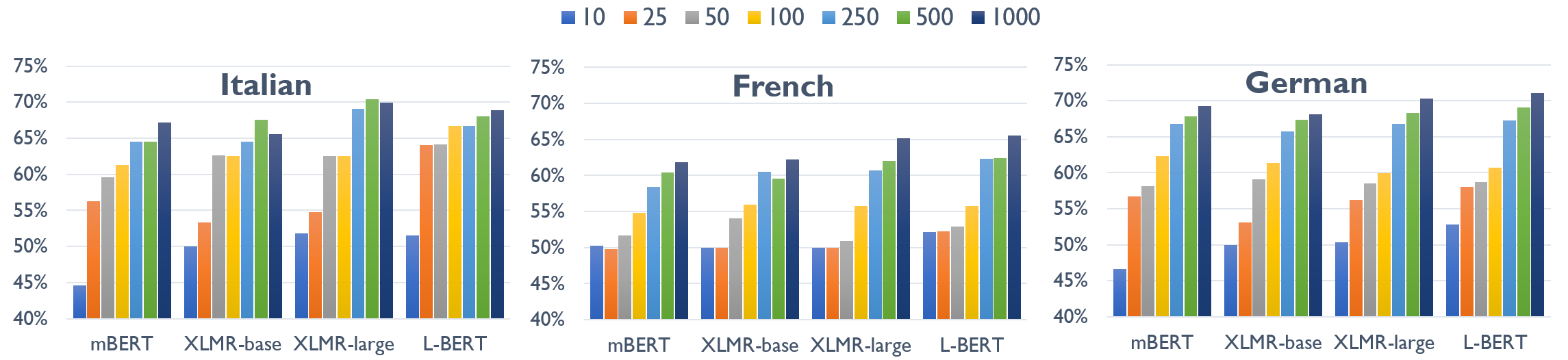
End-to-End Slot Alignment and Recognition for Cross-Lingual NLU
Weijia Xu, Batool Haider, Saab Mansour,

Unsupervised Cross-Lingual Part-of-Speech Tagging for Truly Low-Resource Scenarios
Ramy Eskander, Smaranda Muresan, Michael Collins,
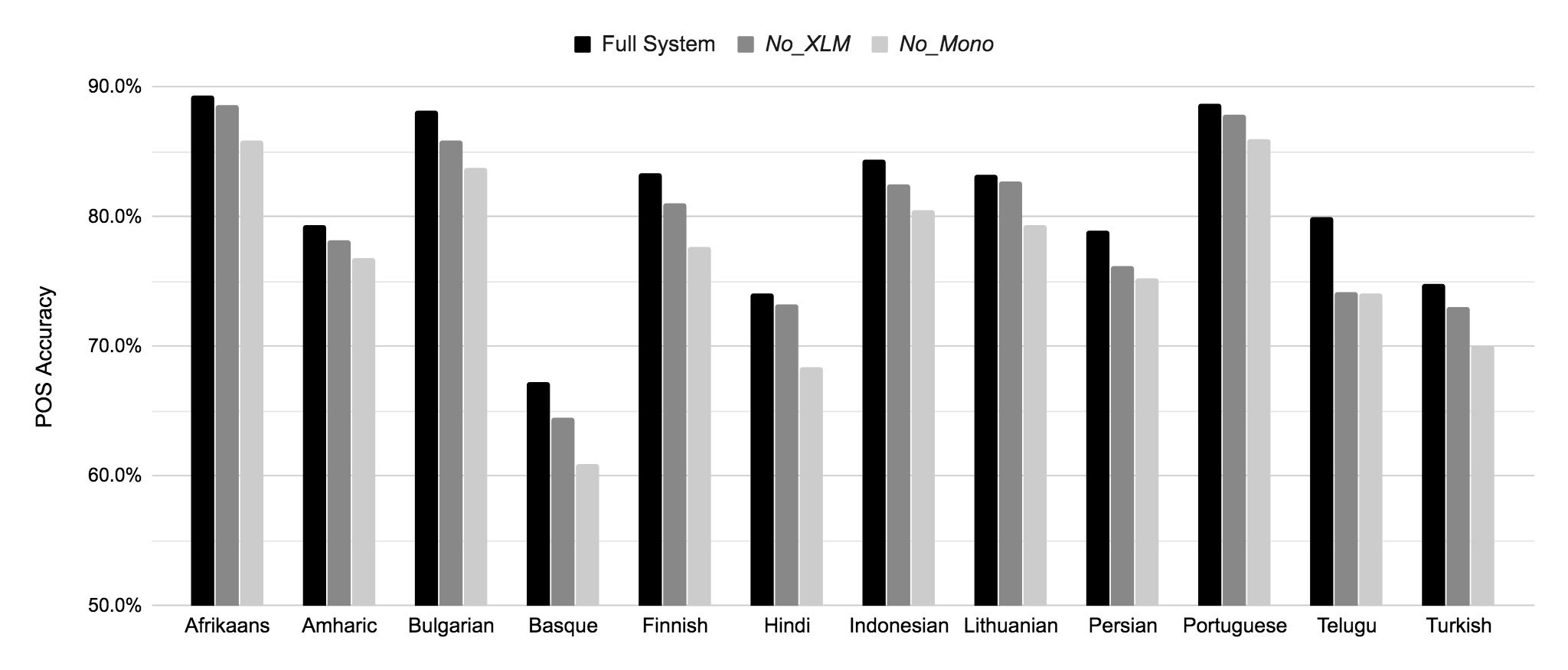