Language Model Prior for Low-Resource Neural Machine Translation
Christos Baziotis, Barry Haddow, Alexandra Birch
Machine Translation and Multilinguality Long Paper
You can open the pre-recorded video in a separate window.
Abstract:
The scarcity of large parallel corpora is an important obstacle for neural machine translation. A common solution is to exploit the knowledge of language models (LM) trained on abundant monolingual data. In this work, we propose a novel approach to incorporate a LM as prior in a neural translation model (TM). Specifically, we add a regularization term, which pushes the output distributions of the TM to be probable under the LM prior, while avoiding wrong predictions when the TM "disagrees" with the LM. This objective relates to knowledge distillation, where the LM can be viewed as teaching the TM about the target language. The proposed approach does not compromise decoding speed, because the LM is used only at training time, unlike previous work that requires it during inference. We present an analysis of the effects that different methods have on the distributions of the TM. Results on two low-resource machine translation datasets show clear improvements even with limited monolingual data.
NOTE: Video may display a random order of authors.
Correct author list is at the top of this page.
Connected Papers in EMNLP2020
Similar Papers
Pre-training Multilingual Neural Machine Translation by Leveraging Alignment Information
Zehui Lin, Xiao Pan, Mingxuan Wang, Xipeng Qiu, Jiangtao Feng, Hao Zhou, Lei Li,

Multi-task Learning for Multilingual Neural Machine Translation
Yiren Wang, ChengXiang Zhai, Hany Hassan,
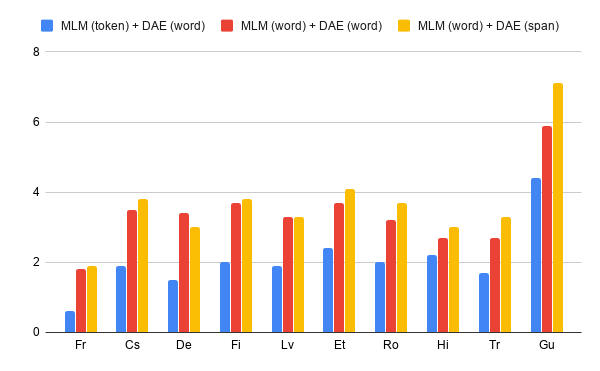
Zero-Shot Cross-Lingual Transfer with Meta Learning
Farhad Nooralahzadeh, Giannis Bekoulis, Johannes Bjerva, Isabelle Augenstein,
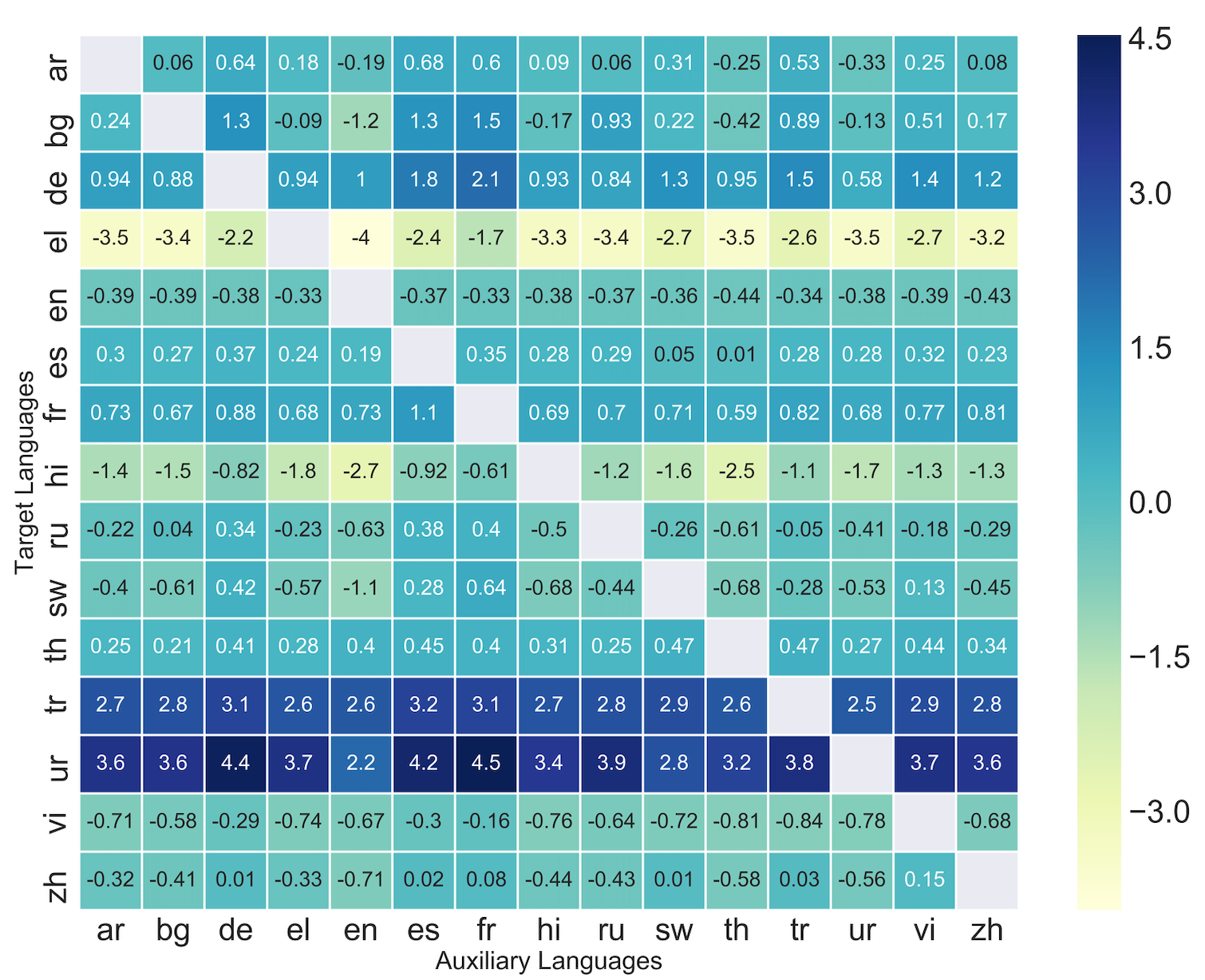
On the Sparsity of Neural Machine Translation Models
Yong Wang, Longyue Wang, Victor Li, Zhaopeng Tu,
