Program Enhanced Fact Verification with Verbalization and Graph Attention Network
Xiaoyu Yang, Feng Nie, Yufei Feng, Quan Liu, Zhigang Chen, Xiaodan Zhu
Semantics: Sentence-level Semantics, Textual Inference and Other areas Long Paper
You can open the pre-recorded video in a separate window.
Abstract:
Performing fact verification based on structured data is important for many real-life applications and is a challenging research problem, particularly when it involves both symbolic operations and informal inference based on language understanding. In this paper, we present a Program-enhanced Verbalization and Graph Attention Network (ProgVGAT) to integrate programs and execution into textual inference models. Specifically, a verbalization with program execution model is proposed to accumulate evidences that are embedded in operations over the tables. Built on that, we construct the graph attention verification networks, which are designed to fuse different sources of evidences from verbalized program execution, program structures, and the original statements and tables, to make the final verification decision. To support the above framework, we propose a program selection module optimized with a new training strategy based on margin loss, to produce more accurate programs, which is shown to be effective in enhancing the final verification results. Experimental results show that the proposed framework achieves the new state-of-the-art performance, a 74.4% accuracy, on the benchmark dataset TABFACT.
NOTE: Video may display a random order of authors.
Correct author list is at the top of this page.
Connected Papers in EMNLP2020
Similar Papers
Table Fact Verification with Structure-Aware Transformer
Hongzhi Zhang, Yingyao Wang, Sirui Wang, Xuezhi Cao, Fuzheng Zhang, Zhongyuan Wang,
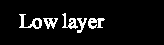
A Knowledge-Aware Sequence-to-Tree Network for Math Word Problem Solving
Qinzhuo Wu, Qi Zhang, Jinlan Fu, Xuanjing Huang,

Evaluating the Factual Consistency of Abstractive Text Summarization
Wojciech Kryscinski, Bryan McCann, Caiming Xiong, Richard Socher,
