SRLGRN: Semantic Role Labeling Graph Reasoning Network
Chen Zheng, Parisa Kordjamshidi
Question Answering Long Paper
You can open the pre-recorded video in a separate window.
Abstract:
This work deals with the challenge of learning and reasoning over multi-hop question answering (QA). We propose a graph reasoning network based on the semantic structure of the sentences to learn cross paragraph reasoning paths and find the supporting facts and the answer jointly. The proposed graph is a heterogeneous document-level graph that contains nodes of type sentence (question, title, and other sentences), and semantic role labeling sub-graphs per sentence that contain arguments as nodes and predicates as edges. Incorporating the argument types, the argument phrases, and the semantics of the edges originated from SRL predicates into the graph encoder helps in finding and also the explainability of the reasoning paths. Our proposed approach shows competitive performance on the HotpotQA distractor setting benchmark compared to the recent state-of-the-art models.
NOTE: Video may display a random order of authors.
Correct author list is at the top of this page.
Connected Papers in EMNLP2020
Similar Papers
Hierarchical Graph Network for Multi-hop Question Answering
Yuwei Fang, Siqi Sun, Zhe Gan, Rohit Pillai, Shuohang Wang, Jingjing Liu,
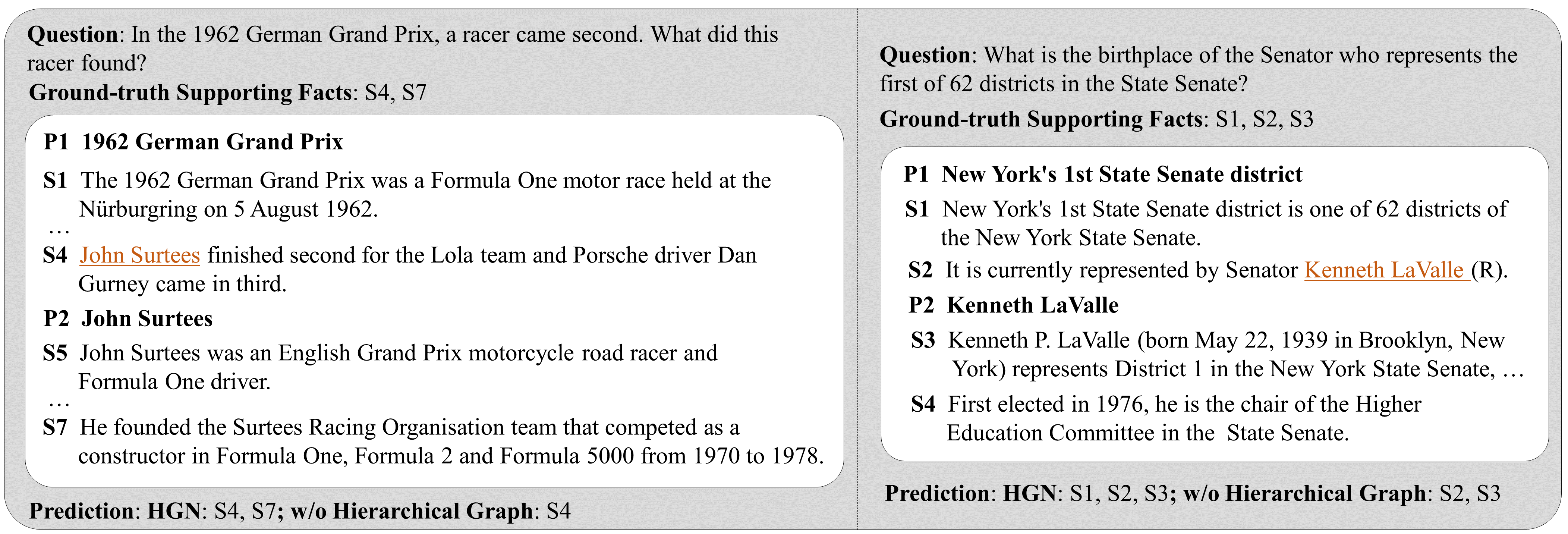
Is Graph Structure Necessary for Multi-hop Question Answering?
Nan Shao, Yiming Cui, Ting Liu, Shijin Wang, Guoping Hu,
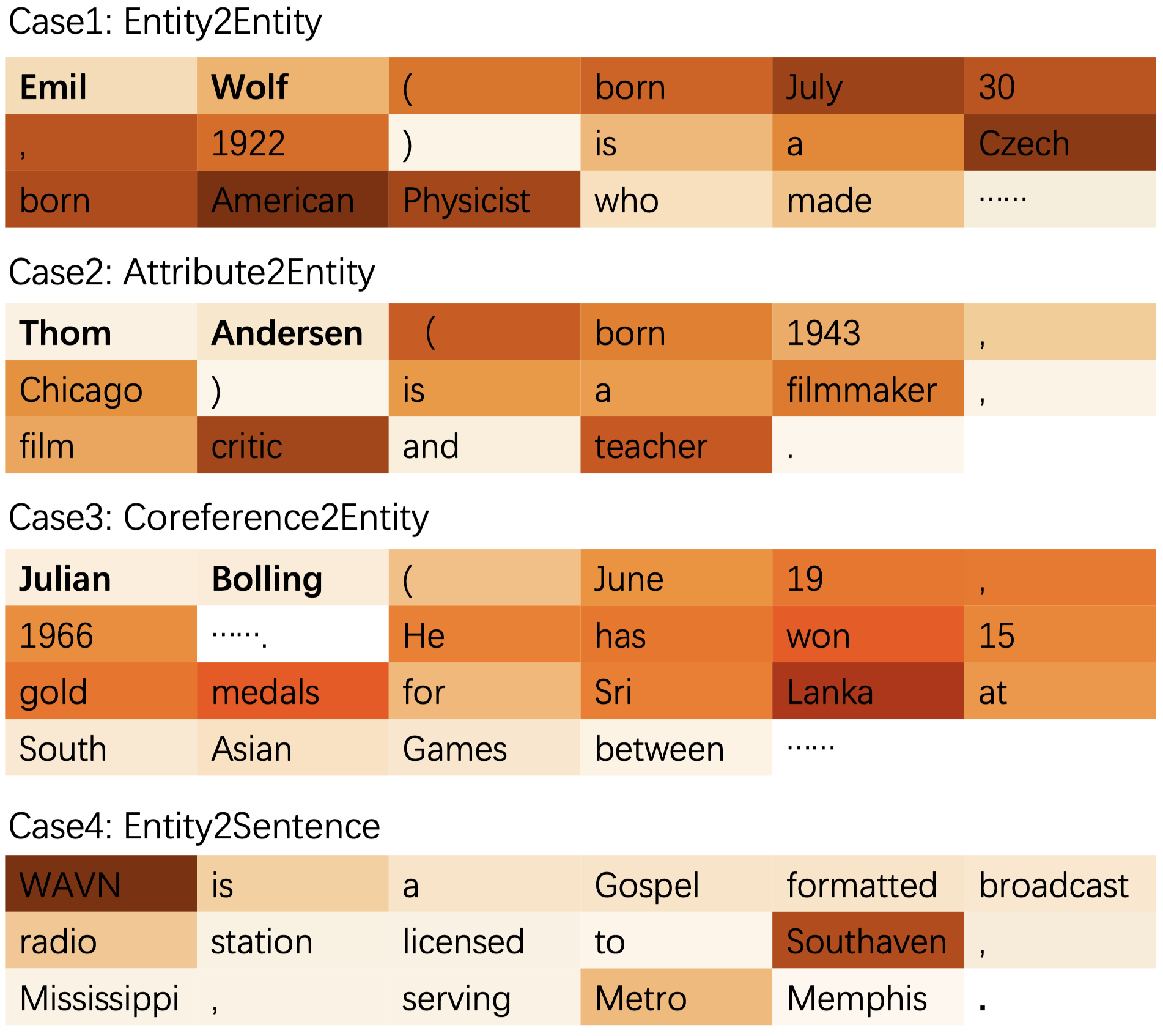
Double Graph Based Reasoning for Document-level Relation Extraction
Shuang Zeng, Runxin Xu, Baobao Chang, Lei Li,

Connecting the Dots: Event Graph Schema Induction with Path Language Modeling
Manling Li, Qi Zeng, Ying Lin, Kyunghyun Cho, Heng Ji, Jonathan May, Nathanael Chambers, Clare Voss,
