A Knowledge-Aware Sequence-to-Tree Network for Math Word Problem Solving
Qinzhuo Wu, Qi Zhang, Jinlan Fu, Xuanjing Huang
NLP Applications Long Paper
You can open the pre-recorded video in a separate window.
Abstract:
With the advancements in natural language processing tasks, math word problem solving has received increasing attention. Previous methods have achieved promising results but ignore background common-sense knowledge not directly provided by the problem. In addition, during generation, they focus on local features while neglecting global information. To incorporate external knowledge and global expression information, we propose a novel knowledge-aware sequence-to-tree (KA-S2T) network in which the entities in the problem sequences and their categories are modeled as an entity graph. Based on this entity graph, a graph attention network is used to capture knowledge-aware problem representations. Further, we use a tree-structured decoder with a state aggregation mechanism to capture the long-distance dependency and global expression information. Experimental results on the Math23K dataset revealed that the KA-S2T model can achieve better performance than previously reported best results.
NOTE: Video may display a random order of authors.
Correct author list is at the top of this page.
Connected Papers in EMNLP2020
Similar Papers
Exploiting Structured Knowledge in Text via Graph-Guided Representation Learning
Tao Shen, Yi Mao, Pengcheng He, Guodong Long, Adam Trischler, Weizhu Chen,

Modeling Global and Local Node Contexts for Text Generation from Knowledge Graphs
Leonardo F. R. Ribeiro, Yue Zhang, Claire Gardent, Iryna Gurevych,

Semantically-Aligned Universal Tree-Structured Solver for Math Word Problems
Jinghui Qin, Lihui Lin, Xiaodan Liang, Rumin Zhang, Liang Lin,

Scalable Multi-Hop Relational Reasoning for Knowledge-Aware Question Answering
Yanlin Feng, Xinyue Chen, Bill Yuchen Lin, Peifeng Wang, Jun Yan, Xiang Ren,
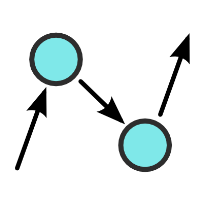