DualTKB: A Dual Learning Bridge between Text and Knowledge Base
Pierre Dognin, Igor Melnyk, Inkit Padhi, Cicero Nogueira dos Santos, Payel Das
Information Extraction Long Paper
You can open the pre-recorded video in a separate window.
Abstract:
In this work, we present a dual learning approach for unsupervised text to path and path to text transfers in Commonsense Knowledge Bases (KBs). We investigate the impact of weak supervision by creating a weakly supervised dataset and show that even a slight amount of supervision can significantly improve the model performance and enable better-quality transfers. We examine different model architectures, and evaluation metrics, proposing a novel Commonsense KB completion metric tailored for generative models. Extensive experimental results show that the proposed method compares very favorably to the existing baselines. This approach is a viable step towards a more advanced system for automatic KB construction/expansion and the reverse operation of KB conversion to coherent textual descriptions.
NOTE: Video may display a random order of authors.
Correct author list is at the top of this page.
Connected Papers in EMNLP2020
Similar Papers
Q-learning with Language Model for Edit-based Unsupervised Summarization
Ryosuke Kohita, Akifumi Wachi, Yang Zhao, Ryuki Tachibana,

Cross-Thought for Sentence Encoder Pre-training
Shuohang Wang, Yuwei Fang, Siqi Sun, Zhe Gan, Yu Cheng, Jingjing Liu, Jing Jiang,
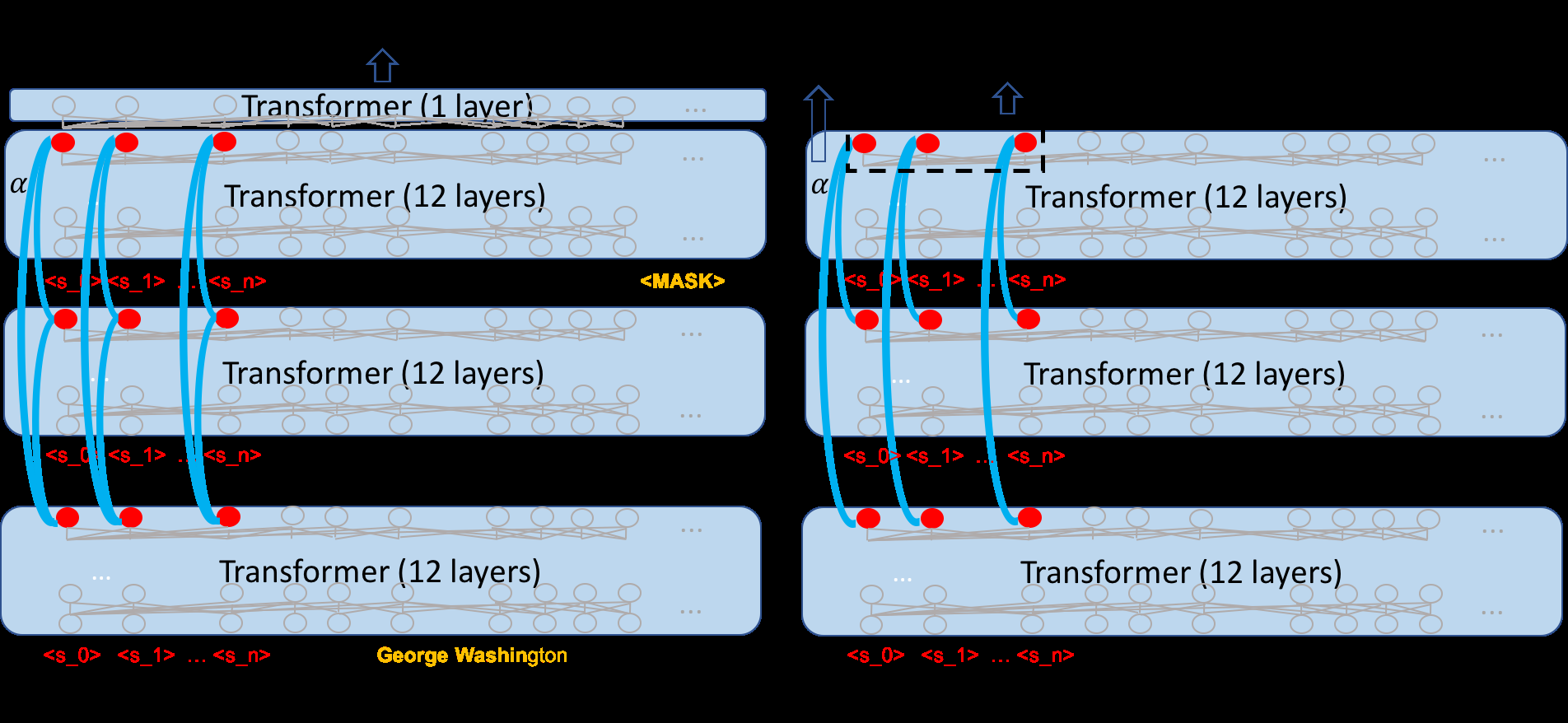
Transfer Learning and Distant Supervision for Multilingual Transformer Models: A Study on African Languages
Michael A. Hedderich, David Adelani, Dawei Zhu, Jesujoba Alabi, Udia Markus, Dietrich Klakow,
