Transfer Learning and Distant Supervision for Multilingual Transformer Models: A Study on African Languages
Michael A. Hedderich, David Adelani, Dawei Zhu, Jesujoba Alabi, Udia Markus, Dietrich Klakow
NLP Applications Short Paper
You can open the pre-recorded video in a separate window.
Abstract:
Multilingual transformer models like mBERT and XLM-RoBERTa have obtained great improvements for many NLP tasks on a variety of languages. However, recent works also showed that results from high-resource languages could not be easily transferred to realistic, low-resource scenarios. In this work, we study trends in performance for different amounts of available resources for the three African languages Hausa, isiXhosa and \yoruba on both NER and topic classification. We show that in combination with transfer learning or distant supervision, these models can achieve with as little as 10 or 100 labeled sentences the same performance as baselines with much more supervised training data. However, we also find settings where this does not hold. Our discussions and additional experiments on assumptions such as time and hardware restrictions highlight challenges and opportunities in low-resource learning.
NOTE: Video may display a random order of authors.
Correct author list is at the top of this page.
Connected Papers in EMNLP2020
Similar Papers
Multi-task Learning for Multilingual Neural Machine Translation
Yiren Wang, ChengXiang Zhai, Hany Hassan,
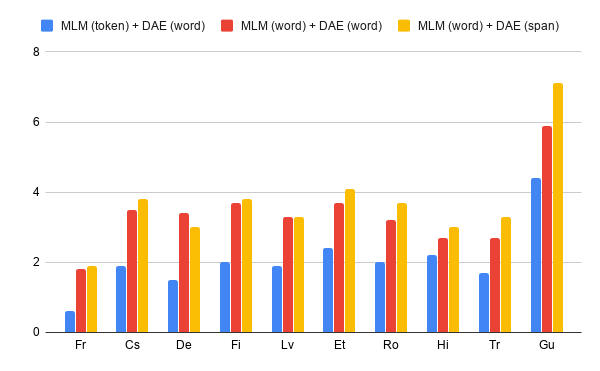
From Zero to Hero: On the Limitations of Zero-Shot Language Transfer with Multilingual Transformers
Anne Lauscher, Vinit Ravishankar, Ivan Vulić, Goran Glavaš,
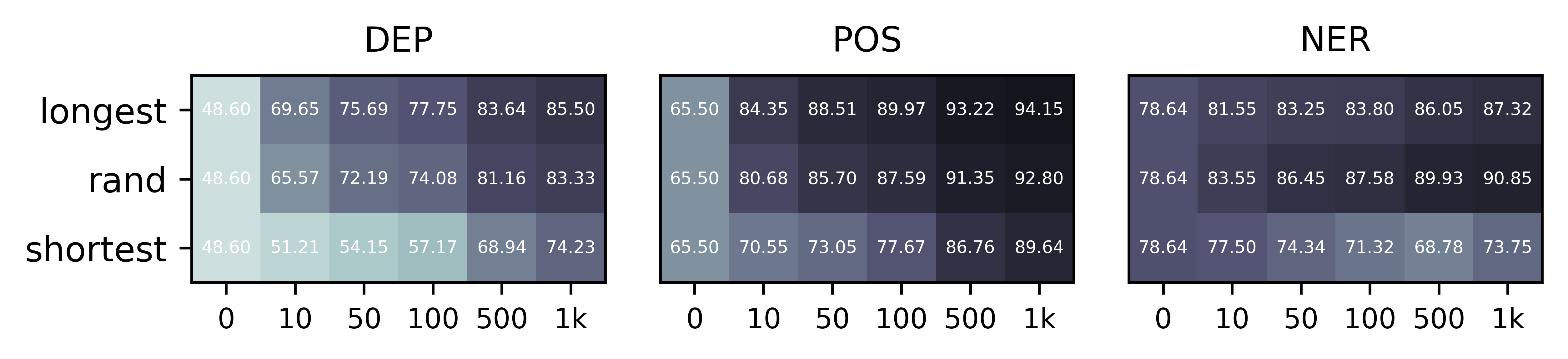
Don't Use English Dev: On the Zero-Shot Cross-Lingual Evaluation of Contextual Embeddings
Phillip Keung, Yichao Lu, Julian Salazar, Vikas Bhardwaj,
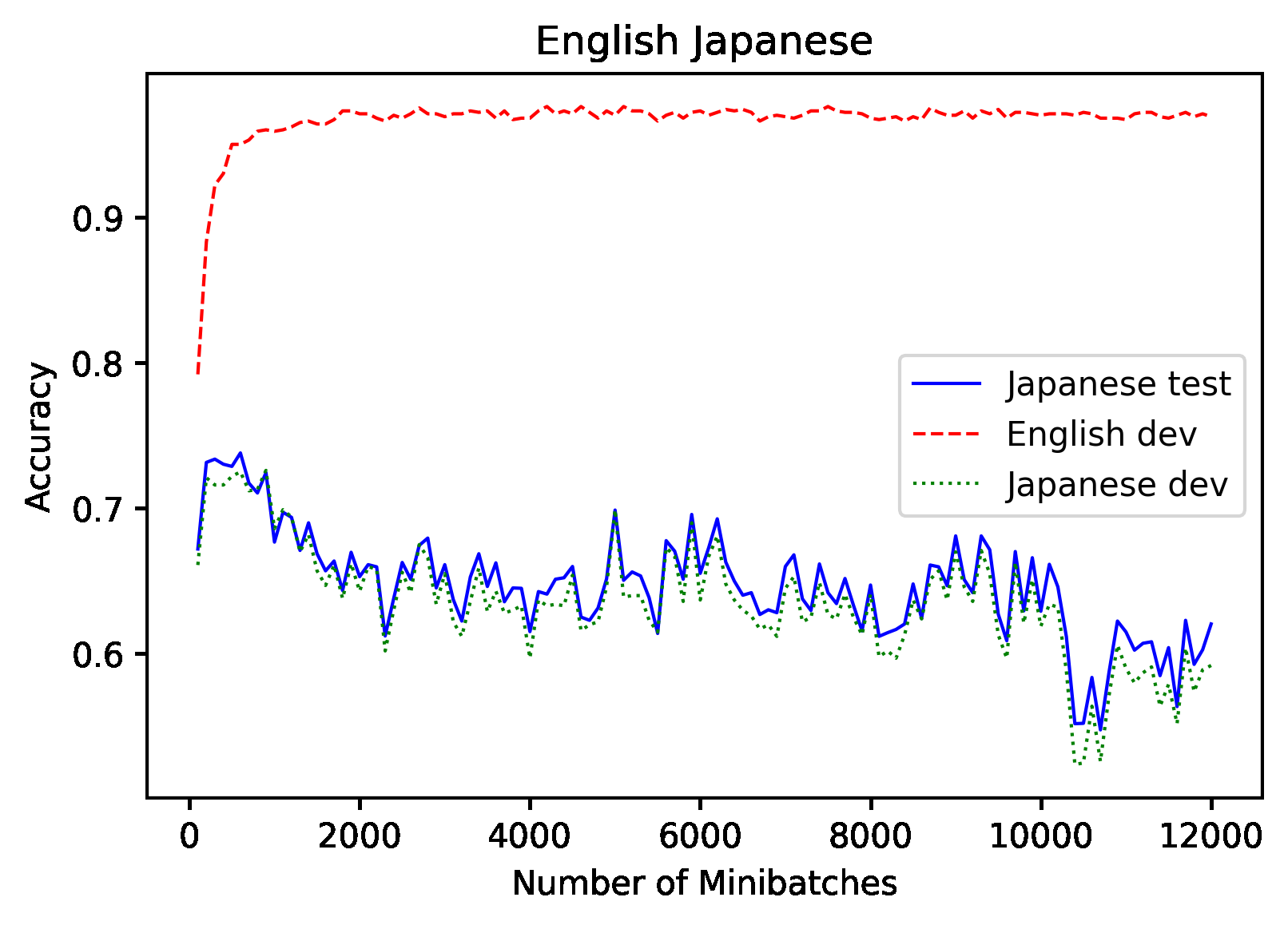