Cross-Thought for Sentence Encoder Pre-training
Shuohang Wang, Yuwei Fang, Siqi Sun, Zhe Gan, Yu Cheng, Jingjing Liu, Jing Jiang
Semantics: Sentence-level Semantics, Textual Inference and Other areas Long Paper
You can open the pre-recorded video in a separate window.
Abstract:
In this paper, we propose Cross-Thought, a novel approach to pre-training sequence encoder, which is instrumental in building reusable sequence embeddings for large-scale NLP tasks such as question answering. Instead of using the original signals of full sentences, we train a Transformer-based sequence encoder over a large set of short sequences, which allows the model to automatically select the most useful information for predicting masked words. Experiments on question answering and textual entailment tasks demonstrate that our pre-trained encoder can outperform state-of-the-art encoders trained with continuous sentence signals as well as traditional masked language modeling baselines. Our proposed approach also achieves new state of the art on HotpotQA (full-wiki setting) by improving intermediate information retrieval performance.
NOTE: Video may display a random order of authors.
Correct author list is at the top of this page.
Connected Papers in EMNLP2020
Similar Papers
Uncertainty-Aware Semantic Augmentation for Neural Machine Translation
Xiangpeng Wei, Heng Yu, Yue Hu, Rongxiang Weng, Luxi Xing, Weihua Luo,
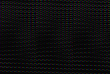
SLM: Learning a Discourse Language Representation with Sentence Unshuffling
Haejun Lee, Drew A. Hudson, Kangwook Lee, Christopher D. Manning,
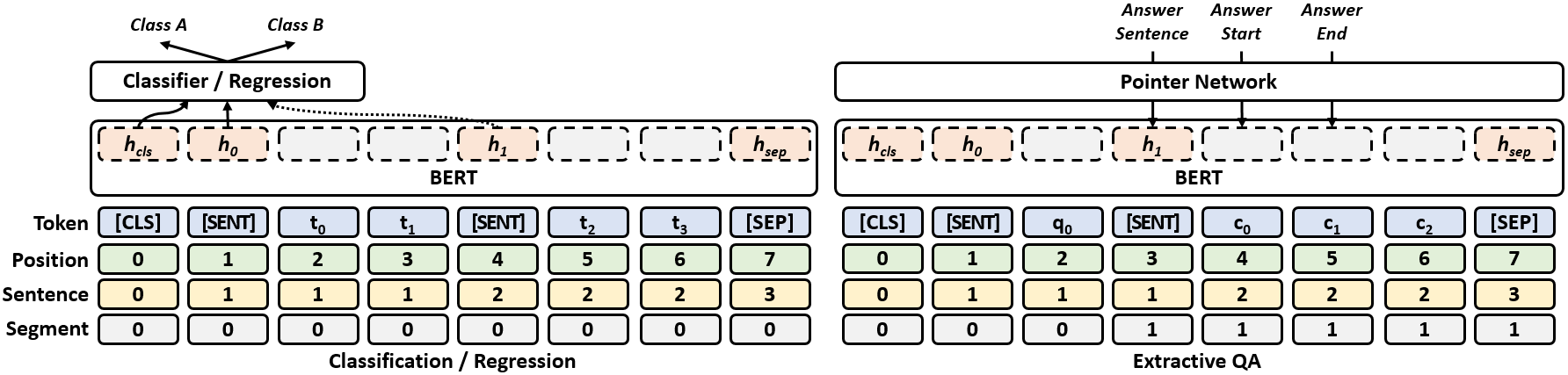
Augmented Natural Language for Generative Sequence Labeling
Ben Athiwaratkun, Cicero Nogueira dos Santos, Jason Krone, Bing Xiang,
