Factual Error Correction for Abstractive Summarization Models
Meng Cao, Yue Dong, Jiapeng Wu, Jackie Chi Kit Cheung
Summarization Short Paper
You can open the pre-recorded video in a separate window.
Abstract:
Neural abstractive summarization systems have achieved promising progress, thanks to the availability of large-scale datasets and models pre-trained with self-supervised methods. However, ensuring the factual consistency of the generated summaries for abstractive summarization systems is a challenge. We propose a post-editing corrector module to address this issue by identifying and correcting factual errors in generated summaries. The neural corrector model is pre-trained on artificial examples that are created by applying a series of heuristic transformations on reference summaries. These transformations are inspired by the error analysis of state-of-the-art summarization model outputs. Experimental results show that our model is able to correct factual errors in summaries generated by other neural summarization models and outperforms previous models on factual consistency evaluation on the CNN/DailyMail dataset. We also find that transferring from artificial error correction to downstream settings is still very challenging.
NOTE: Video may display a random order of authors.
Correct author list is at the top of this page.
Connected Papers in EMNLP2020
Similar Papers
Evaluating the Factual Consistency of Abstractive Text Summarization
Wojciech Kryscinski, Bryan McCann, Caiming Xiong, Richard Socher,

Pre-training for Abstractive Document Summarization by Reinstating Source Text
Yanyan Zou, Xingxing Zhang, Wei Lu, Furu Wei, Ming Zhou,
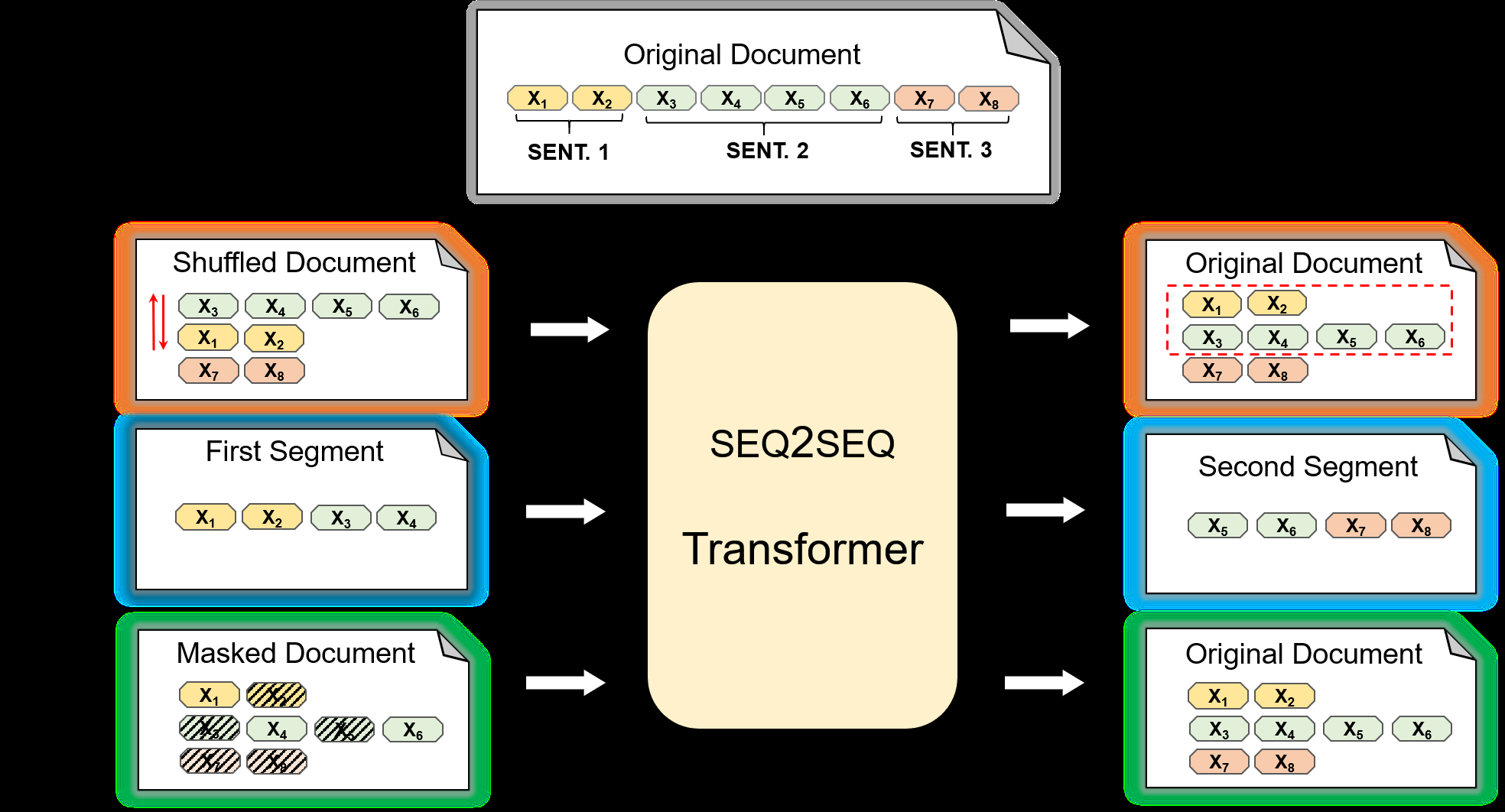
Understanding Neural Abstractive Summarization Models via Uncertainty
Jiacheng Xu, Shrey Desai, Greg Durrett,
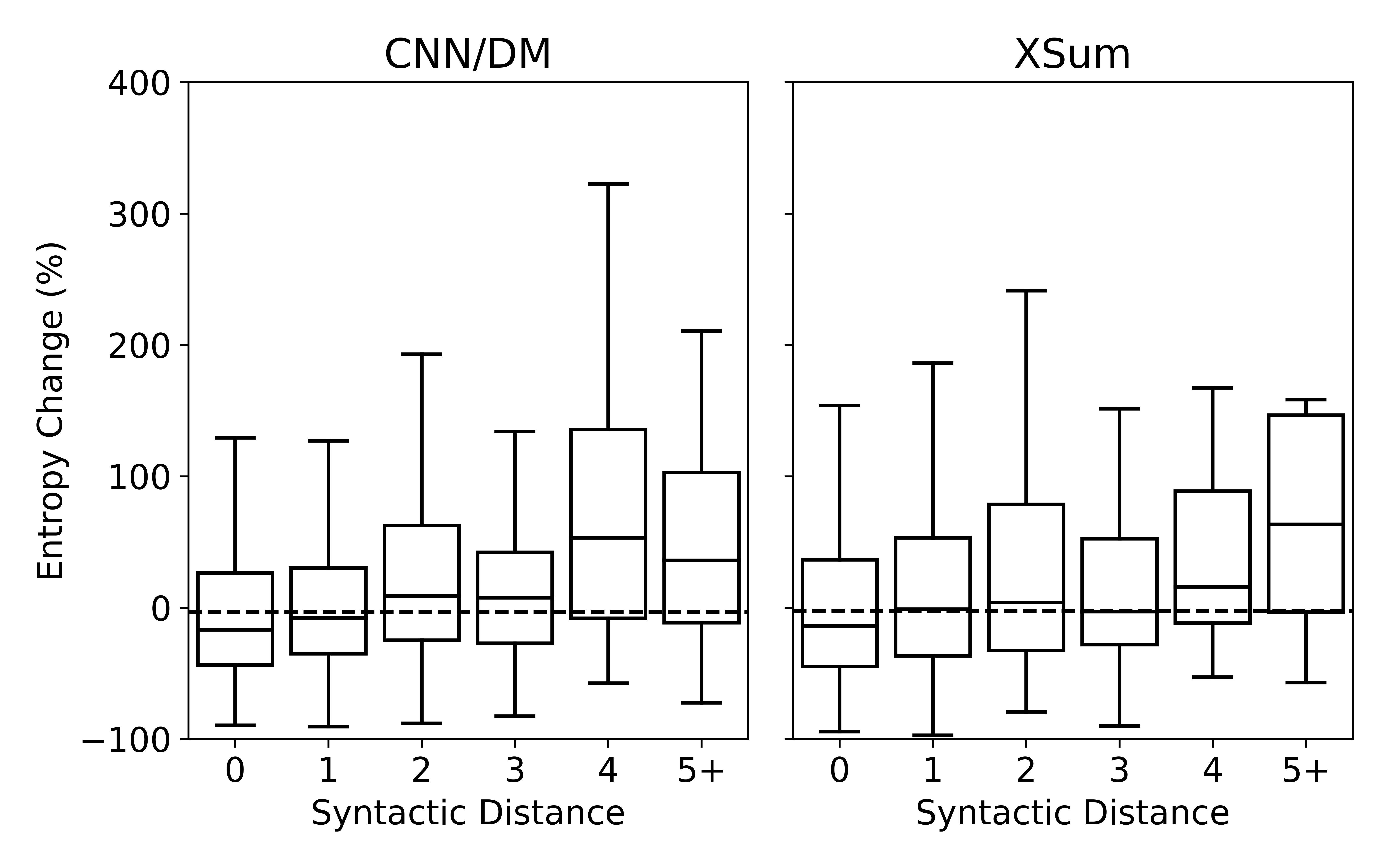
On Extractive and Abstractive Neural Document Summarization with Transformer Language Models
Jonathan Pilault, Raymond Li, Sandeep Subramanian, Chris Pal,
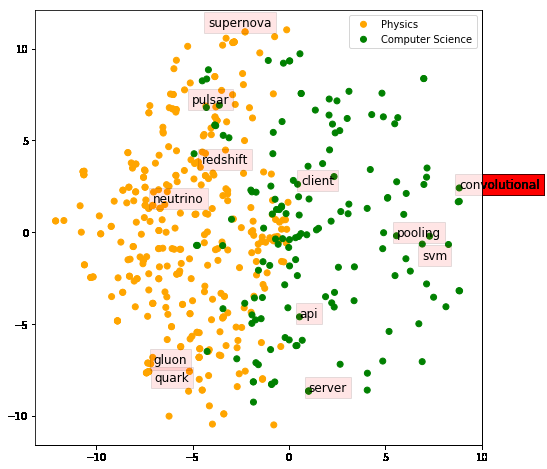