COGS: A Compositional Generalization Challenge Based on Semantic Interpretation
Najoung Kim, Tal Linzen
Interpretability and Analysis of Models for NLP Long Paper
You can open the pre-recorded video in a separate window.
Abstract:
Natural language is characterized by compositionality: the meaning of a complex expression is constructed from the meanings of its constituent parts. To facilitate the evaluation of the compositional abilities of language processing architectures, we introduce COGS, a semantic parsing dataset based on a fragment of English. The evaluation portion of COGS contains multiple systematic gaps that can only be addressed by compositional generalization; these include new combinations of familiar syntactic structures, or new combinations of familiar words and familiar structures. In experiments with Transformers and LSTMs, we found that in-distribution accuracy on the COGS test set was near-perfect (96--99%), but generalization accuracy was substantially lower (16--35%) and showed high sensitivity to random seed (+-6--8%). These findings indicate that contemporary standard NLP models are limited in their compositional generalization capacity, and position COGS as a good way to measure progress.
NOTE: Video may display a random order of authors.
Correct author list is at the top of this page.
Connected Papers in EMNLP2020
Similar Papers
Compositional and Lexical Semantics in RoBERTa, BERT and DistilBERT: A Case Study on CoQA
Ieva Staliūnaitė, Ignacio Iacobacci,

XL-WiC: A Multilingual Benchmark for Evaluating Semantic Contextualization
Alessandro Raganato, Tommaso Pasini, Jose Camacho-Collados, Mohammad Taher Pilehvar,
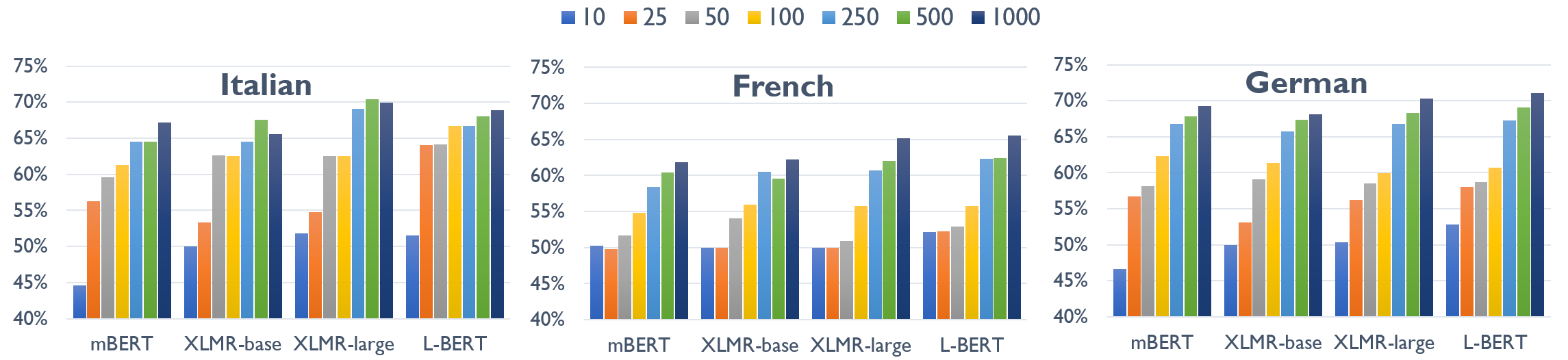
COD3S: Diverse Generation with Discrete Semantic Signatures
Nathaniel Weir, João Sedoc, Benjamin Van Durme,
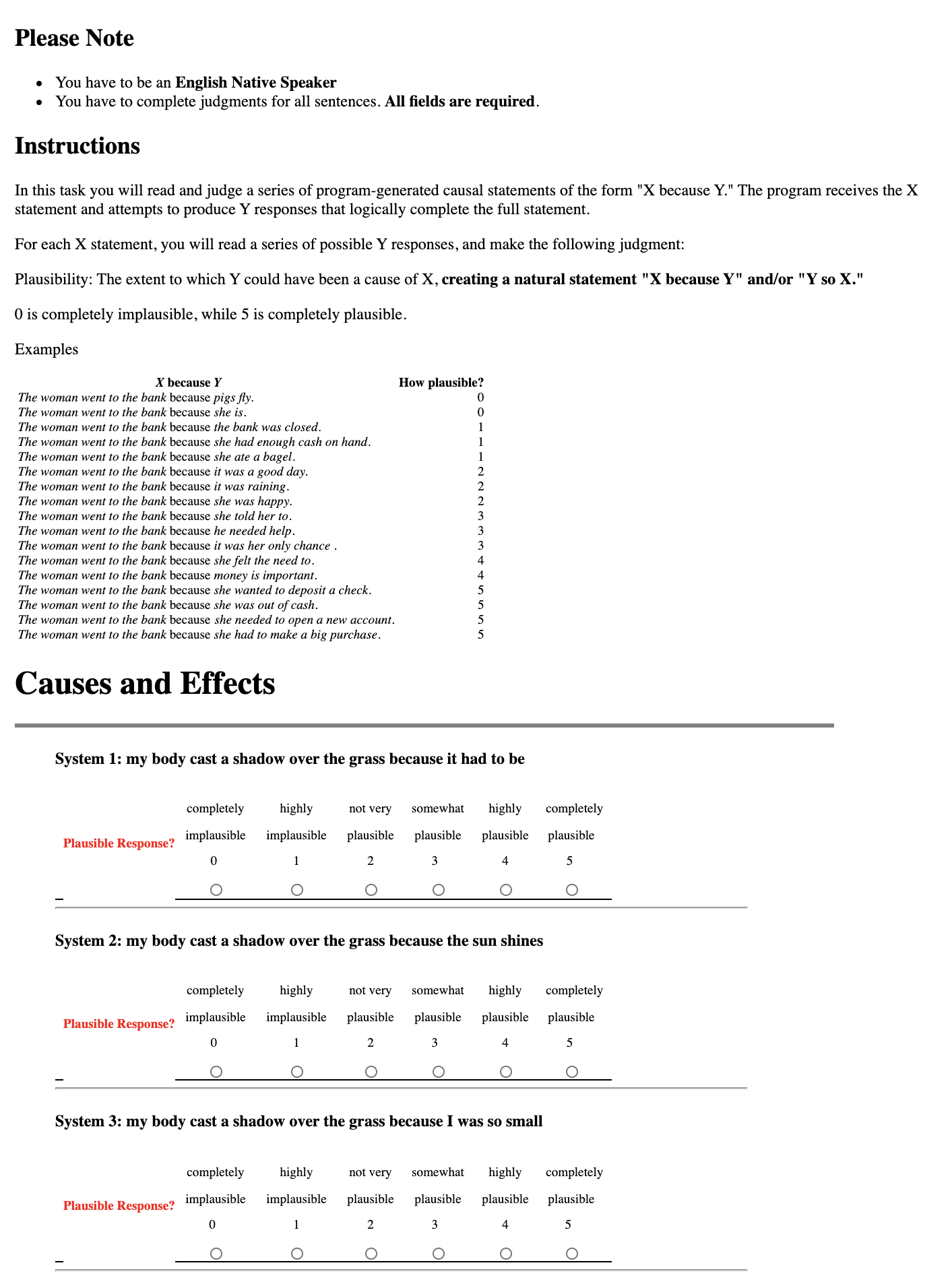