COD3S: Diverse Generation with Discrete Semantic Signatures
Nathaniel Weir, João Sedoc, Benjamin Van Durme
Language Generation Short Paper
You can open the pre-recorded video in a separate window.
Abstract:
We present COD3S, a novel method for generating semantically diverse sentences using neural sequence-to-sequence (seq2seq) models. Conditioned on an input, seq2seqs typically produce semantically and syntactically homogeneous sets of sentences and thus perform poorly on one-to-many sequence generation tasks. Our two-stage approach improves output diversity by conditioning generation on locality-sensitive hash (LSH)-based semantic sentence codes whose Hamming distances highly correlate with human judgments of semantic textual similarity. Though it is generally applicable, we apply \method{} to causal generation, the task of predicting a proposition's plausible causes or effects. We demonstrate through automatic and human evaluation that responses produced using our method exhibit improved diversity without degrading task performance.
NOTE: Video may display a random order of authors.
Correct author list is at the top of this page.
Connected Papers in EMNLP2020
Similar Papers
Understanding Neural Abstractive Summarization Models via Uncertainty
Jiacheng Xu, Shrey Desai, Greg Durrett,
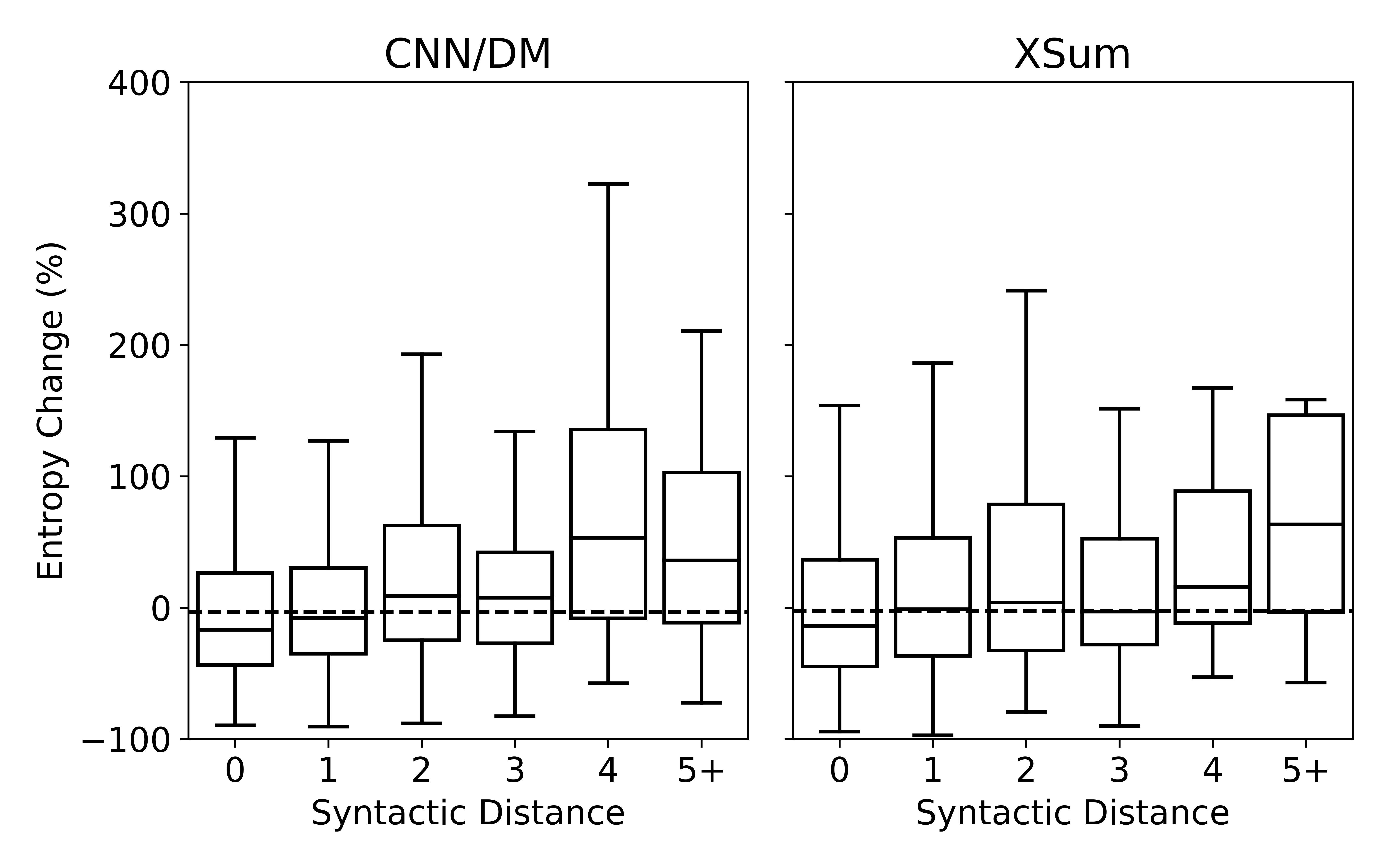
BLiMP: The Benchmark of Linguistic Minimal Pairs for English
Alex Warstadt, Alicia Parrish, Haokun Liu, Anhad Monananey, Wei Peng, Sheng-Fu Wang, Samuel Bowman,
