Compositional and Lexical Semantics in RoBERTa, BERT and DistilBERT: A Case Study on CoQA
Ieva Staliūnaitė, Ignacio Iacobacci
Interpretability and Analysis of Models for NLP Long Paper
You can open the pre-recorded video in a separate window.
Abstract:
Many NLP tasks have benefited from transferring knowledge from contextualized word embeddings, however the picture of what type of knowledge is transferred is incomplete. This paper studies the types of linguistic phenomena accounted for by language models in the context of a Conversational Question Answering (CoQA) task. We identify the problematic areas for the finetuned RoBERTa, BERT and DistilBERT models through systematic error analysis - basic arithmetic (counting phrases), compositional semantics (negation and Semantic Role Labeling), and lexical semantics (surprisal and antonymy). When enhanced with the relevant linguistic knowledge through multitask learning, the models improve in performance. Ensembles of the enhanced models yield a boost between 2.2 and 2.7 points in F1 score overall, and up to 42.1 points in F1 on the hardest question classes. The results show differences in ability to represent compositional and lexical information between RoBERTa, BERT and DistilBERT.
NOTE: Video may display a random order of authors.
Correct author list is at the top of this page.
Connected Papers in EMNLP2020
Similar Papers
XL-WiC: A Multilingual Benchmark for Evaluating Semantic Contextualization
Alessandro Raganato, Tommaso Pasini, Jose Camacho-Collados, Mohammad Taher Pilehvar,
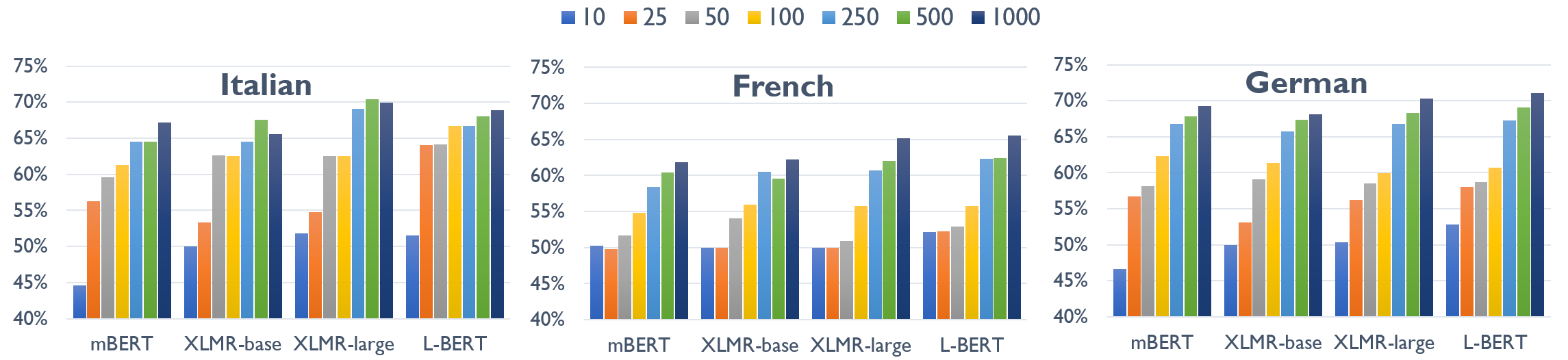
Syntactic Structure Distillation Pretraining for Bidirectional Encoders
Adhiguna Kuncoro, Lingpeng Kong, Daniel Fried, Dani Yogatama, Laura Rimell, Chris Dyer, Phil Blunsom,

BLiMP: The Benchmark of Linguistic Minimal Pairs for English
Alex Warstadt, Alicia Parrish, Haokun Liu, Anhad Monananey, Wei Peng, Sheng-Fu Wang, Samuel Bowman,

COGS: A Compositional Generalization Challenge Based on Semantic Interpretation
Najoung Kim, Tal Linzen,
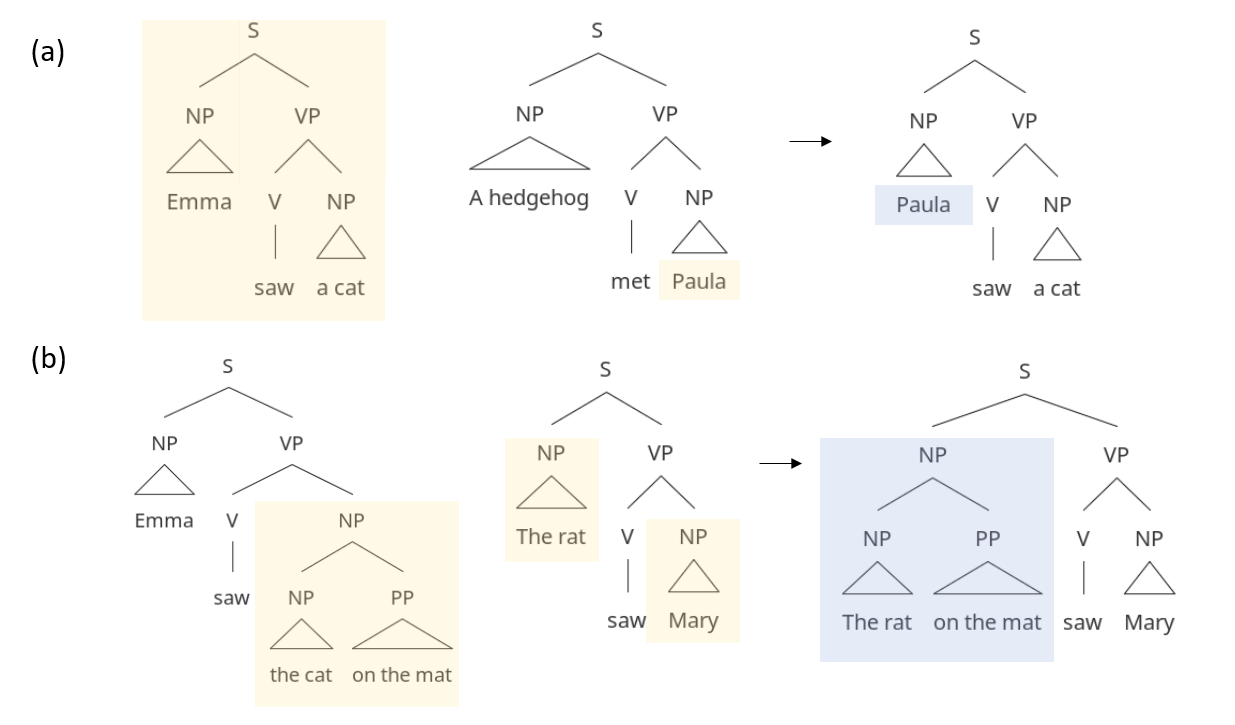