Retrofitting Structure-aware Transformer Language Model for End Tasks
Hao Fei, Yafeng Ren, Donghong Ji
Interpretability and Analysis of Models for NLP Long Paper
You can open the pre-recorded video in a separate window.
Abstract:
We consider retrofitting structure-aware Transformer language model for facilitating end tasks by proposing to exploit syntactic distance to encode both the phrasal constituency and dependency connection into the language model. A middle-layer structural learning strategy is leveraged for structure integration, accomplished with main semantic task training under multi-task learning scheme. Experimental results show that the retrofitted structure-aware Transformer language model achieves improved perplexity, meanwhile inducing accurate syntactic phrases. By performing structure-aware fine-tuning, our model achieves significant improvements for both semantic- and syntactic-dependent tasks.
NOTE: Video may display a random order of authors.
Correct author list is at the top of this page.
Connected Papers in EMNLP2020
Similar Papers
ETC: Encoding Long and Structured Inputs in Transformers
Joshua Ainslie, Santiago Ontanon, Chris Alberti, Vaclav Cvicek, Zachary Fisher, Philip Pham, Anirudh Ravula, Sumit Sanghai, Qifan Wang, Li Yang,
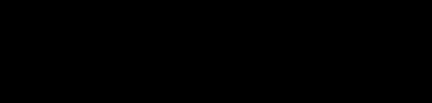
KERMIT: Complementing Transformer Architectures with Encoders of Explicit Syntactic Interpretations
Fabio Massimo Zanzotto, Andrea Santilli, Leonardo Ranaldi, Dario Onorati, Pierfrancesco Tommasino, Francesca Fallucchi,
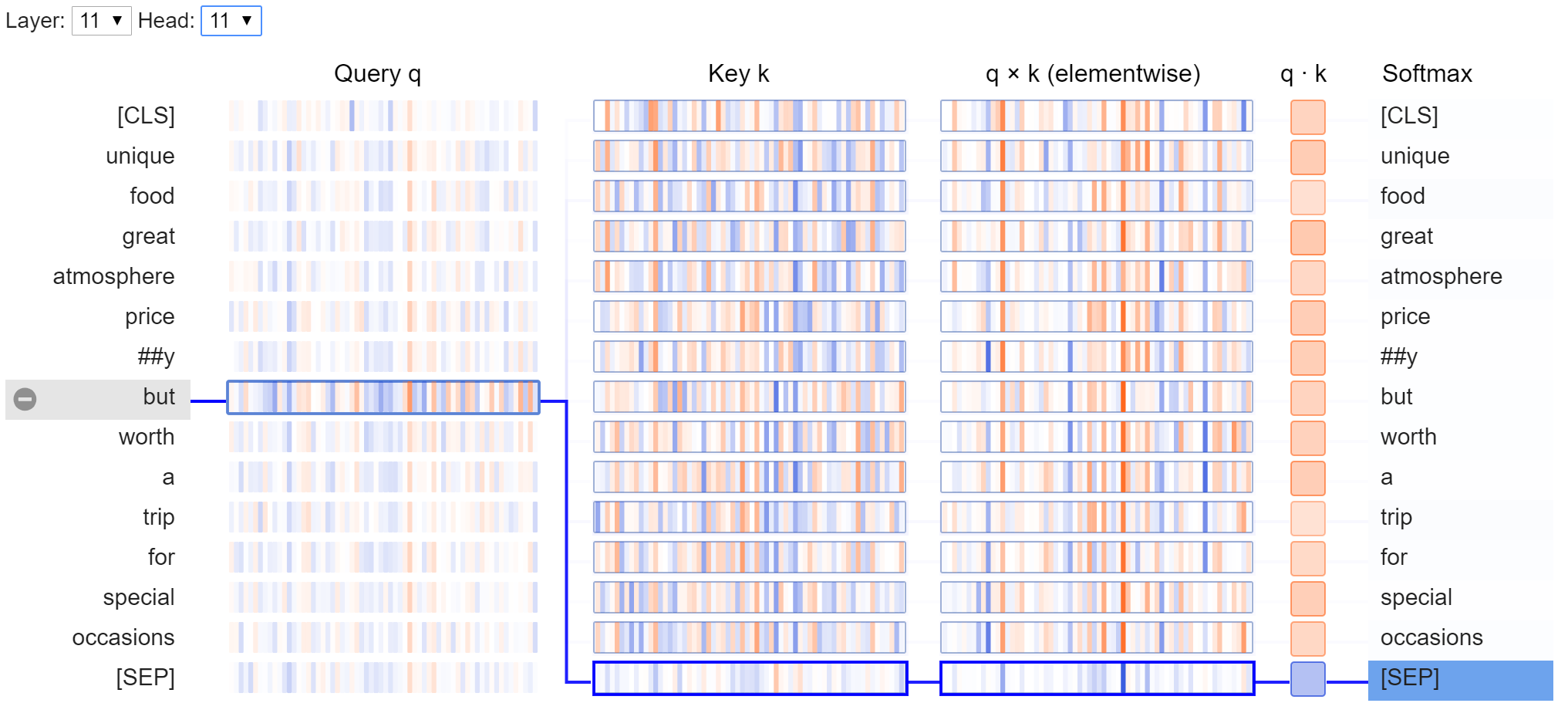
Cross-Thought for Sentence Encoder Pre-training
Shuohang Wang, Yuwei Fang, Siqi Sun, Zhe Gan, Yu Cheng, Jingjing Liu, Jing Jiang,
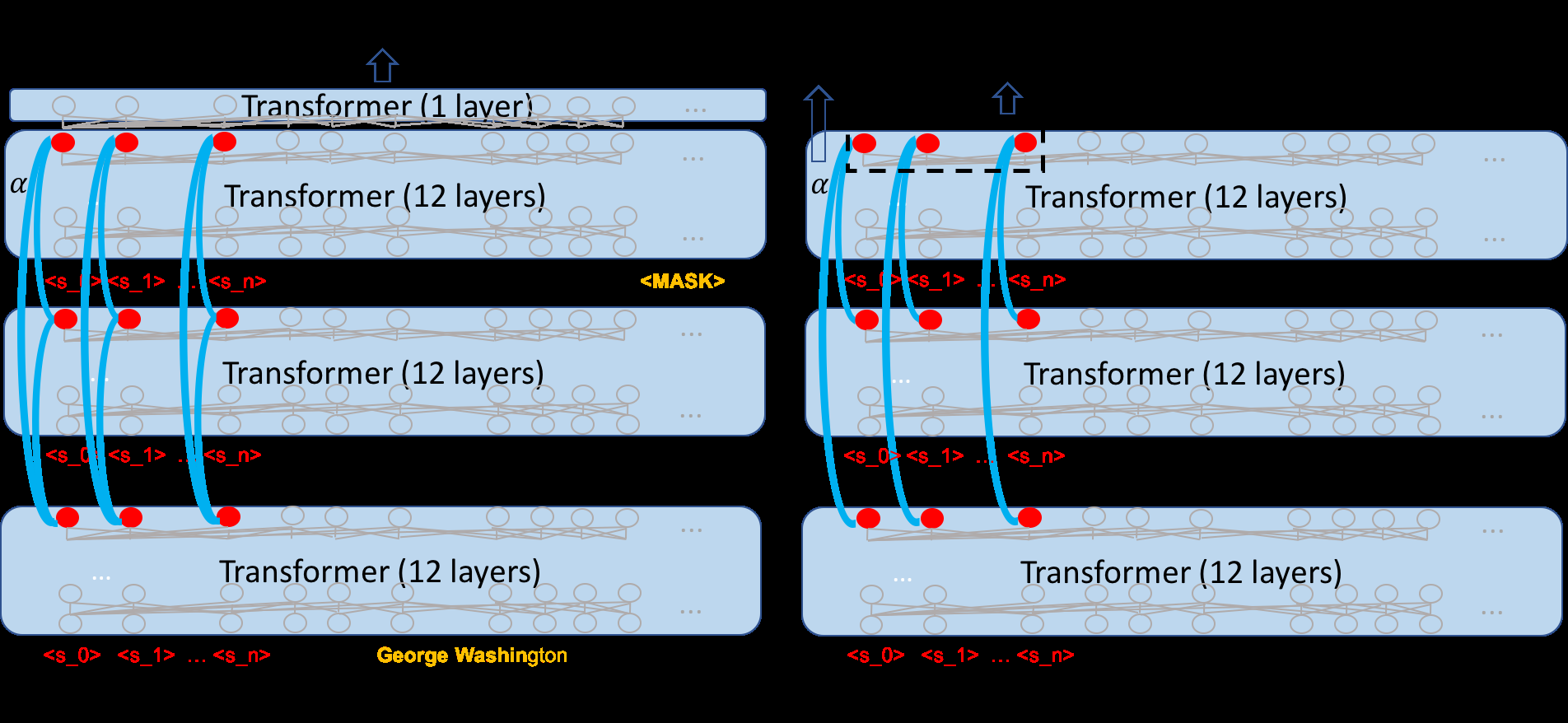