On the importance of pre-training data volume for compact language models
Vincent Micheli, Martin d'Hoffschmidt, François Fleuret
Machine Learning for NLP Short Paper
You can open the pre-recorded video in a separate window.
Abstract:
Recent advances in language modeling have led to computationally intensive and resource-demanding state-of-the-art models. In an effort towards sustainable practices, we study the impact of pre-training data volume on compact language models. Multiple BERT-based models are trained on gradually increasing amounts of French text. Through fine-tuning on the French Question Answering Dataset (FQuAD), we observe that well-performing models are obtained with as little as 100 MB of text. In addition, we show that past critically low amounts of pre-training data, an intermediate pre-training step on the task-specific corpus does not yield substantial improvements.
NOTE: Video may display a random order of authors.
Correct author list is at the top of this page.
Connected Papers in EMNLP2020
Similar Papers
On the Sparsity of Neural Machine Translation Models
Yong Wang, Longyue Wang, Victor Li, Zhaopeng Tu,

Data Weighted Training Strategies for Grammatical Error Correction
Jared Lichtarge, Chris Alberti, Shankar Kumar,

An Empirical Investigation Towards Efficient Multi-Domain Language Model Pre-training
Kristjan Arumae, Qing Sun, Parminder Bhatia,
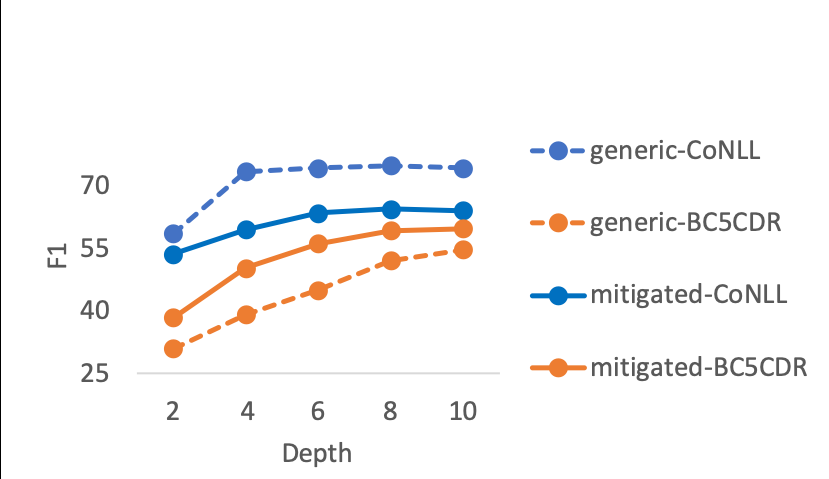
Multi-Stage Pre-training for Low-Resource Domain Adaptation
Rong Zhang, Revanth Gangi Reddy, Md Arafat Sultan, Vittorio Castelli, Anthony Ferritto, Radu Florian, Efsun Sarioglu Kayi, Salim Roukos, Avi Sil, Todd Ward,
