Distilling Multiple Domains for Neural Machine Translation
Anna Currey, Prashant Mathur, Georgiana Dinu
Machine Translation and Multilinguality Long Paper
You can open the pre-recorded video in a separate window.
Abstract:
Neural machine translation achieves impressive results in high-resource conditions, but performance often suffers when the input domain is low-resource. The standard practice of adapting a separate model for each domain of interest does not scale well in practice from both a quality perspective (brittleness under domain shift) as well as a cost perspective (added maintenance and inference complexity). In this paper, we propose a framework for training a single multi-domain neural machine translation model that is able to translate several domains without increasing inference time or memory usage. We show that this model can improve translation on both high- and low-resource domains over strong multi-domain baselines. In addition, our proposed model is effective when domain labels are unknown during training, as well as robust under noisy data conditions.
NOTE: Video may display a random order of authors.
Correct author list is at the top of this page.
Connected Papers in EMNLP2020
Similar Papers
On the Sparsity of Neural Machine Translation Models
Yong Wang, Longyue Wang, Victor Li, Zhaopeng Tu,

Uncertainty-Aware Semantic Augmentation for Neural Machine Translation
Xiangpeng Wei, Heng Yu, Yue Hu, Rongxiang Weng, Luxi Xing, Weihua Luo,
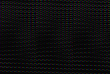
Long-Short Term Masking Transformer: A Simple but Effective Baseline for Document-level Neural Machine Translation
Pei Zhang, Boxing Chen, Niyu Ge, Kai Fan,
