Recall and Learn: Fine-tuning Deep Pretrained Language Models with Less Forgetting
Sanyuan Chen, Yutai Hou, Yiming Cui, Wanxiang Che, Ting Liu, Xiangzhan Yu
Machine Learning for NLP Long Paper
You can open the pre-recorded video in a separate window.
Abstract:
Deep pretrained language models have achieved great success in the way of pretraining first and then fine-tuning. But such a sequential transfer learning paradigm often confronts the catastrophic forgetting problem and leads to sub-optimal performance. To fine-tune with less forgetting, we propose a recall and learn mechanism, which adopts the idea of multi-task learning and jointly learns pretraining tasks and downstream tasks. Specifically, we introduce a Pretraining Simulation mechanism to recall the knowledge from pretraining tasks without data, and an Objective Shifting mechanism to focus the learning on downstream tasks gradually. Experiments show that our method achieves state-of-the-art performance on the GLUE benchmark. Our method also enables BERT-base to achieve better average performance than directly fine-tuning of BERT-large. Further, we provide the open-source RecAdam optimizer, which integrates the proposed mechanisms into Adam optimizer, to facility the NLP community.
NOTE: Video may display a random order of authors.
Correct author list is at the top of this page.
Connected Papers in EMNLP2020
Similar Papers
Train No Evil: Selective Masking for Task-Guided Pre-Training
Yuxian Gu, Zhengyan Zhang, Xiaozhi Wang, Zhiyuan Liu, Maosong Sun,
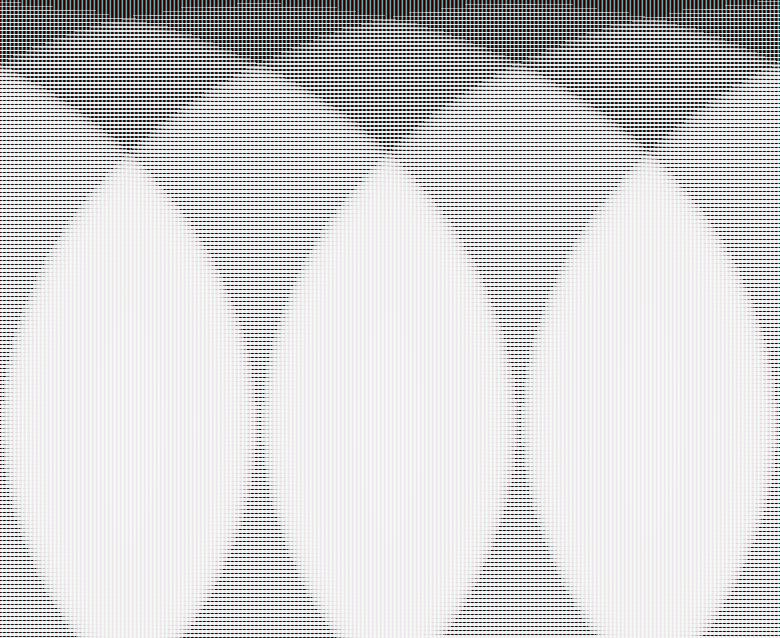
oLMpics - On what Language Model Pre-training Captures
Alon Talmor, Yanai Elazar, Yoav Goldberg, Jonathan Berant,

Zero-Shot Cross-Lingual Transfer with Meta Learning
Farhad Nooralahzadeh, Giannis Bekoulis, Johannes Bjerva, Isabelle Augenstein,
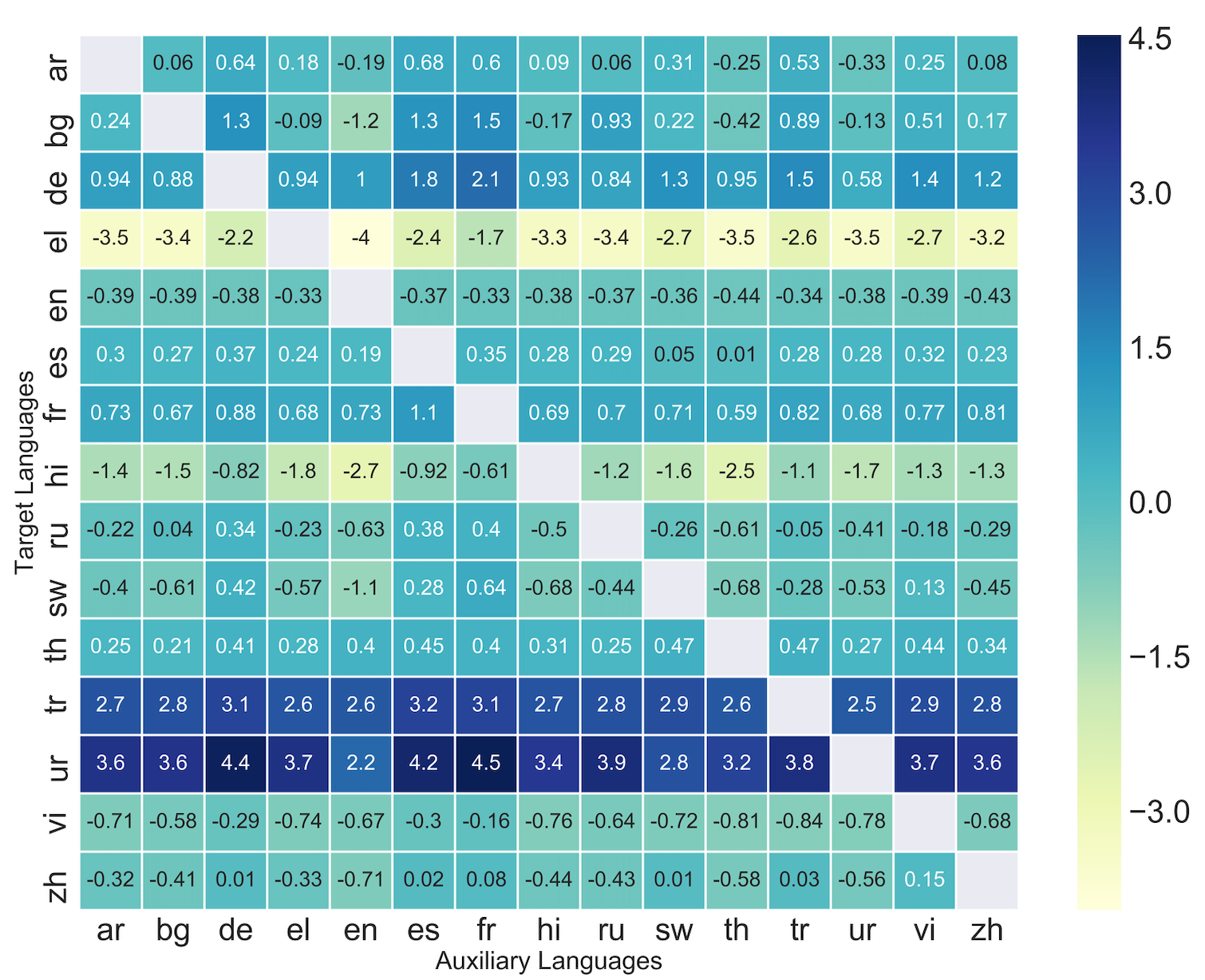