Zero-Shot Cross-Lingual Transfer with Meta Learning
Farhad Nooralahzadeh, Giannis Bekoulis, Johannes Bjerva, Isabelle Augenstein
Machine Translation and Multilinguality Long Paper
You can open the pre-recorded video in a separate window.
Abstract:
Learning what to share between tasks has become a topic of great importance, as strategic sharing of knowledge has been shown to improve downstream task performance. This is particularly important for multilingual applications, as most languages in the world are under-resourced. Here, we consider the setting of training models on multiple different languages at the same time, when little or no data is available for languages other than English. We show that this challenging setup can be approached using meta-learning: in addition to training a source language model, another model learns to select which training instances are the most beneficial to the first. We experiment using standard supervised, zero-shot cross-lingual, as well as few-shot cross-lingual settings for different natural language understanding tasks (natural language inference, question answering). Our extensive experimental setup demonstrates the consistent effectiveness of meta-learning for a total of 15 languages. We improve upon the state-of-the-art for zero-shot and few-shot NLI (on MultiNLI and XNLI) and QA (on the MLQA dataset). A comprehensive error analysis indicates that the correlation of typological features between languages can partly explain when parameter sharing learned via meta-learning is beneficial.
NOTE: Video may display a random order of authors.
Correct author list is at the top of this page.
Connected Papers in EMNLP2020
Similar Papers
MAD-X: An Adapter-Based Framework for Multi-Task Cross-Lingual Transfer
Jonas Pfeiffer, Ivan Vulić, Iryna Gurevych, Sebastian Ruder,

Cross-lingual Spoken Language Understanding with Regularized Representation Alignment
Zihan Liu, Genta Indra Winata, Peng Xu, Zhaojiang Lin, Pascale Fung,

Learn to Cross-lingual Transfer with Meta Graph Learning Across Heterogeneous Languages
Zheng Li, Mukul Kumar, William Headden, Bing Yin, Ying Wei, Yu Zhang, Qiang Yang,

From Zero to Hero: On the Limitations of Zero-Shot Language Transfer with Multilingual Transformers
Anne Lauscher, Vinit Ravishankar, Ivan Vulić, Goran Glavaš,
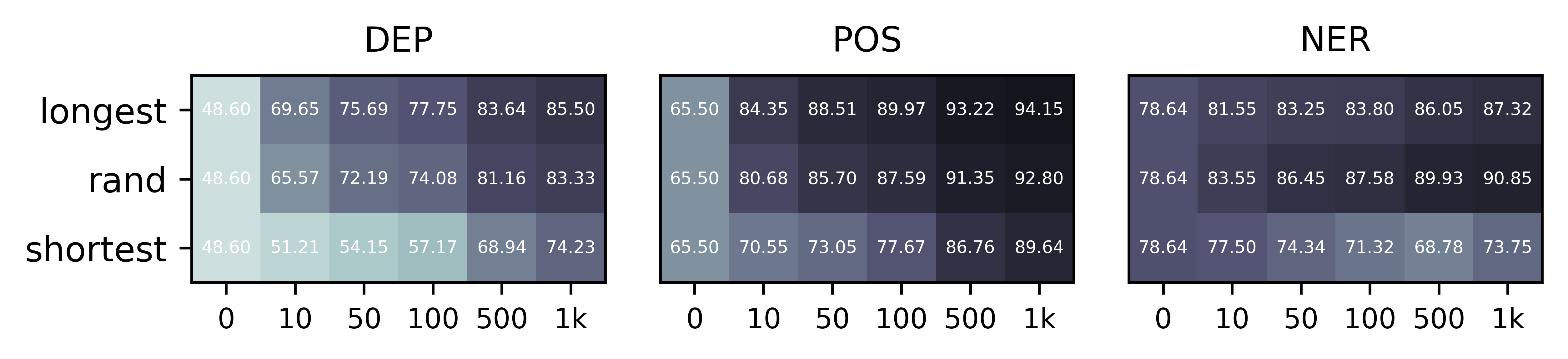