Authorship Attribution for Neural Text Generation
Adaku Uchendu, Thai Le, Kai Shu, Dongwon Lee
NLP Applications Long Paper
You can open the pre-recorded video in a separate window.
Abstract:
In recent years, the task of generating realistic short and long texts have made tremendous advancements. In particular, several recently proposed neural network-based language models have demonstrated their astonishing capabilities to generate texts that are challenging to distinguish from human-written texts with the naked eye. Despite many benefits and utilities of such neural methods, in some applications, being able to tell the “author” of a text in question becomes critically important. In this work, in the context of this Turing Test, we investigate the so-called authorship attribution problem in three versions: (1) given two texts T1 and T2, are both generated by the same method or not? (2) is the given text T written by a human or machine? (3) given a text T and k candidate neural methods, can we single out the method (among k alternatives) that generated T? Against one humanwritten and eight machine-generated texts (i.e., CTRL, GPT, GPT2, GROVER, XLM, XLNET, PPLM, FAIR), we empirically experiment with the performance of various models in three problems. By and large, we find that most generators still generate texts significantly different from human-written ones, thereby making three problems easier to solve. However, the qualities of texts generated by GPT2, GROVER, and FAIR are better, often confusing machine classifiers in solving three problems. All codes and datasets of our experiments are available at: https://bit.ly/ 302zWdz
NOTE: Video may display a random order of authors.
Correct author list is at the top of this page.
Connected Papers in EMNLP2020
Similar Papers
Understanding Neural Abstractive Summarization Models via Uncertainty
Jiacheng Xu, Shrey Desai, Greg Durrett,
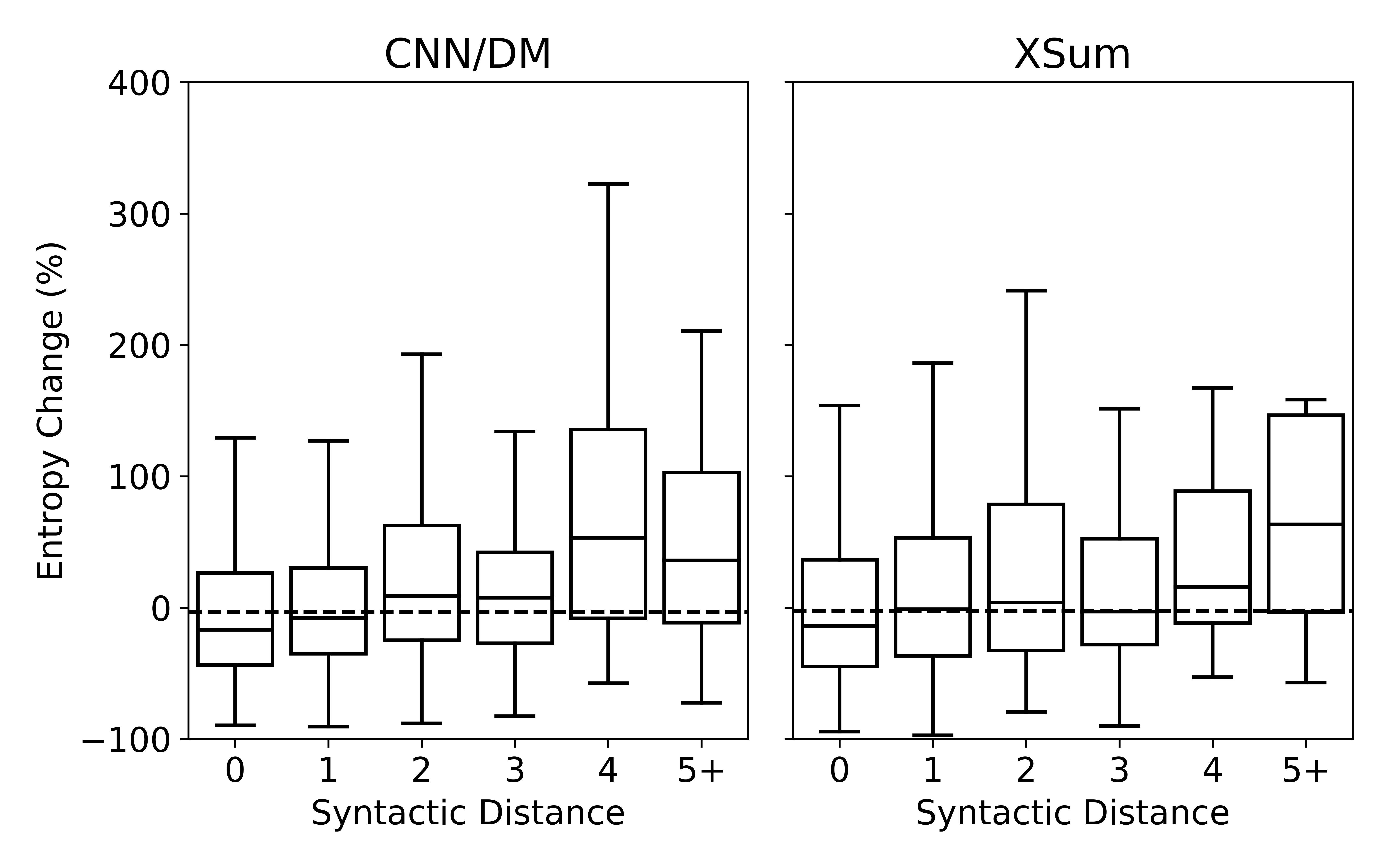
Neural Deepfake Detection with Factual Structure of Text
Wanjun Zhong, Duyu Tang, Zenan Xu, Ruize Wang, Nan Duan, Ming Zhou, Jiahai Wang, Jian Yin,
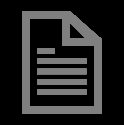