Sparse Text Generation
Pedro Henrique Martins, Zita Marinho, André F. T. Martins
Language Generation Long Paper
You can open the pre-recorded video in a separate window.
Abstract:
Current state-of-the-art text generators build on powerful language models such as GPT-2, achieving impressive performance. However, to avoid degenerate text, they require sampling from a modified softmax, via temperature parameters or ad-hoc truncation techniques, as in top-$k$ or nucleus sampling. This creates a mismatch between training and testing conditions. In this paper, we use the recently introduced entmax transformation to train and sample from a natively sparse language model, avoiding this mismatch. The result is a text generator with favorable performance in terms of fluency and consistency, fewer repetitions, and n-gram diversity closer to human text. In order to evaluate our model, we propose three new metrics for comparing sparse or truncated distributions: $\epsilon$-perplexity, sparsemax score, and Jensen-Shannon divergence. Human-evaluated experiments in story completion and dialogue generation show that entmax sampling leads to more engaging and coherent stories and conversations.
NOTE: Video may display a random order of authors.
Correct author list is at the top of this page.
Connected Papers in EMNLP2020
Similar Papers
POINTER: Constrained Progressive Text Generation via Insertion-based Generative Pre-training
Yizhe Zhang, Guoyin Wang, Chunyuan Li, Zhe Gan, Chris Brockett, Bill Dolan,
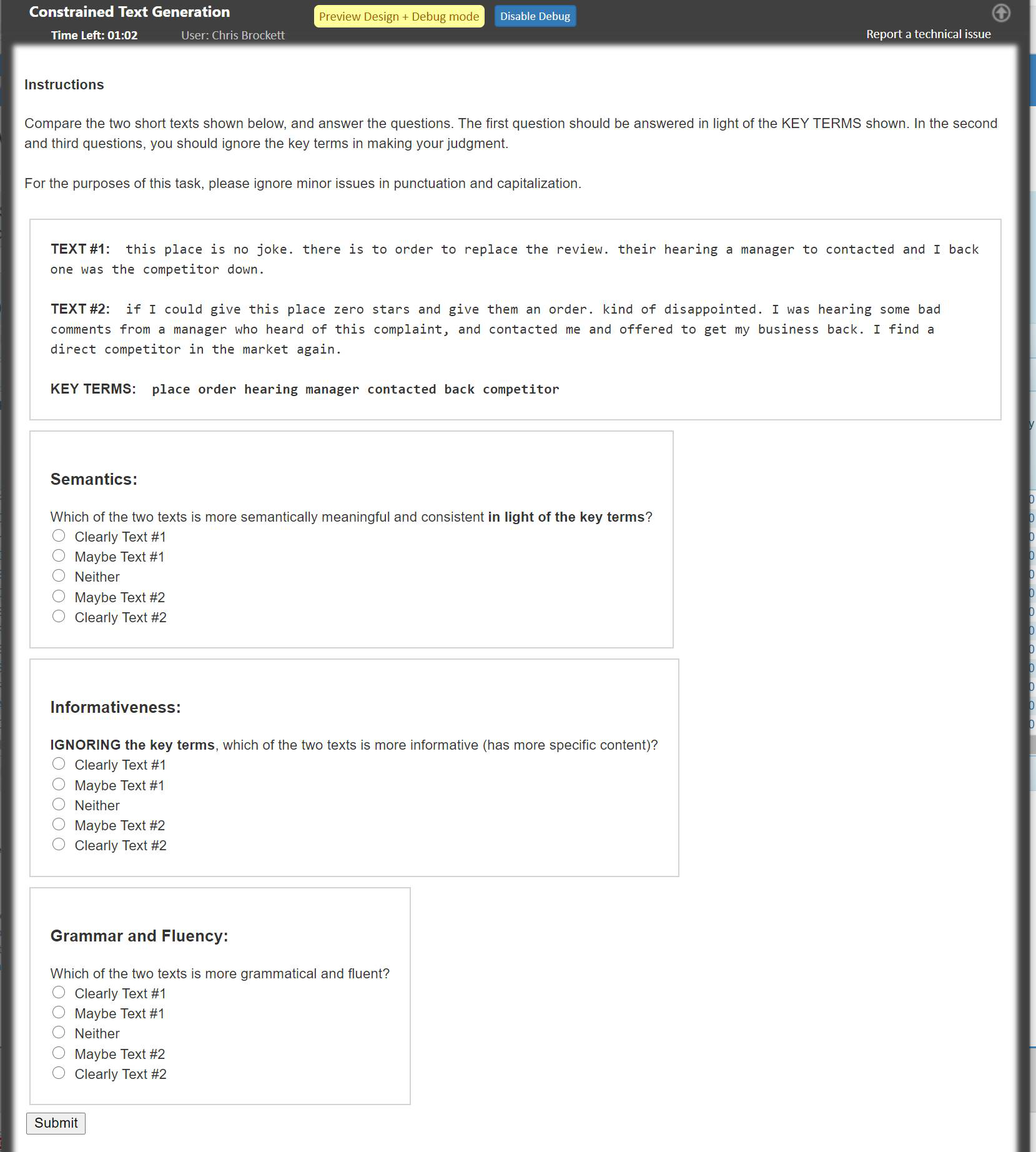
Improving Text Generation with Student-Forcing Optimal Transport
Jianqiao Li, Chunyuan Li, Guoyin Wang, Hao Fu, Yuhchen Lin, Liqun Chen, Yizhe Zhang, Chenyang Tao, Ruiyi Zhang, Wenlin Wang, Dinghan Shen, Qian Yang, Lawrence Carin,
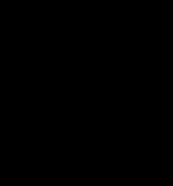
Training for Gibbs Sampling on Conditional Random Fields with Neural Scoring Factors
Sida Gao, Matthew R. Gormley,
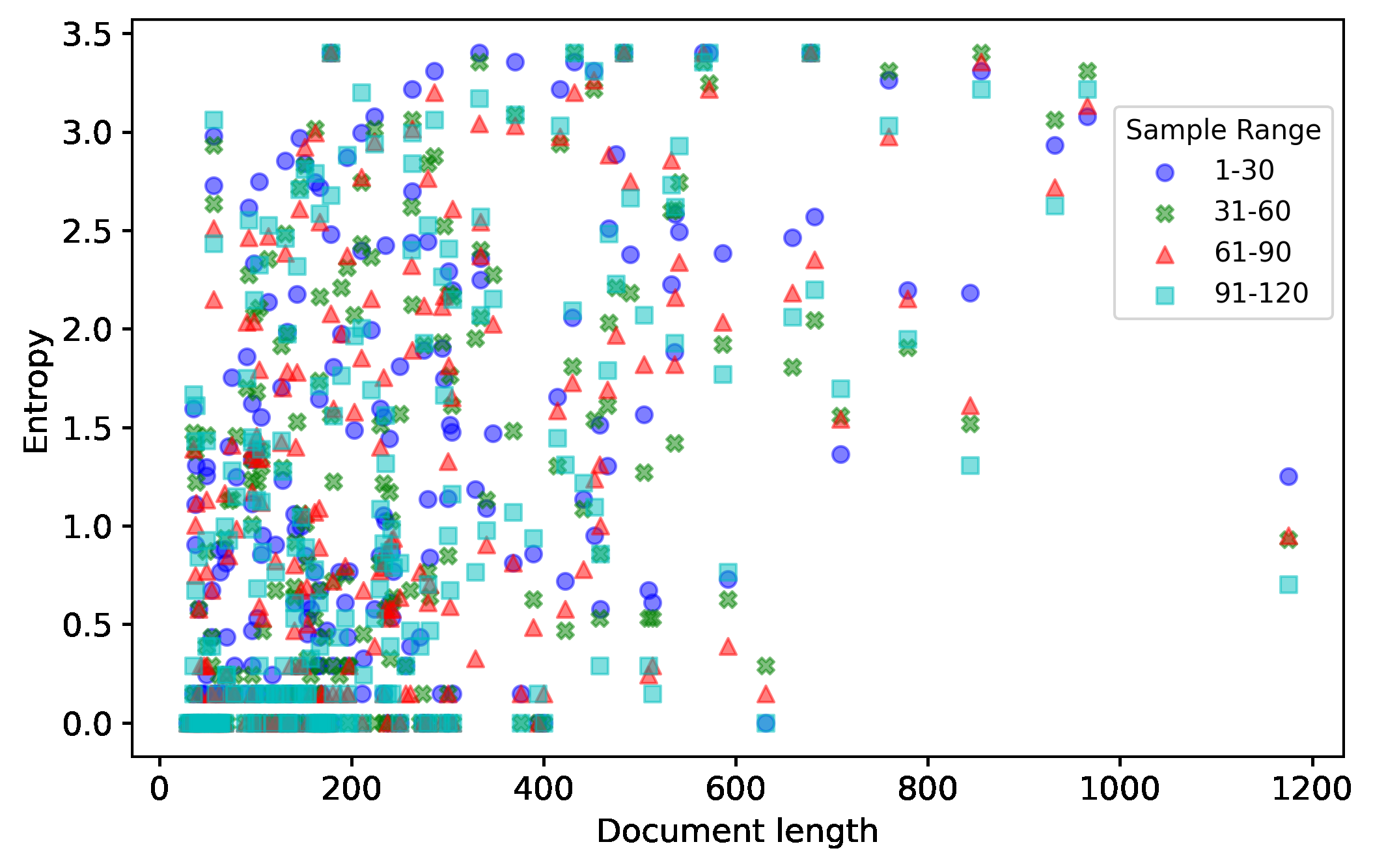