Neural Deepfake Detection with Factual Structure of Text
Wanjun Zhong, Duyu Tang, Zenan Xu, Ruize Wang, Nan Duan, Ming Zhou, Jiahai Wang, Jian Yin
Semantics: Sentence-level Semantics, Textual Inference and Other areas Long Paper
You can open the pre-recorded video in a separate window.
Abstract:
Deepfake detection, the task of automatically discriminating machine-generated text, is increasingly critical with recent advances in natural language generative models. Existing approaches to deepfake detection typically represent documents with coarse-grained representations. However, they struggle to capture factual structures of documents, which is a discriminative factor between machine-generated and human-written text according to our statistical analysis. To address this, we propose a graph-based model that utilizes the factual structure of a document for deepfake detection of text. Our approach represents the factual structure of a given document as an entity graph, which is further utilized to learn sentence representations with a graph neural network. Sentence representations are then composed to a document representation for making predictions, where consistent relations between neighboring sentences are sequentially modeled. Results of experiments on two public deepfake datasets show that our approach significantly improves strong base models built with RoBERTa. Model analysis further indicates that our model can distinguish the difference in the factual structure between machine-generated text and human-written text.
NOTE: Video may display a random order of authors.
Correct author list is at the top of this page.
Connected Papers in EMNLP2020
Similar Papers
SLM: Learning a Discourse Language Representation with Sentence Unshuffling
Haejun Lee, Drew A. Hudson, Kangwook Lee, Christopher D. Manning,
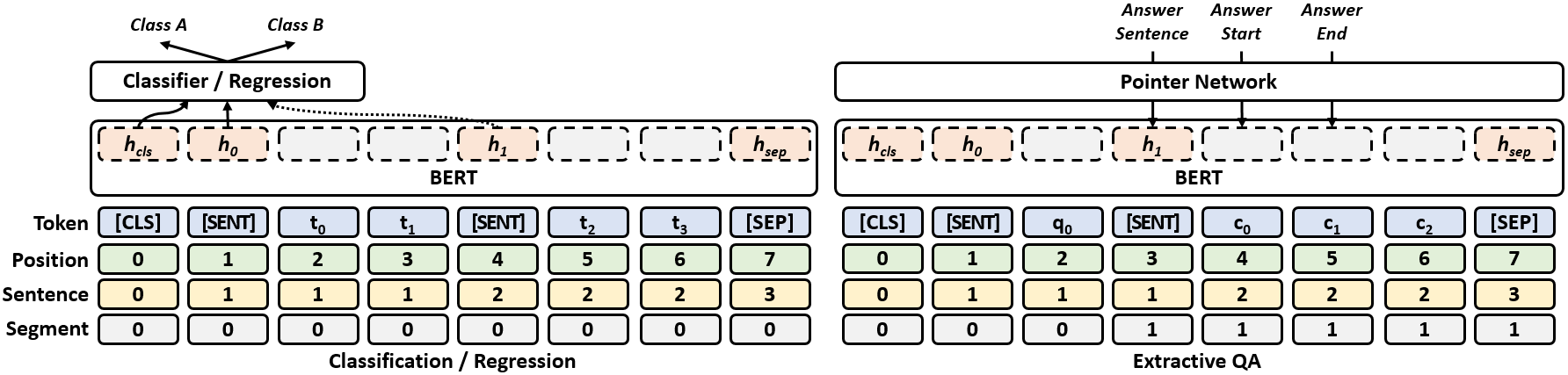
Learning from Context or Names? An Empirical Study on Neural Relation Extraction
Hao Peng, Tianyu Gao, Xu Han, Yankai Lin, Peng Li, Zhiyuan Liu, Maosong Sun, Jie Zhou,
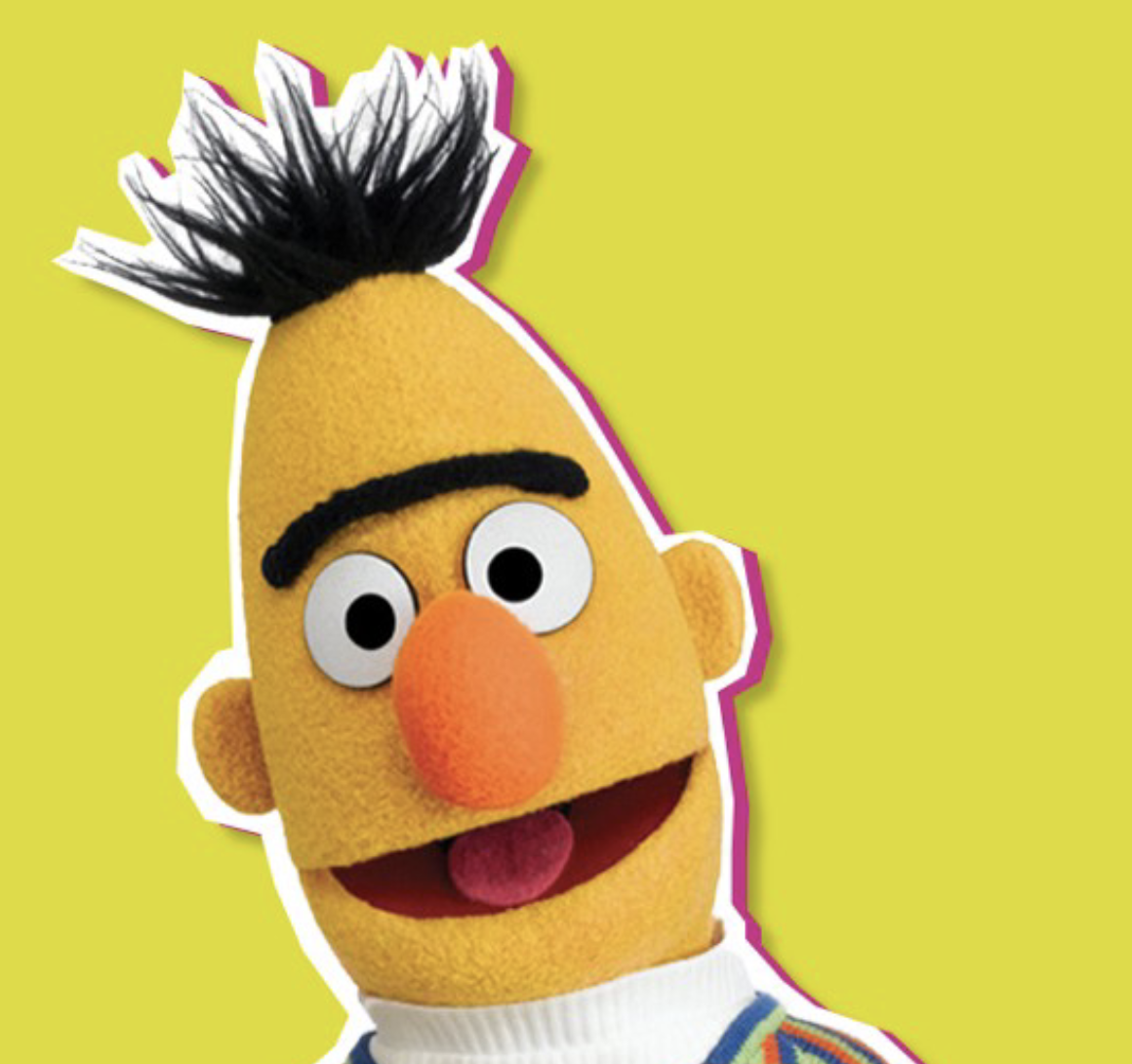