PatchBERT: Just-in-Time, Out-of-Vocabulary Patching
Sangwhan Moon, Naoaki Okazaki
Machine Learning for NLP Short Paper
You can open the pre-recorded video in a separate window.
Abstract:
Large scale pre-trained language models have shown groundbreaking performance improvements for transfer learning in the domain of natural language processing. In our paper, we study a pre-trained multilingual BERT model and analyze the OOV rate on downstream tasks, how it introduces information loss, and as a side-effect, obstructs the potential of the underlying model. We then propose multiple approaches for mitigation and demonstrate that it improves performance with the same parameter count when combined with fine-tuning.
NOTE: Video may display a random order of authors.
Correct author list is at the top of this page.
Connected Papers in EMNLP2020
Similar Papers
Cross-Thought for Sentence Encoder Pre-training
Shuohang Wang, Yuwei Fang, Siqi Sun, Zhe Gan, Yu Cheng, Jingjing Liu, Jing Jiang,
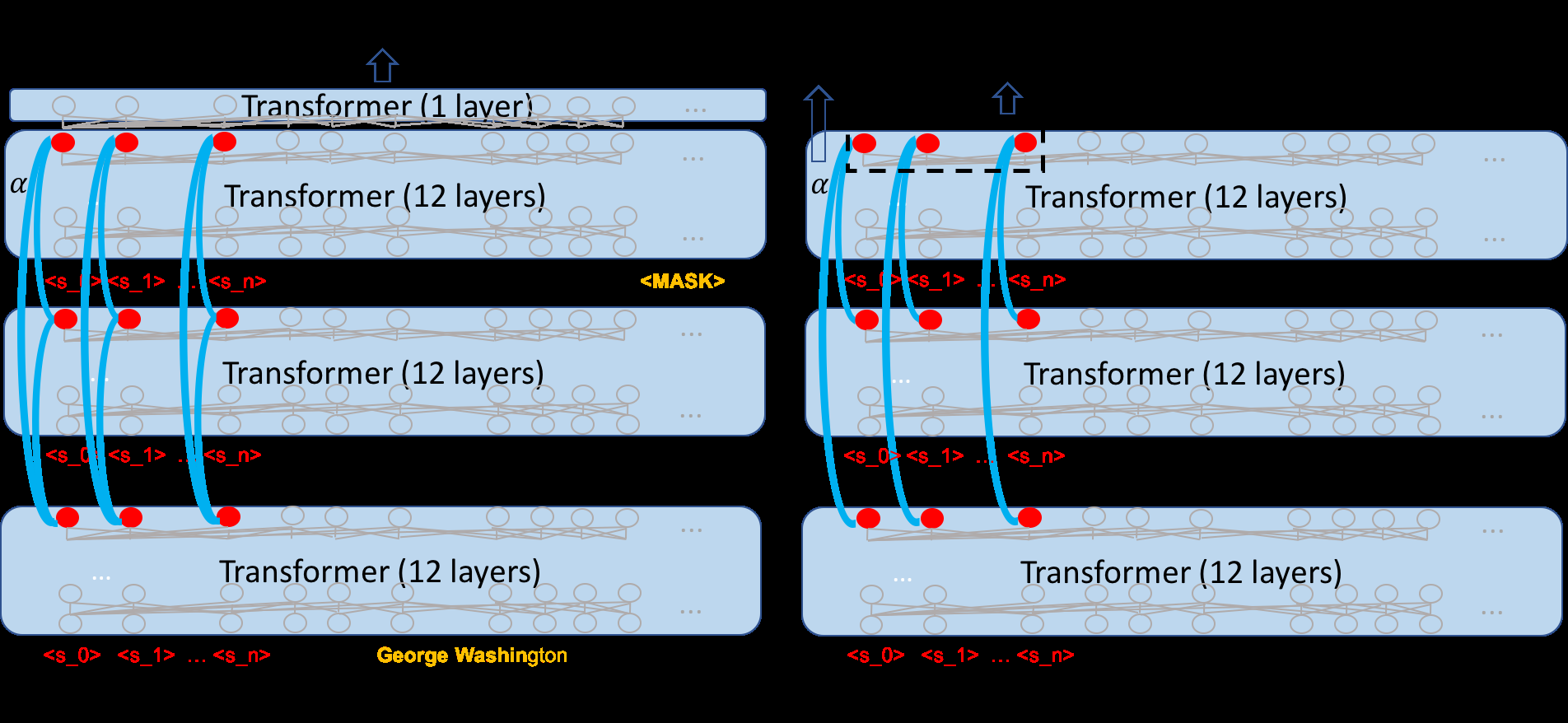
On the Sparsity of Neural Machine Translation Models
Yong Wang, Longyue Wang, Victor Li, Zhaopeng Tu,

On the importance of pre-training data volume for compact language models
Vincent Micheli, Martin d'Hoffschmidt, François Fleuret,

Don't Use English Dev: On the Zero-Shot Cross-Lingual Evaluation of Contextual Embeddings
Phillip Keung, Yichao Lu, Julian Salazar, Vikas Bhardwaj,
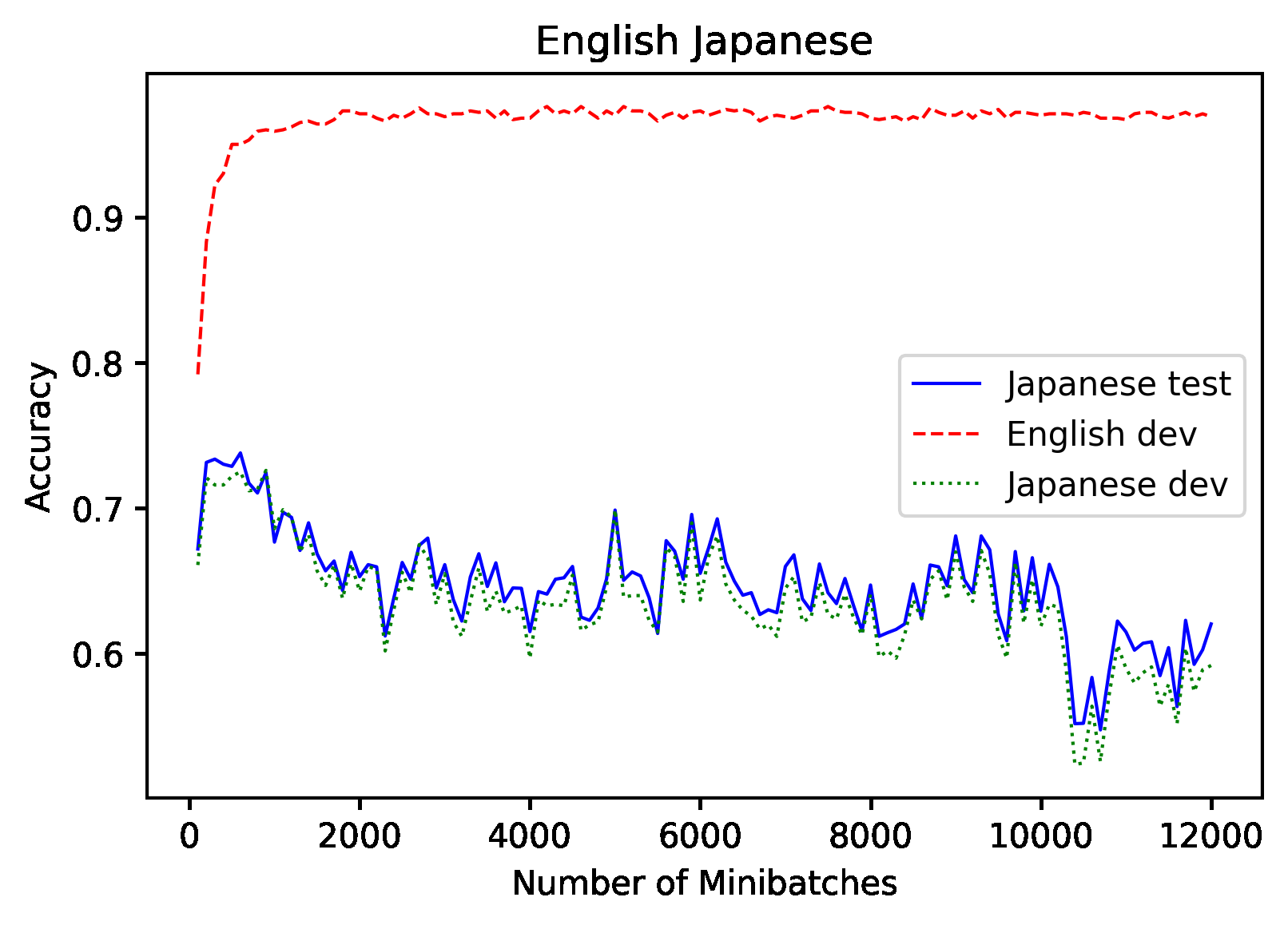