Don't Use English Dev: On the Zero-Shot Cross-Lingual Evaluation of Contextual Embeddings
Phillip Keung, Yichao Lu, Julian Salazar, Vikas Bhardwaj
Machine Translation and Multilinguality Short Paper
You can open the pre-recorded video in a separate window.
Abstract:
Multilingual contextual embeddings have demonstrated state-of-the-art performance in zero-shot cross-lingual transfer learning, where multilingual BERT is fine-tuned on one source language and evaluated on a different target language. However, published results for mBERT zero-shot accuracy vary as much as 17 points on the MLDoc classification task across four papers. We show that the standard practice of using English dev accuracy for model selection in the zero-shot setting makes it difficult to obtain reproducible results on the MLDoc and XNLI tasks. English dev accuracy is often uncorrelated (or even anti-correlated) with target language accuracy, and zero-shot performance varies greatly at different points in the same fine-tuning run and between different fine-tuning runs. These reproducibility issues are also present for other tasks with different pre-trained embeddings (e.g., MLQA with XLM-R). We recommend providing oracle scores alongside zero-shot results: still fine-tune using English data, but choose a checkpoint with the target dev set. Reporting this upper bound makes results more consistent by avoiding arbitrarily bad checkpoints.
NOTE: Video may display a random order of authors.
Correct author list is at the top of this page.
Connected Papers in EMNLP2020
Similar Papers
From Zero to Hero: On the Limitations of Zero-Shot Language Transfer with Multilingual Transformers
Anne Lauscher, Vinit Ravishankar, Ivan Vulić, Goran Glavaš,
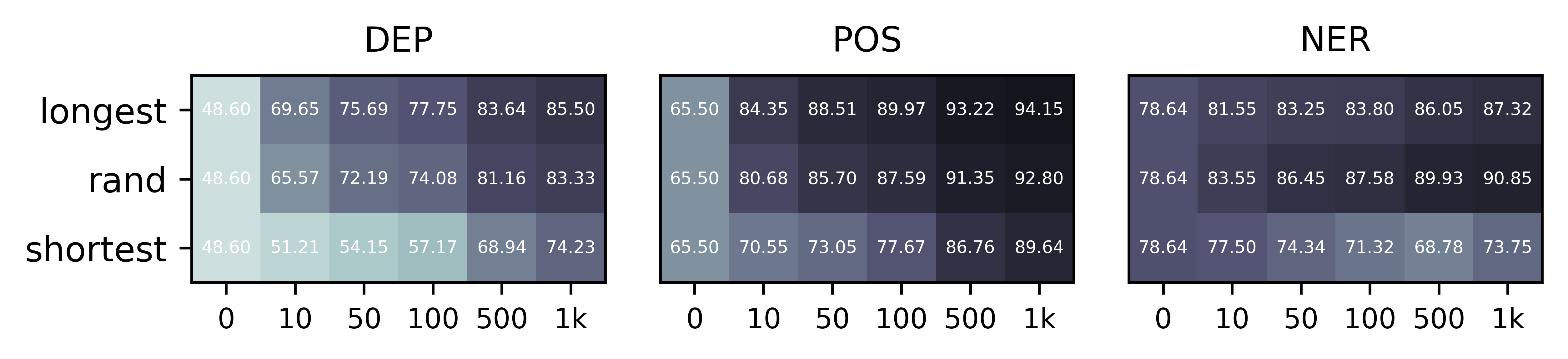
MAD-X: An Adapter-Based Framework for Multi-Task Cross-Lingual Transfer
Jonas Pfeiffer, Ivan Vulić, Iryna Gurevych, Sebastian Ruder,

MultiCQA: Zero-Shot Transfer of Self-Supervised Text Matching Models on a Massive Scale
Andreas Rücklé, Jonas Pfeiffer, Iryna Gurevych,

Cross-lingual Spoken Language Understanding with Regularized Representation Alignment
Zihan Liu, Genta Indra Winata, Peng Xu, Zhaojiang Lin, Pascale Fung,
