TOD-BERT: Pre-trained Natural Language Understanding for Task-Oriented Dialogue
Chien-Sheng Wu, Steven C.H. Hoi, Richard Socher, Caiming Xiong
Dialog and Interactive Systems Long Paper
You can open the pre-recorded video in a separate window.
Abstract:
The underlying difference of linguistic patterns between general text and task-oriented dialogue makes existing pre-trained language models less useful in practice. In this work, we unify nine human-human and multi-turn task-oriented dialogue datasets for language modeling. To better model dialogue behavior during pre-training, we incorporate user and system tokens into the masked language modeling. We propose a contrastive objective function to simulate the response selection task. Our pre-trained task-oriented dialogue BERT (TOD-BERT) outperforms strong baselines like BERT on four downstream task-oriented dialogue applications, including intention recognition, dialogue state tracking, dialogue act prediction, and response selection. We also show that TOD-BERT has a stronger few-shot ability that can mitigate the data scarcity problem for task-oriented dialogue.
NOTE: Video may display a random order of authors.
Correct author list is at the top of this page.
Connected Papers in EMNLP2020
Similar Papers
Multi-turn Response Selection using Dialogue Dependency Relations
Qi Jia, Yizhu Liu, Siyu Ren, Kenny Zhu, Haifeng Tang,

MinTL: Minimalist Transfer Learning for Task-Oriented Dialogue Systems
Zhaojiang Lin, Andrea Madotto, Genta Indra Winata, Pascale Fung,
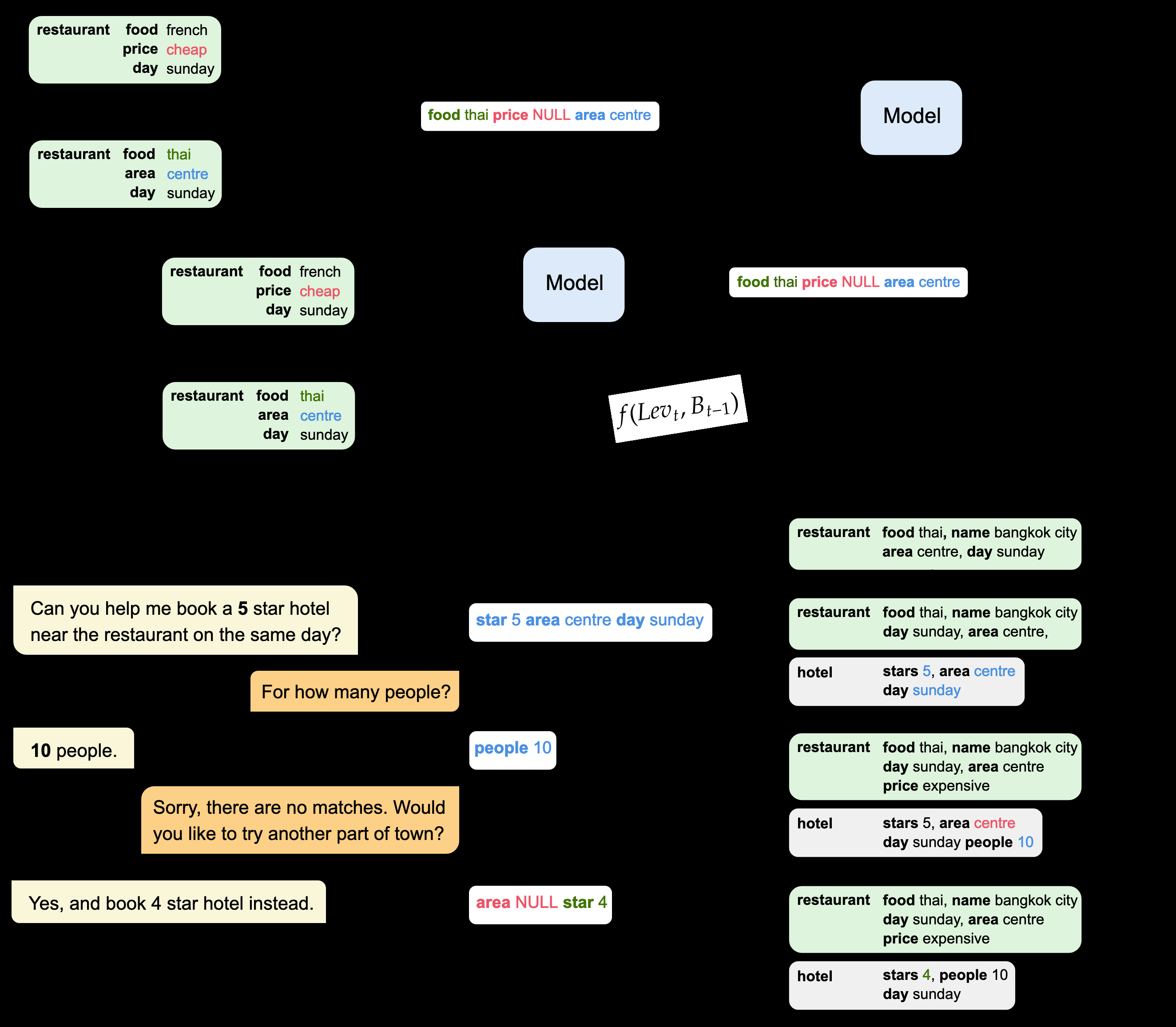
Structured Attention for Unsupervised Dialogue Structure Induction
Liang Qiu, Yizhou Zhao, Weiyan Shi, Yuan Liang, Feng Shi, Tao Yuan, Zhou Yu, Song-Chun Zhu,
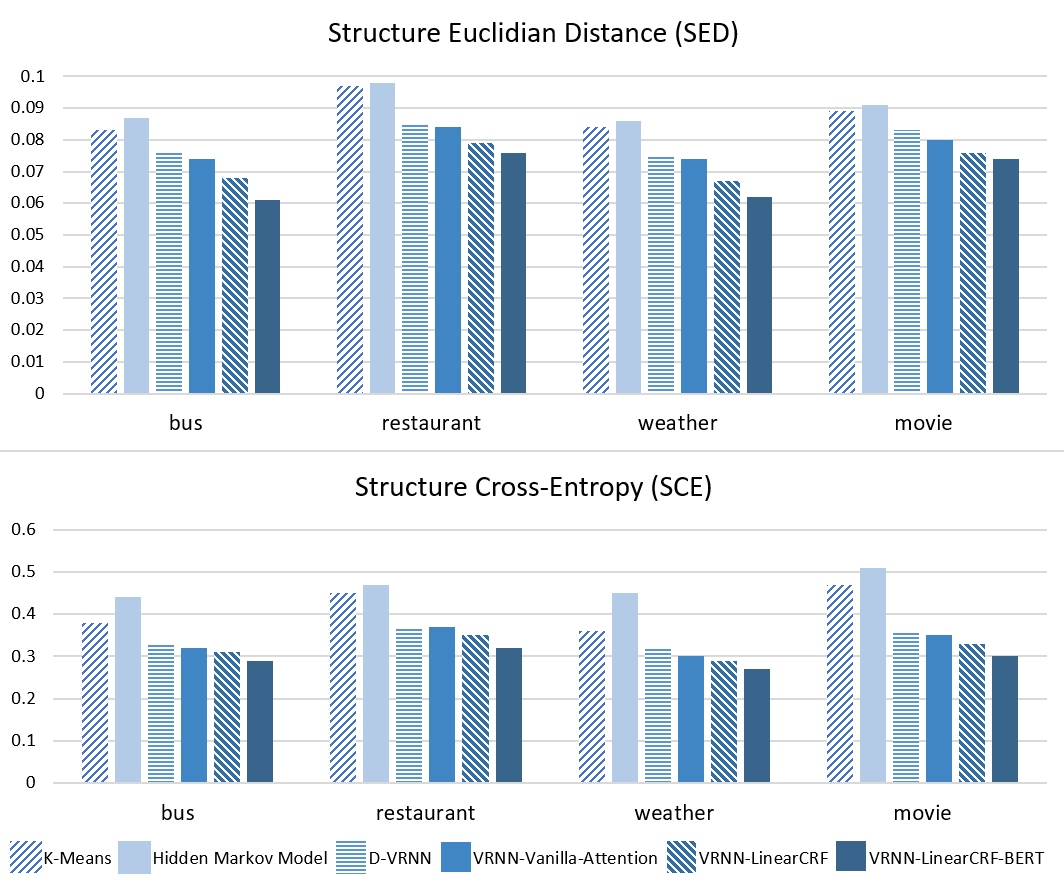
Simple Data Augmentation with the Mask Token Improves Domain Adaptation for Dialog Act Tagging
Semih Yavuz, Kazuma Hashimoto, Wenhao Liu, Nitish Shirish Keskar, Richard Socher, Caiming Xiong,
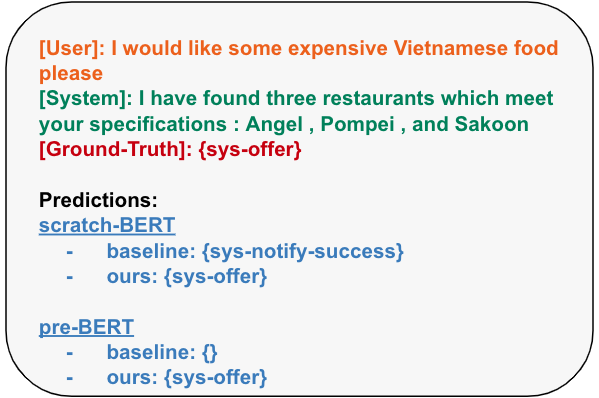