Simple Data Augmentation with the Mask Token Improves Domain Adaptation for Dialog Act Tagging
Semih Yavuz, Kazuma Hashimoto, Wenhao Liu, Nitish Shirish Keskar, Richard Socher, Caiming Xiong
Dialog and Interactive Systems Short Paper
You can open the pre-recorded video in a separate window.
Abstract:
The concept of Dialogue Act (DA) is universal across different task-oriented dialogue domains - the act of ``request" carries the same speaker intention whether it is for restaurant reservation or flight booking. However, DA taggers trained on one domain do not generalize well to other domains, which leaves us with the expensive need for a large amount of annotated data in the target domain. In this work, we investigate how to better adapt DA taggers to desired target domains with only unlabeled data. We propose MaskAugment, a controllable mechanism that augments text input by leveraging the pre-trained Mask token from BERT model. Inspired by consistency regularization, we use MaskAugment to introduce an unsupervised teacher-student learning scheme to examine the domain adaptation of DA taggers. Our extensive experiments on the Simulated Dialogue (GSim) and Schema-Guided Dialogue (SGD) datasets show that MaskAugment is useful in improving the cross-domain generalization for DA tagging.
NOTE: Video may display a random order of authors.
Correct author list is at the top of this page.
Connected Papers in EMNLP2020
Similar Papers
Dialogue Distillation: Open-Domain Dialogue Augmentation Using Unpaired Data
Rongsheng Zhang, Yinhe Zheng, Jianzhi Shao, Xiaoxi Mao, Yadong Xi, Minlie Huang,
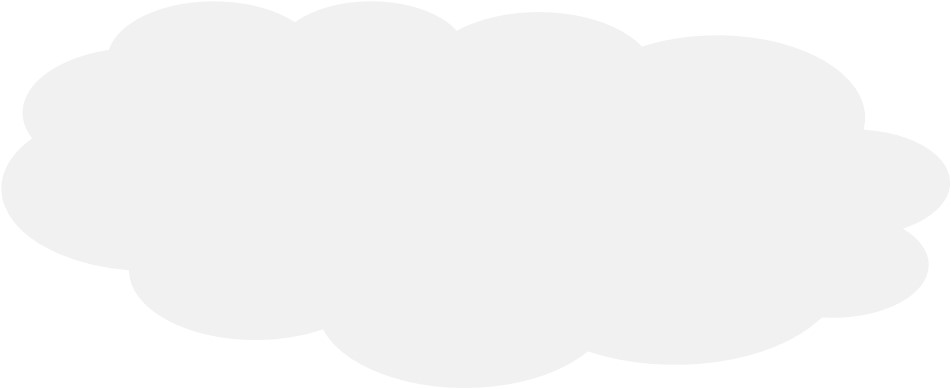
Structured Attention for Unsupervised Dialogue Structure Induction
Liang Qiu, Yizhou Zhao, Weiyan Shi, Yuan Liang, Feng Shi, Tao Yuan, Zhou Yu, Song-Chun Zhu,
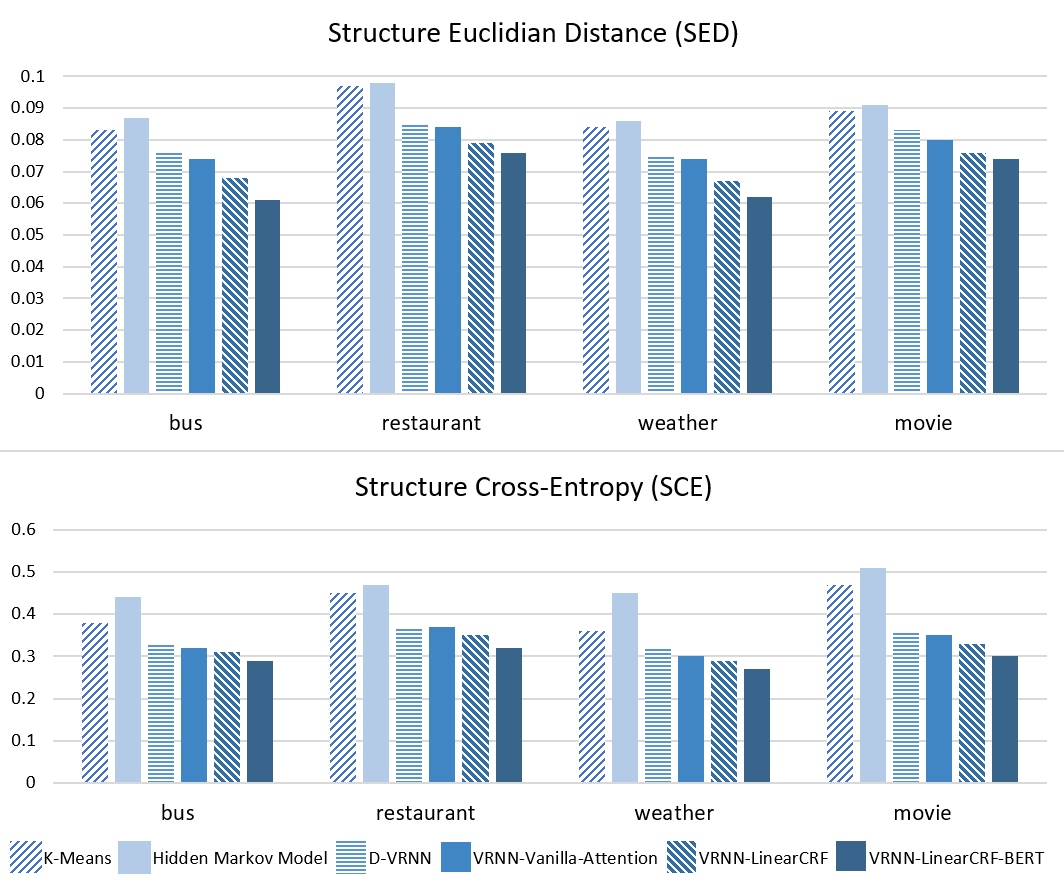
MinTL: Minimalist Transfer Learning for Task-Oriented Dialogue Systems
Zhaojiang Lin, Andrea Madotto, Genta Indra Winata, Pascale Fung,
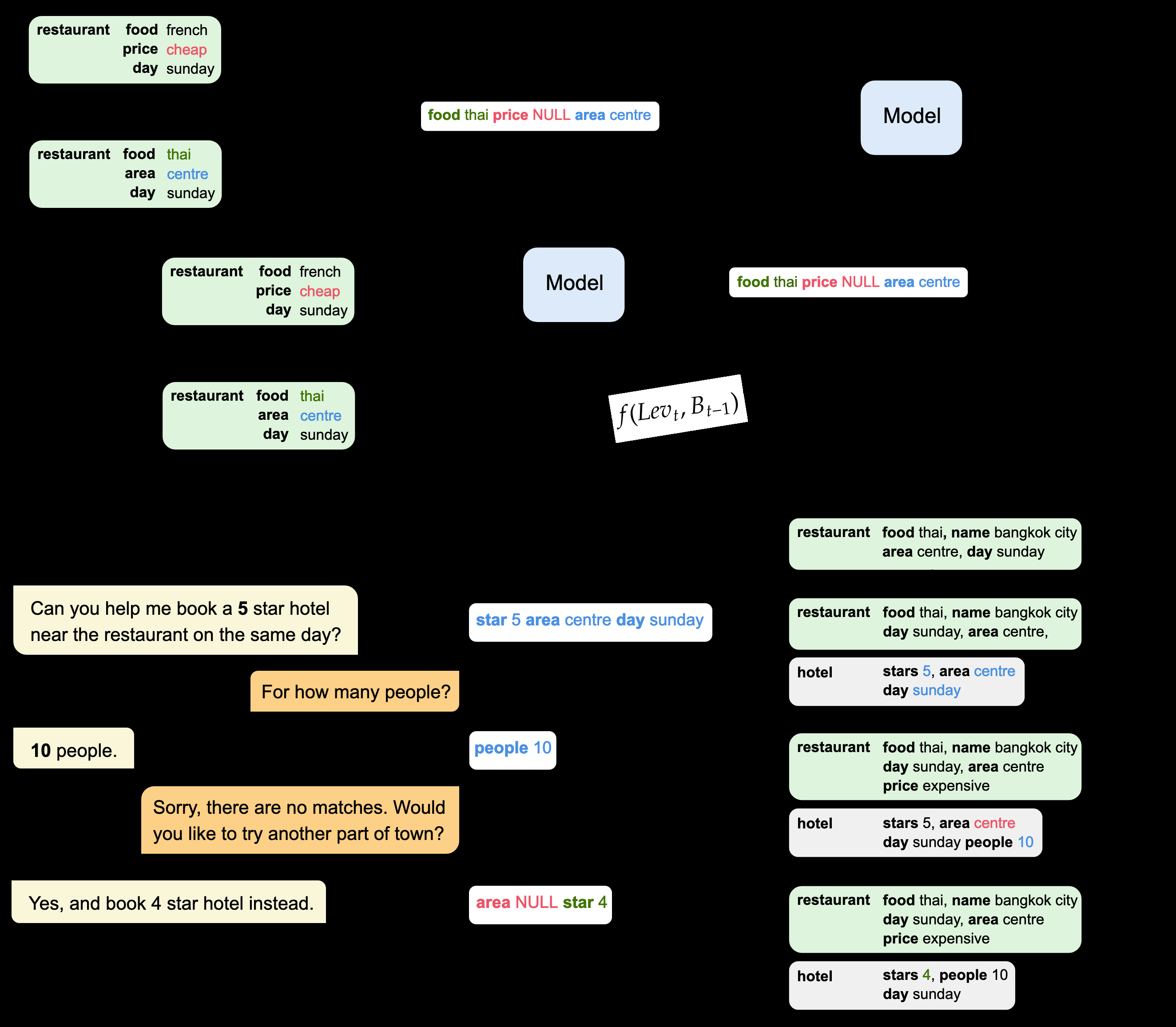
RiSAWOZ: A Large-Scale Multi-Domain Wizard-of-Oz Dataset with Rich Semantic Annotations for Task-Oriented Dialogue Modeling
Jun Quan, Shian Zhang, Qian Cao, Zizhong Li, Deyi Xiong,
