MinTL: Minimalist Transfer Learning for Task-Oriented Dialogue Systems
Zhaojiang Lin, Andrea Madotto, Genta Indra Winata, Pascale Fung
Dialog and Interactive Systems Long Paper
You can open the pre-recorded video in a separate window.
Abstract:
In this paper, we propose Minimalist Transfer Learning (MinTL) to simplify the system design process of task-oriented dialogue systems and alleviate the over-dependency on annotated data. MinTL is a simple yet effective transfer learning framework, which allows us to plug-and-play pre-trained seq2seq models, and jointly learn dialogue state tracking and dialogue response generation. Unlike previous approaches, which use a copy mechanism to "carryover'' the old dialogue states to the new one, we introduce Levenshtein belief spans (Lev), that allows efficient dialogue state tracking with a minimal generation length. We instantiate our learning framework with two pre-trained backbones: T5 and BART, and evaluate them on MultiWOZ. Extensive experiments demonstrate that: 1) our systems establish new state-of-the-art results on end-to-end response generation, 2) MinTL-based systems are more robust than baseline methods in the low resource setting, and they achieve competitive results with only 20\% training data, and 3) Lev greatly improves the inference efficiency.
NOTE: Video may display a random order of authors.
Correct author list is at the top of this page.
Connected Papers in EMNLP2020
Similar Papers
TOD-BERT: Pre-trained Natural Language Understanding for Task-Oriented Dialogue
Chien-Sheng Wu, Steven C.H. Hoi, Richard Socher, Caiming Xiong,
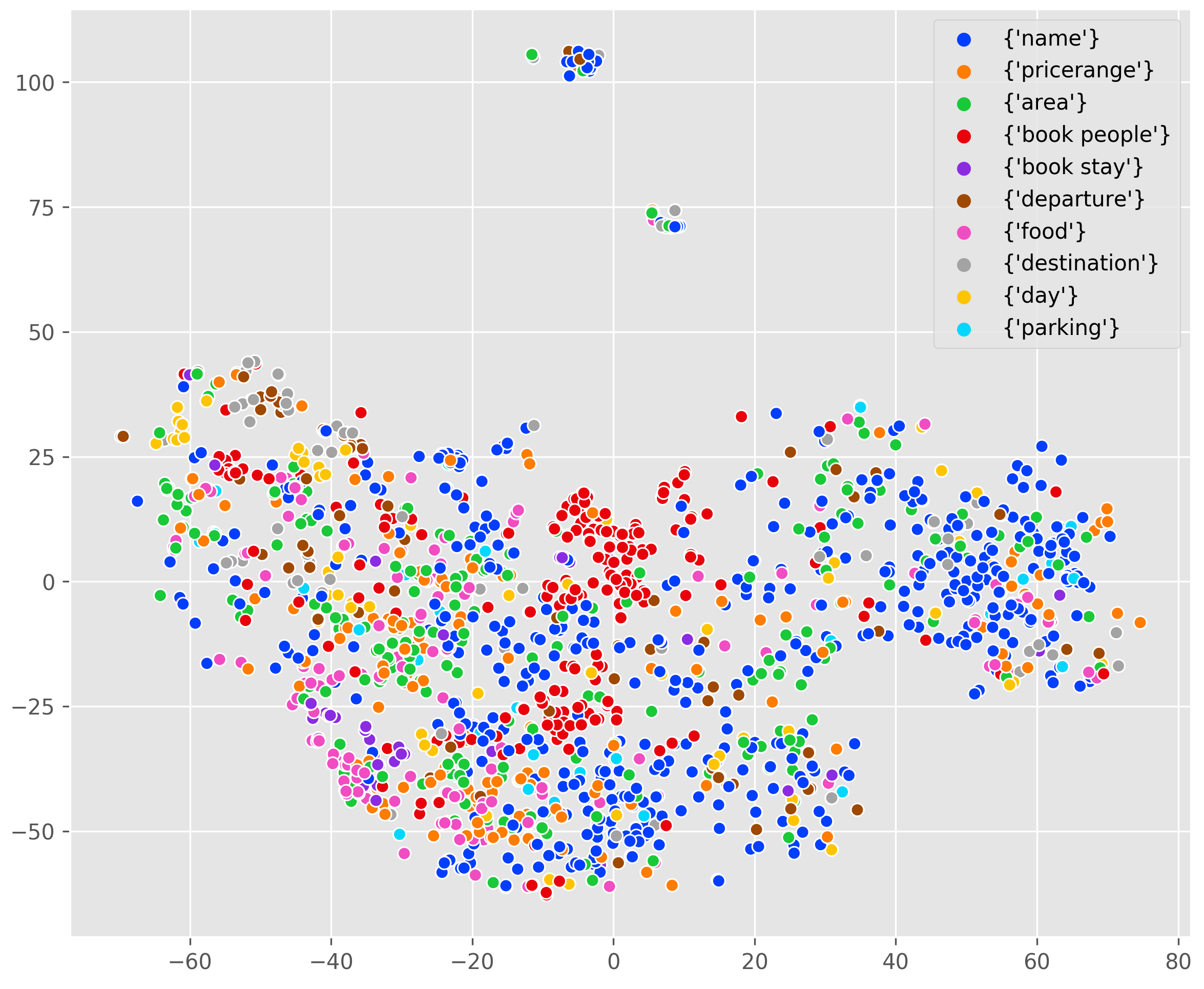
Dialogue Distillation: Open-Domain Dialogue Augmentation Using Unpaired Data
Rongsheng Zhang, Yinhe Zheng, Jianzhi Shao, Xiaoxi Mao, Yadong Xi, Minlie Huang,
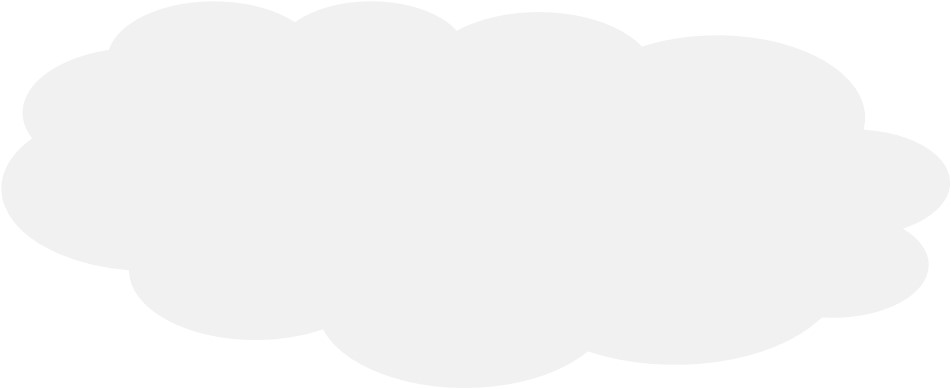
UniConv: A Unified Conversational Neural Architecture for Multi-domain Task-oriented Dialogues
Hung Le, Doyen Sahoo, Chenghao Liu, Nancy Chen, Steven C.H. Hoi,
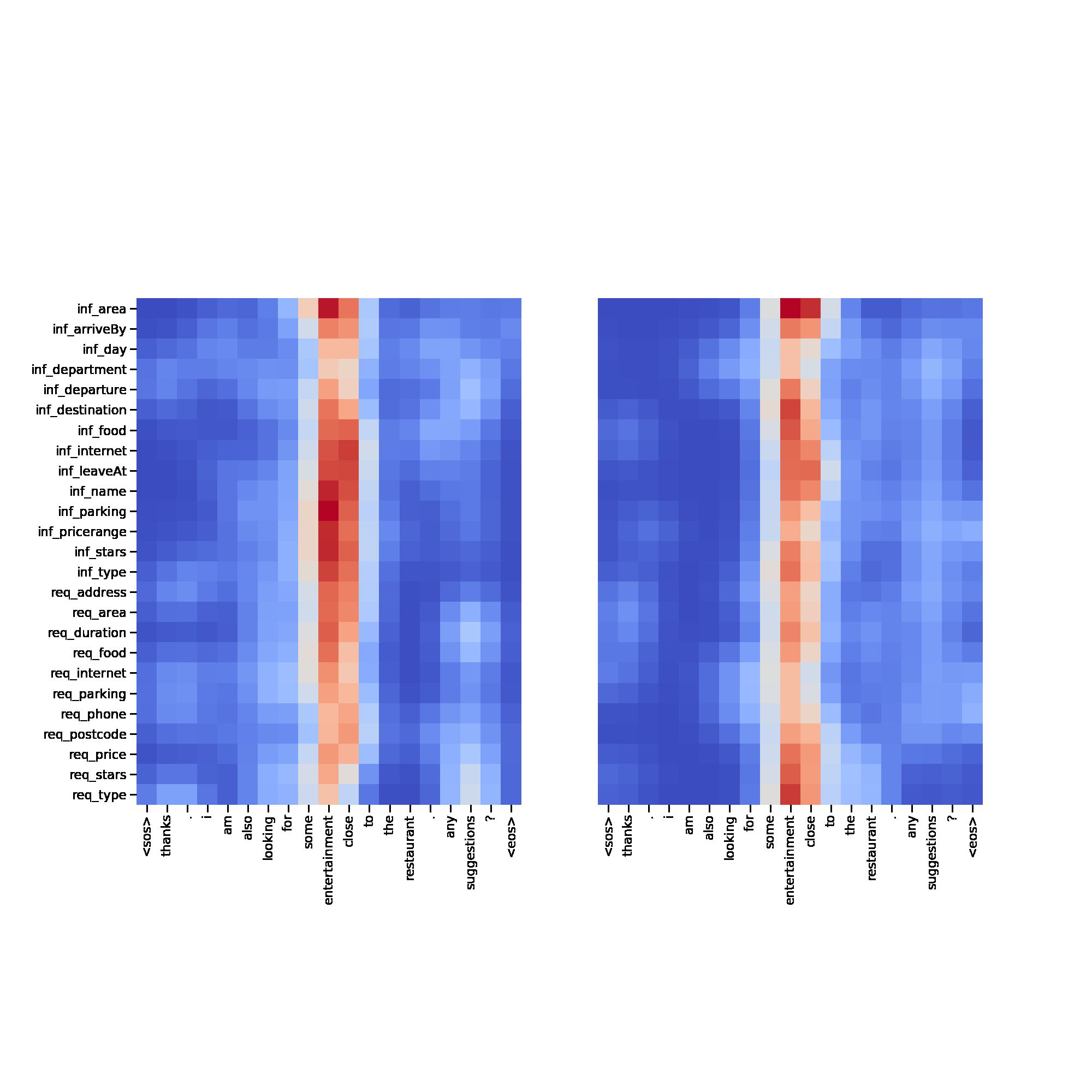
Multi-turn Response Selection using Dialogue Dependency Relations
Qi Jia, Yizhu Liu, Siyu Ren, Kenny Zhu, Haifeng Tang,
