Structured Attention for Unsupervised Dialogue Structure Induction
Liang Qiu, Yizhou Zhao, Weiyan Shi, Yuan Liang, Feng Shi, Tao Yuan, Zhou Yu, Song-Chun Zhu
Dialog and Interactive Systems Long Paper
You can open the pre-recorded video in a separate window.
Abstract:
Inducing a meaningful structural representation from one or a set of dialogues is a crucial but challenging task in computational linguistics. Advancement made in this area is critical for dialogue system design and discourse analysis. It can also be extended to solve grammatical inference. In this work, we propose to incorporate structured attention layers into a Variational Recurrent Neural Network (VRNN) model with discrete latent states to learn dialogue structure in an unsupervised fashion. Compared to a vanilla VRNN, structured attention enables a model to focus on different parts of the source sentence embeddings while enforcing a structural inductive bias. Experiments show that on two-party dialogue datasets, VRNN with structured attention learns semantic structures that are similar to templates used to generate this dialogue corpus. While on multi-party dialogue datasets, our model learns an interactive structure demonstrating its capability of distinguishing speakers or addresses, automatically disentangling dialogues without explicit human annotation.
NOTE: Video may display a random order of authors.
Correct author list is at the top of this page.
Connected Papers in EMNLP2020
Similar Papers
Generating Dialogue Responses from a Semantic Latent Space
Wei-Jen Ko, Avik Ray, Yilin Shen, Hongxia Jin,
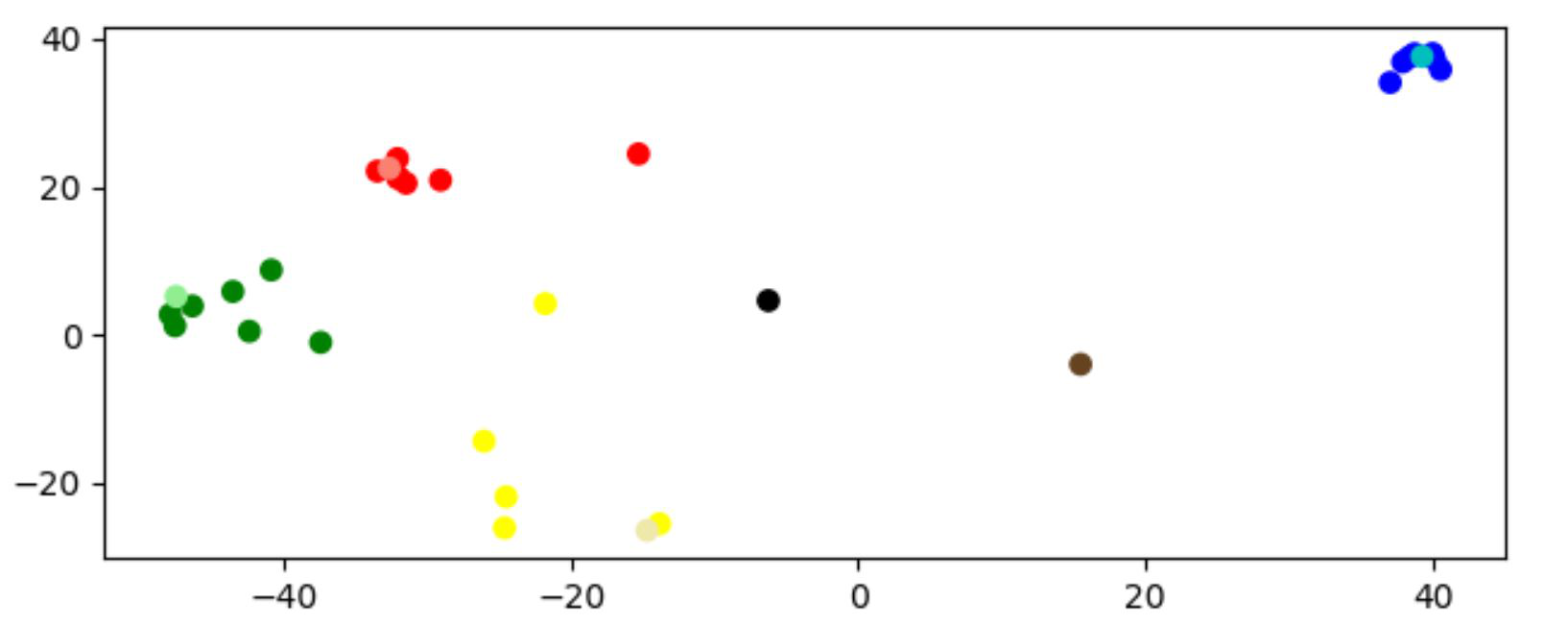
TOD-BERT: Pre-trained Natural Language Understanding for Task-Oriented Dialogue
Chien-Sheng Wu, Steven C.H. Hoi, Richard Socher, Caiming Xiong,
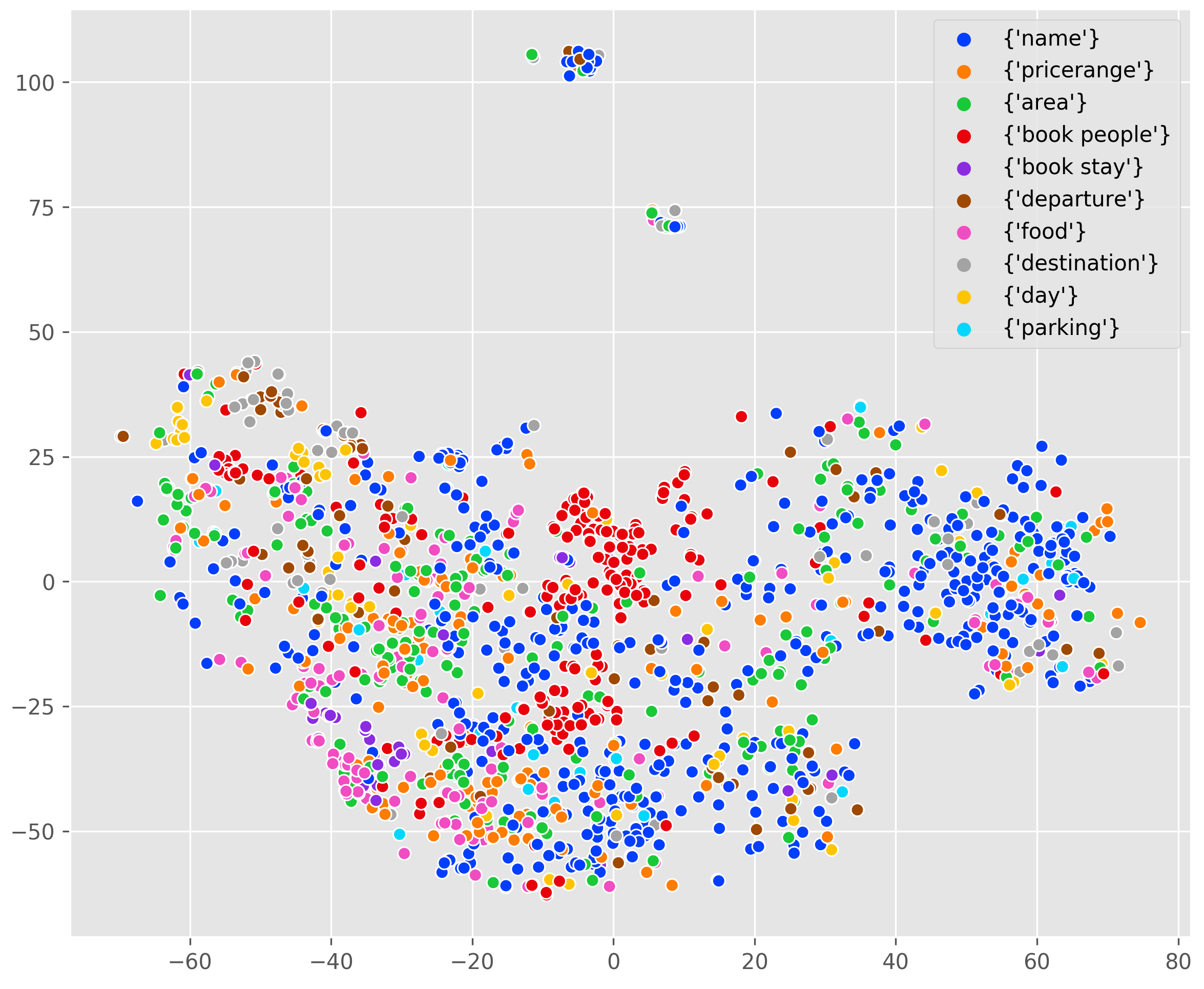
Filtering Noisy Dialogue Corpora by Connectivity and Content Relatedness
Reina Akama, Sho Yokoi, Jun Suzuki, Kentaro Inui,
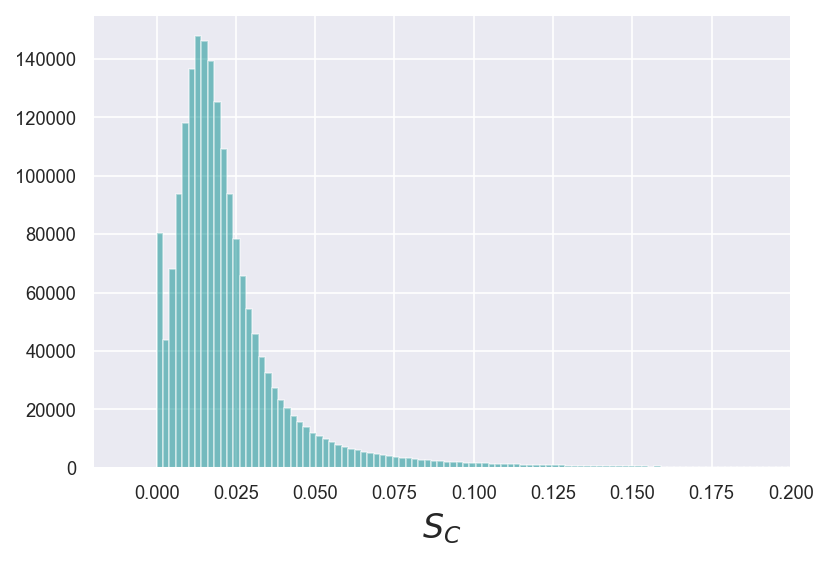
Multi-turn Response Selection using Dialogue Dependency Relations
Qi Jia, Yizhu Liu, Siyu Ren, Kenny Zhu, Haifeng Tang,
