Easy, Reproducible and Quality-Controlled Data Collection with CROWDAQ
Qiang Ning, Hao Wu, Pradeep Dasigi, Dheeru Dua, Matt Gardner, Robert L Logan IV, Ana Marasović, Zhen Nie
Demo Paper
Abstract:
High-quality and large-scale data are key to success for AI systems. However, large-scale data annotation efforts are often confronted with a set of common challenges: (1) designing a user-friendly annotation interface; (2) training enough annotators efficiently; and (3) reproducibility. To address these problems, we introduce CROWDAQ, an open-source platform that standardizes the data collection pipeline with customizable user-interface components, automated annotator qualification, and saved pipelines in a re-usable format. We show that CROWDAQ simplifies data annotation significantly on a diverse set of data collection use cases and we hope it will be a convenient tool for the community.
Similar Papers
New Protocols and Negative Results for Textual Entailment Data Collection
Samuel R. Bowman, Jennimaria Palomaki, Livio Baldini Soares, Emily Pitler,

Iterative Feature Mining for Constraint-Based Data Collection to Increase Data Diversity and Model Robustness
Stefan Larson, Anthony Zheng, Anish Mahendran, Rishi Tekriwal, Adrian Cheung, Eric Guldan, Kevin Leach, Jonathan K. Kummerfeld,

Small but Mighty: New Benchmarks for Split and Rephrase
Li Zhang, Huaiyu Zhu, Siddhartha Brahma, Yunyao Li,
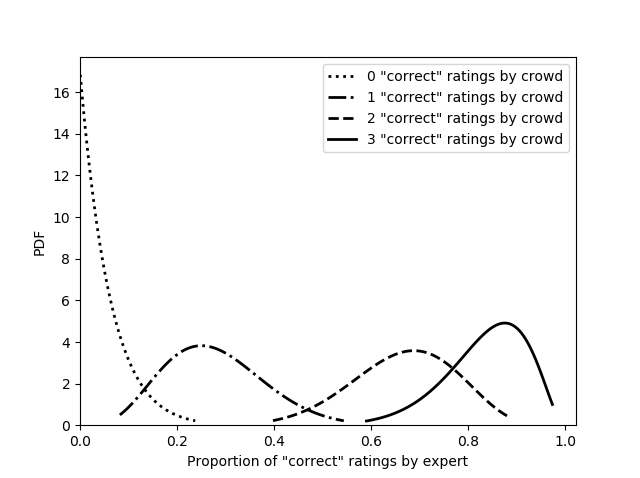