New Protocols and Negative Results for Textual Entailment Data Collection
Samuel R. Bowman, Jennimaria Palomaki, Livio Baldini Soares, Emily Pitler
Semantics: Sentence-level Semantics, Textual Inference and Other areas Long Paper
You can open the pre-recorded video in a separate window.
Abstract:
Natural language inference (NLI) data has proven useful in benchmarking and, especially, as pretraining data for tasks requiring language understanding. However, the crowdsourcing protocol that was used to collect this data has known issues and was not explicitly optimized for either of these purposes, so it is likely far from ideal. We propose four alternative protocols, each aimed at improving either the ease with which annotators can produce sound training examples or the quality and diversity of those examples. Using these alternatives and a fifth baseline protocol, we collect and compare five new 8.5k-example training sets. In evaluations focused on transfer learning applications, our results are solidly negative, with models trained on our baseline dataset yielding good transfer performance to downstream tasks, but none of our four new methods (nor the recent ANLI) showing any improvements over that baseline. In a small silver lining, we observe that all four new protocols, especially those where annotators edit *pre-filled* text boxes, reduce previously observed issues with annotation artifacts.
NOTE: Video may display a random order of authors.
Correct author list is at the top of this page.
Connected Papers in EMNLP2020
Similar Papers
Exposing Shallow Heuristics of Relation Extraction Models with Challenge Data
Shachar Rosenman, Alon Jacovi, Yoav Goldberg,

More Bang for Your Buck: Natural Perturbation for Robust Question Answering
Daniel Khashabi, Tushar Khot, Ashish Sabharwal,

What Can We Learn from Collective Human Opinions on Natural Language Inference Data?
Yixin Nie, Xiang Zhou, Mohit Bansal,
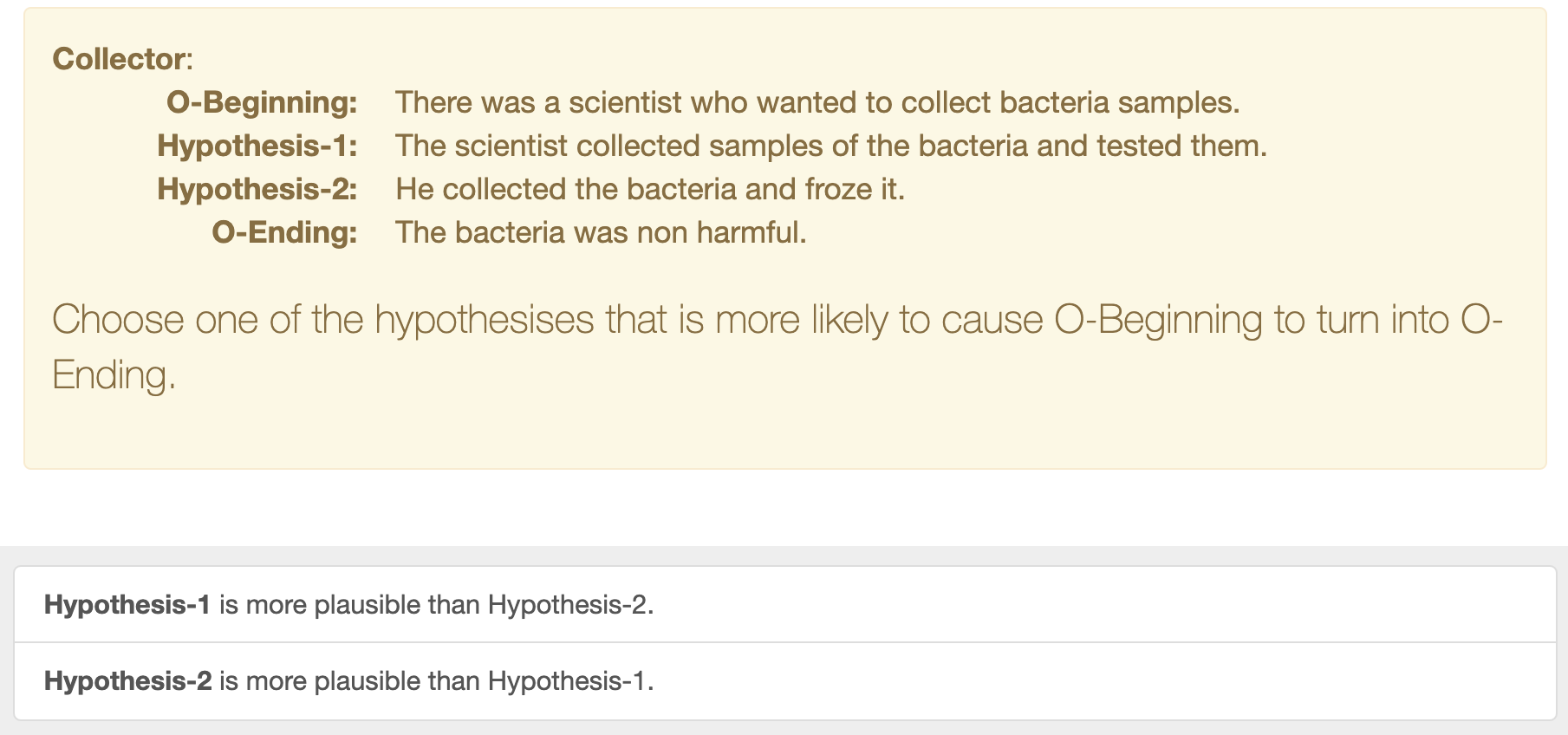