Iterative Feature Mining for Constraint-Based Data Collection to Increase Data Diversity and Model Robustness
Stefan Larson, Anthony Zheng, Anish Mahendran, Rishi Tekriwal, Adrian Cheung, Eric Guldan, Kevin Leach, Jonathan K. Kummerfeld
Dialog and Interactive Systems Short Paper
You can open the pre-recorded video in a separate window.
Abstract:
Diverse data is crucial for training robust models, but crowdsourced text often lacks diversity as workers tend to write simple variations from prompts. We propose a general approach for guiding workers to write more diverse text by iteratively constraining their writing. We show how prior workflows are special cases of our approach, and present a way to apply the approach to dialog tasks such as intent classification and slot-filling. Using our method, we create more challenging versions of test sets from prior dialog datasets and find dramatic performance drops for standard models. Finally, we show that our approach is complementary to recent work on improving data diversity, and training on data collected with our approach leads to more robust models.
NOTE: Video may display a random order of authors.
Correct author list is at the top of this page.
Connected Papers in EMNLP2020
Similar Papers
Cold-start Active Learning through Self-supervised Language Modeling
Michelle Yuan, Hsuan-Tien Lin, Jordan Boyd-Graber,

Textual Data Augmentation for Efficient Active Learning on Tiny Datasets
Husam Quteineh, Spyridon Samothrakis, Richard Sutcliffe,
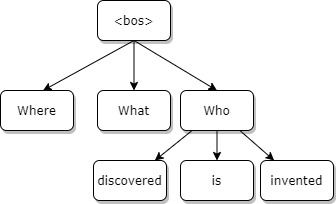
Exposing Shallow Heuristics of Relation Extraction Models with Challenge Data
Shachar Rosenman, Alon Jacovi, Yoav Goldberg,

DAGA: Data Augmentation with a Generation Approach forLow-resource Tagging Tasks
Bosheng Ding, Linlin Liu, Lidong Bing, Canasai Kruengkrai, Thien Hai Nguyen, Shafiq Joty, Luo Si, Chunyan Miao,
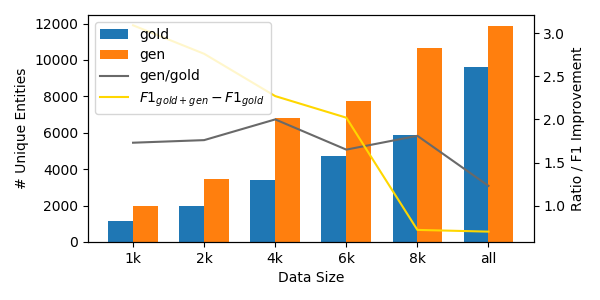