F1 is Not Enough! Models and Evaluation Towards User-Centered Explainable Question Answering
Hendrik Schuff, Heike Adel, Ngoc Thang Vu
Interpretability and Analysis of Models for NLP Long Paper
You can open the pre-recorded video in a separate window.
Abstract:
Explainable question answering systems predict an answer together with an explanation showing why the answer has been selected. The goal is to enable users to assess the correctness of the system and understand its reasoning process. However, we show that current models and evaluation settings have shortcomings regarding the coupling of answer and explanation which might cause serious issues in user experience. As a remedy, we propose a hierarchical model and a new regularization term to strengthen the answer-explanation coupling as well as two evaluation scores to quantify the coupling. We conduct experiments on the HOTPOTQA benchmark data set and perform a user study. The user study shows that our models increase the ability of the users to judge the correctness of the system and that scores like F1 are not enough to estimate the usefulness of a model in a practical setting with human users. Our scores are better aligned with user experience, making them promising candidates for model selection.
NOTE: Video may display a random order of authors.
Correct author list is at the top of this page.
Connected Papers in EMNLP2020
Similar Papers
Context-Aware Answer Extraction in Question Answering
Yeon Seonwoo, Ji-Hoon Kim, Jung-Woo Ha, Alice Oh,
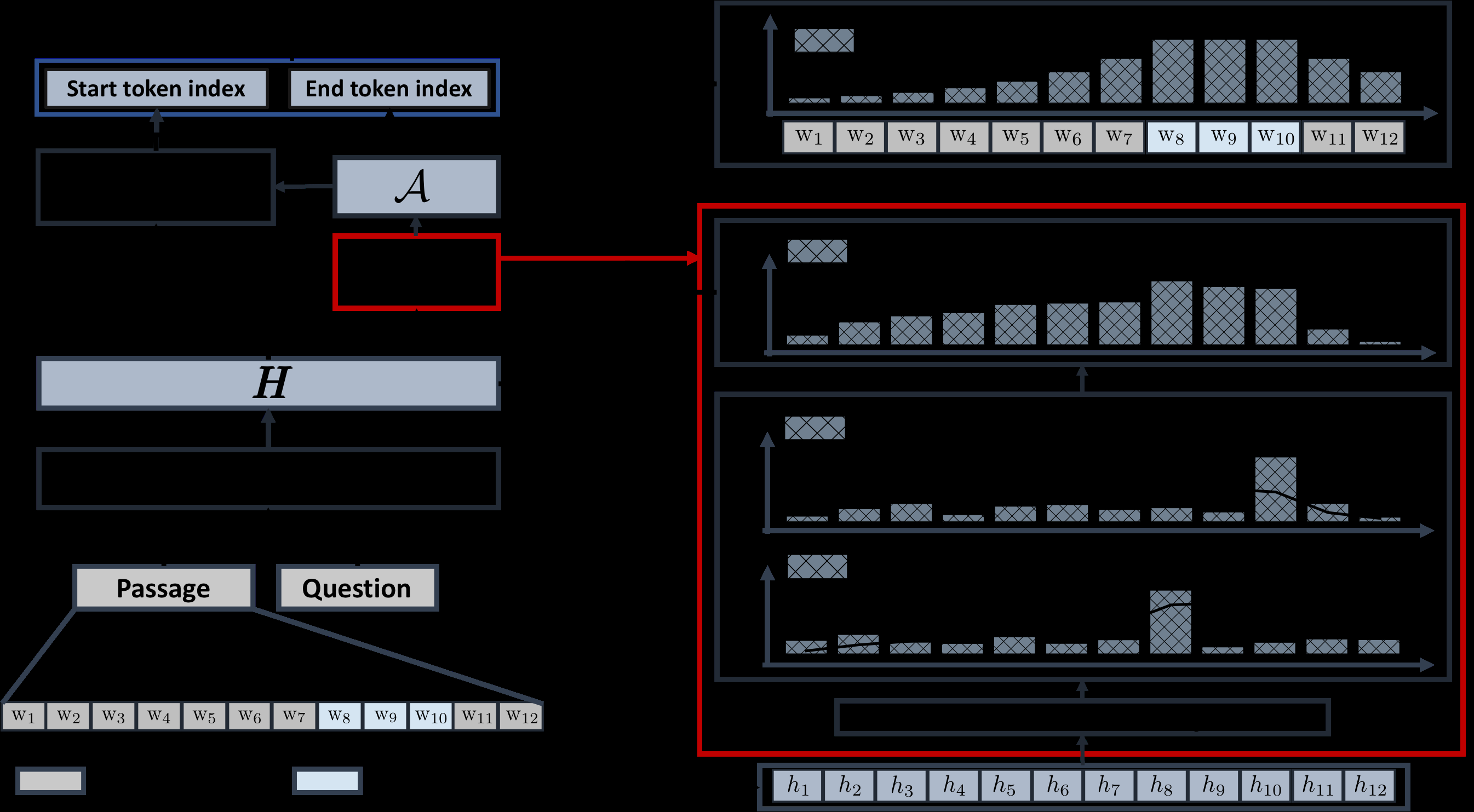
What Does My QA Model Know? Devising Controlled Probes using Expert
Kyle Richardson, Ashish Sabharwal,

ProtoQA: A Question Answering Dataset for Prototypical Common-Sense Reasoning
Michael Boratko, Xiang Li, Tim O'Gorman, Rajarshi Das, Dan Le, Andrew McCallum,
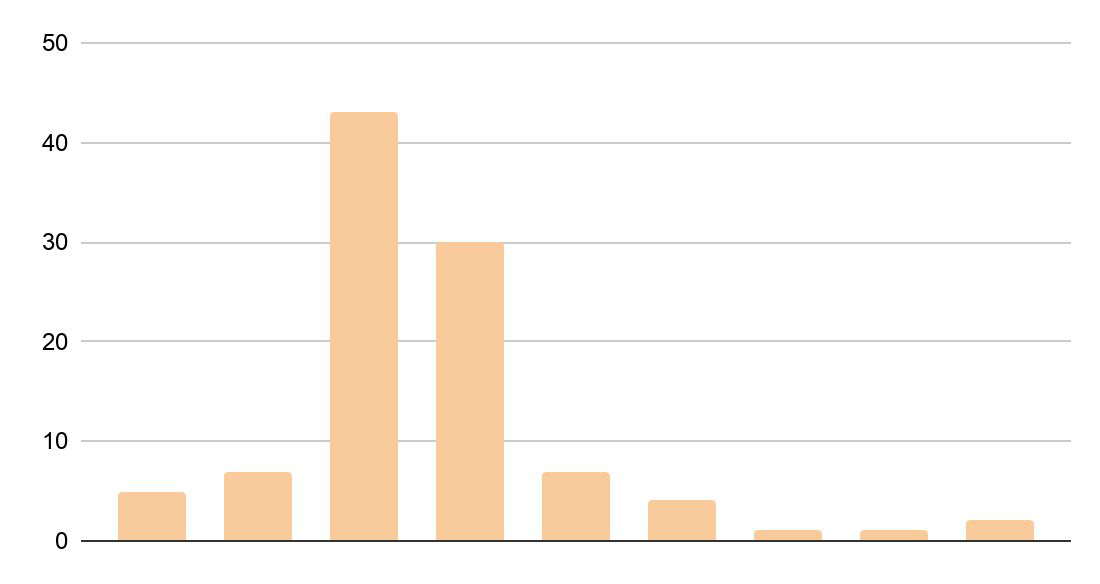