ProtoQA: A Question Answering Dataset for Prototypical Common-Sense Reasoning
Michael Boratko, Xiang Li, Tim O'Gorman, Rajarshi Das, Dan Le, Andrew McCallum
Question Answering Long Paper
You can open the pre-recorded video in a separate window.
Abstract:
Given questions regarding some prototypical situation --- such as Name something that people usually do before they leave the house for work? --- a human can easily answer them via acquired experiences. There can be multiple right answers for such questions, with some more common for a situation than others. This paper introduces a new question answering dataset for training and evaluating common sense reasoning capabilities of artificial intelligence systems in such prototypical situations. The training set is gathered from an existing set of questions played in a long-running international trivia game show -- Family Feud. The hidden evaluation set is created by gathering answers for each question from 100 crowd-workers. We also propose a generative evaluation task where a model has to output a ranked list of answers, ideally covering all prototypical answers for a question. After presenting multiple competitive baseline models, we find that human performance still exceeds model scores on all evaluation metrics with a meaningful gap, supporting the challenging nature of the task.
NOTE: Video may display a random order of authors.
Correct author list is at the top of this page.
Connected Papers in EMNLP2020
Similar Papers
What Does My QA Model Know? Devising Controlled Probes using Expert
Kyle Richardson, Ashish Sabharwal,

Unsupervised Question Decomposition for Question Answering
Ethan Perez, Patrick Lewis, Wen-tau Yih, Kyunghyun Cho, Douwe Kiela,

Inquisitive Question Generation for High Level Text Comprehension
Wei-Jen Ko, Te-yuan Chen, Yiyan Huang, Greg Durrett, Junyi Jessy Li,
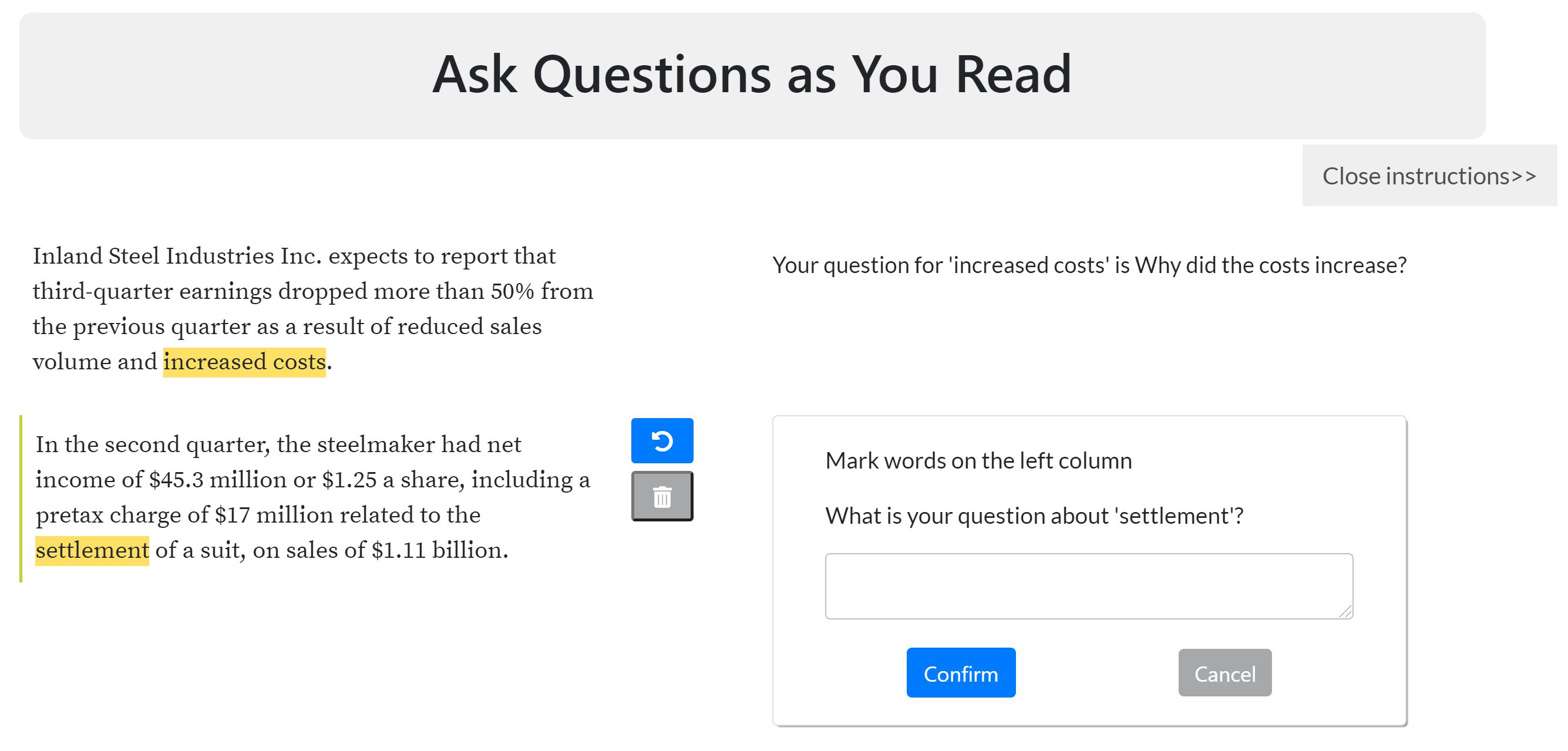