BERT-EMD: Many-to-Many Layer Mapping for BERT Compression with Earth Mover's Distance
Jianquan Li, Xiaokang Liu, Honghong Zhao, Ruifeng Xu, Min Yang, Yaohong Jin
NLP Applications Long Paper
You can open the pre-recorded video in a separate window.
Abstract:
Pre-trained language models (e.g., BERT) have achieved significant success in various natural language processing (NLP) tasks. However, high storage and computational costs obstruct pre-trained language models to be effectively deployed on resource-constrained devices. In this paper, we propose a novel BERT distillation method based on many-to-many layer mapping, which allows each intermediate student layer to learn from any intermediate teacher layers. In this way, our model can learn from different teacher layers adaptively for different NLP tasks. In addition, we leverage Earth Mover's Distance (EMD) to compute the minimum cumulative cost that must be paid to transform knowledge from teacher network to student network. EMD enables effective matching for the many-to-many layer mapping. Furthermore, we propose a cost attention mechanism to learn the layer weights used in EMD automatically, which is supposed to further improve the model's performance and accelerate convergence time. Extensive experiments on GLUE benchmark demonstrate that our model achieves competitive performance compared to strong competitors in terms of both accuracy and model compression
NOTE: Video may display a random order of authors.
Correct author list is at the top of this page.
Connected Papers in EMNLP2020
Similar Papers
Contrastive Distillation on Intermediate Representations for Language Model Compression
Siqi Sun, Zhe Gan, Yuwei Fang, Yu Cheng, Shuohang Wang, Jingjing Liu,
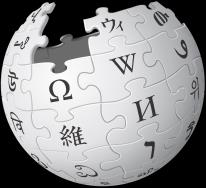
TernaryBERT: Distillation-aware Ultra-low Bit BERT
Wei Zhang, Lu Hou, Yichun Yin, Lifeng Shang, Xiao Chen, Xin Jiang, Qun Liu,
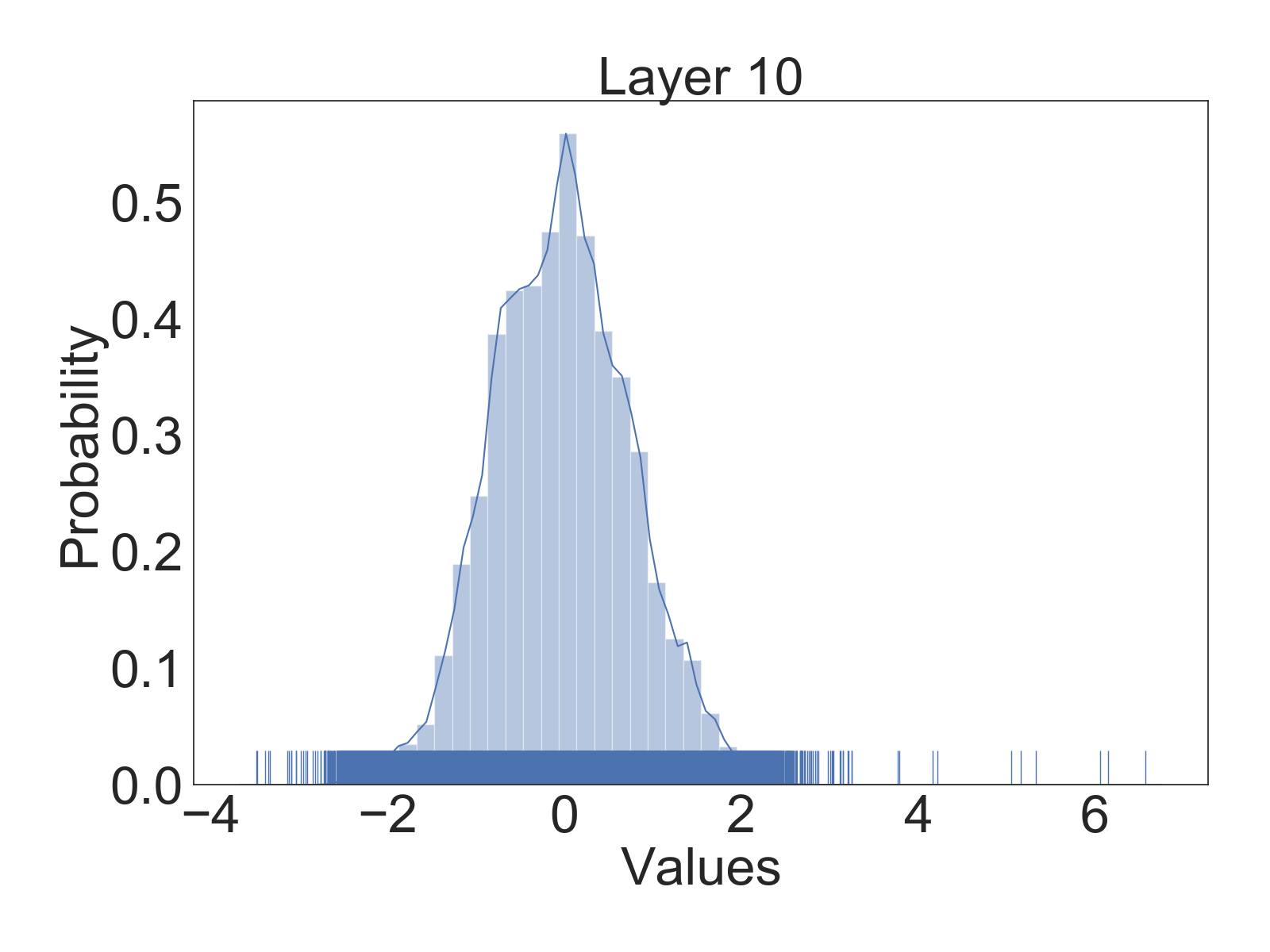
POINTER: Constrained Progressive Text Generation via Insertion-based Generative Pre-training
Yizhe Zhang, Guoyin Wang, Chunyuan Li, Zhe Gan, Chris Brockett, Bill Dolan,
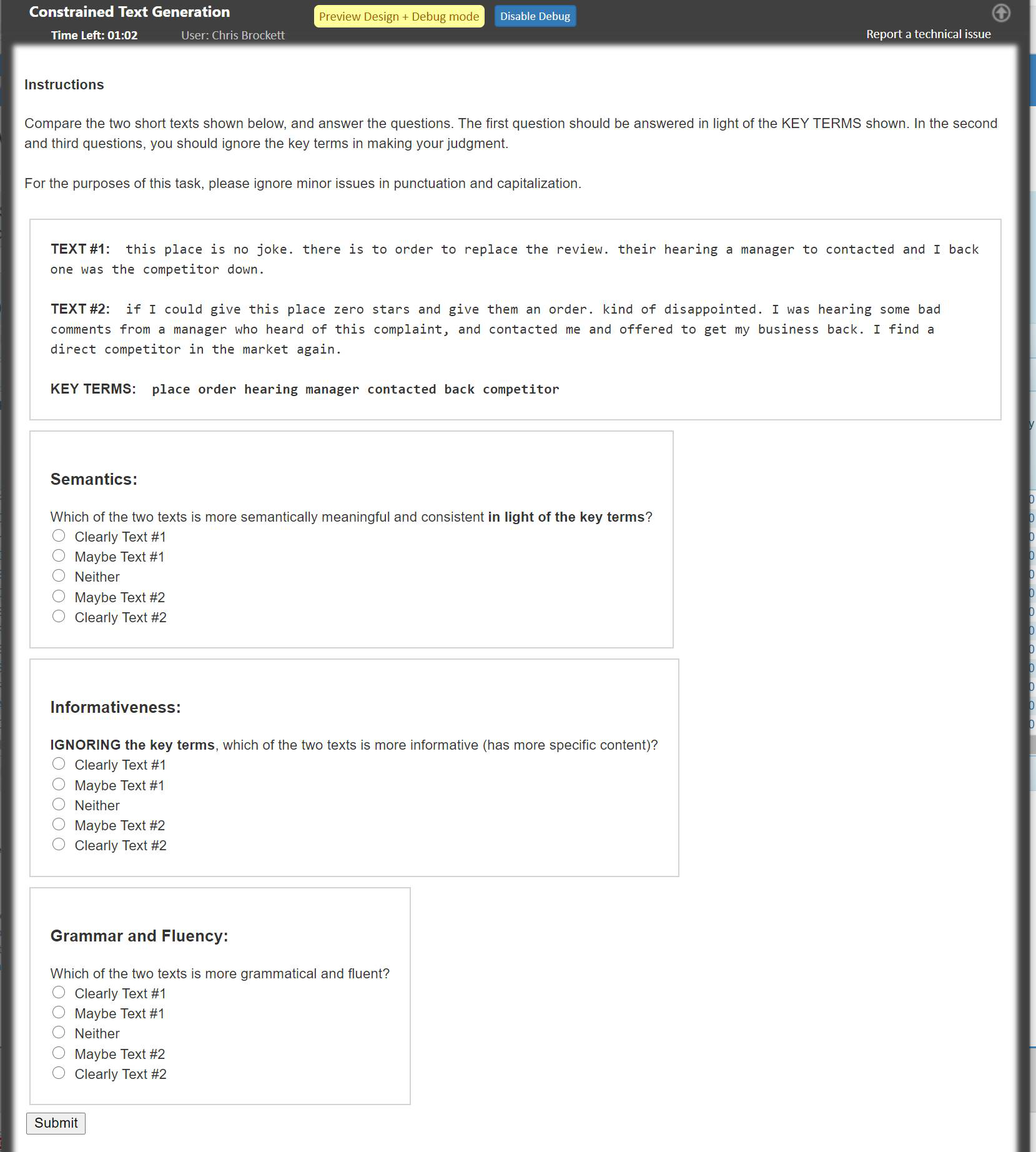
oLMpics - On what Language Model Pre-training Captures
Alon Talmor, Yanai Elazar, Yoav Goldberg, Jonathan Berant,
