Information-Theoretic Probing with Minimum Description Length
Elena Voita, Ivan Titov
Interpretability and Analysis of Models for NLP Long Paper
You can open the pre-recorded video in a separate window.
Abstract:
To measure how well pretrained representations encode some linguistic property, it is common to use accuracy of a probe, i.e. a classifier trained to predict the property from the representations. Despite widespread adoption of probes, differences in their accuracy fail to adequately reflect differences in representations. For example, they do not substantially favour pretrained representations over randomly initialized ones. Analogously, their accuracy can be similar when probing for genuine linguistic labels and probing for random synthetic tasks. To see reasonable differences in accuracy with respect to these random baselines, previous work had to constrain either the amount of probe training data or its model size. Instead, we propose an alternative to the standard probes, information-theoretic probing with minimum description length (MDL). With MDL probing, training a probe to predict labels is recast as teaching it to effectively transmit the data. Therefore, the measure of interest changes from probe accuracy to the description length of labels given representations. In addition to probe quality, the description length evaluates "the amount of effort" needed to achieve the quality. This amount of effort characterizes either (i) size of a probing model, or (ii) the amount of data needed to achieve the high quality. We consider two methods for estimating MDL which can be easily implemented on top of the standard probing pipelines: variational coding and online coding. We show that these methods agree in results and are more informative and stable than the standard probes.
NOTE: Video may display a random order of authors.
Correct author list is at the top of this page.
Connected Papers in EMNLP2020
Similar Papers
Pareto Probing: Trading Off Accuracy for Complexity
Tiago Pimentel, Naomi Saphra, Adina Williams, Ryan Cotterell,
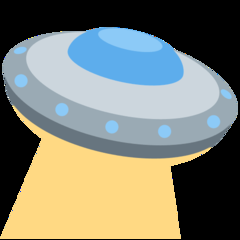
Methods for Numeracy-Preserving Word Embeddings
Dhanasekar Sundararaman, Shijing Si, Vivek Subramanian, Guoyin Wang, Devamanyu Hazarika, Lawrence Carin,
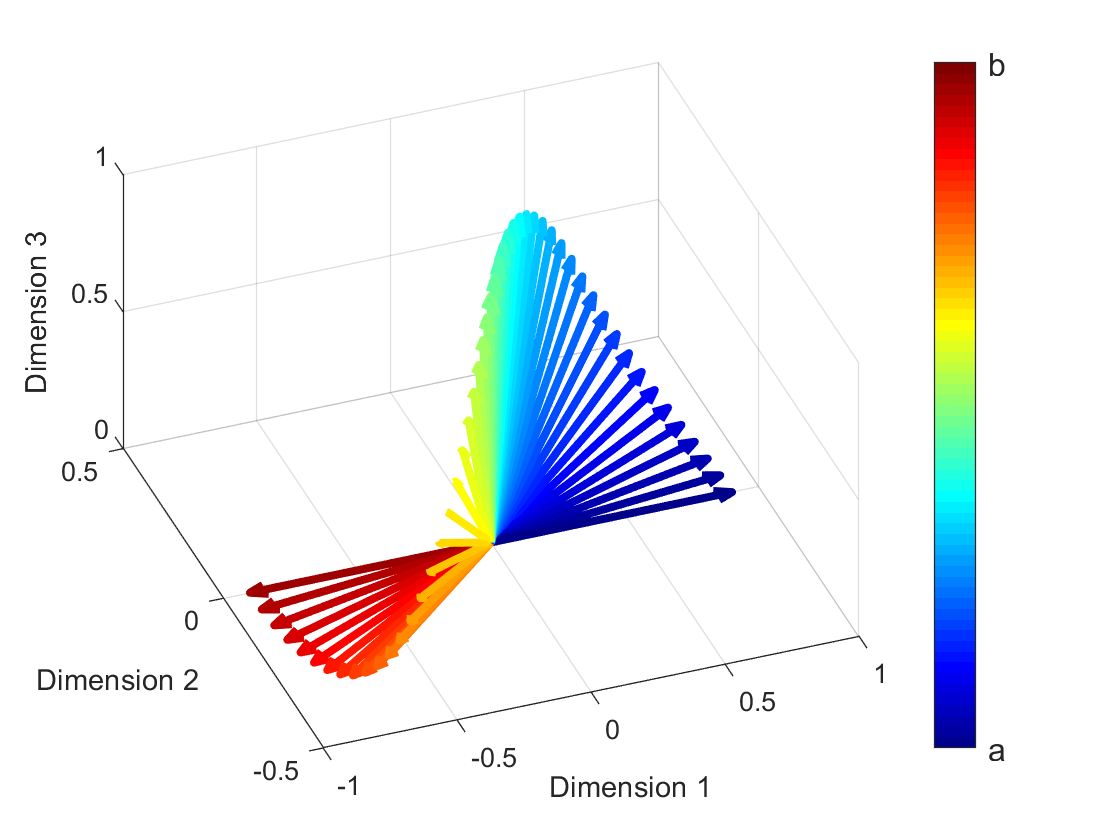
Learning Which Features Matter: RoBERTa Acquires a Preference for Linguistic Generalizations (Eventually)
Alex Warstadt, Yian Zhang, Xiaocheng Li, Haokun Liu, Samuel R. Bowman,
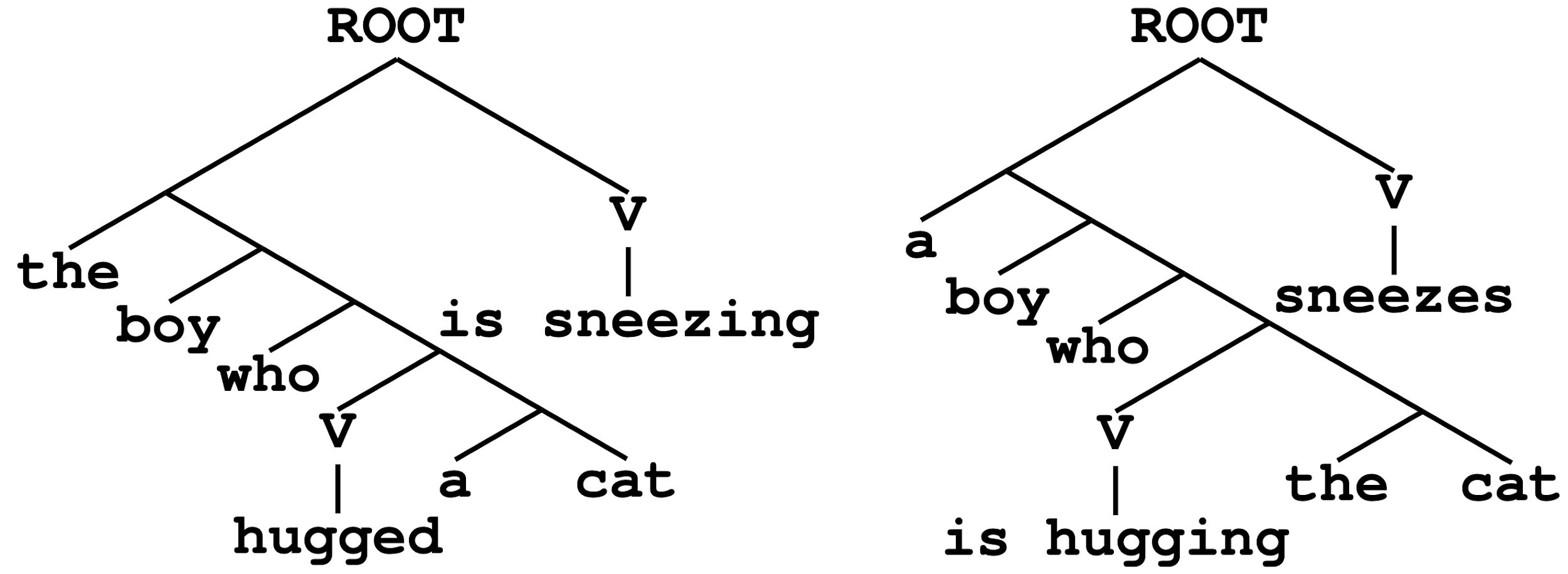