Repulsive Attention: Rethinking Multi-head Attention as Bayesian Inference
Bang An, Jie Lyu, Zhenyi Wang, Chunyuan Li, Changwei Hu, Fei Tan, Ruiyi Zhang, Yifan Hu, Changyou Chen
Machine Learning for NLP Long Paper
You can open the pre-recorded video in a separate window.
Abstract:
The neural attention mechanism plays an important role in many natural language processing applications. In particular, multi-head attention extends single-head attention by allowing a model to jointly attend information from different perspectives. However, without explicit constraining, multi-head attention may suffer from attention collapse, an issue that makes different heads extract similar attentive features, thus limiting the model's representation power. In this paper, for the first time, we provide a novel understanding of multi-head attention from a Bayesian perspective. Based on the recently developed particle-optimization sampling techniques, we propose a non-parametric approach that explicitly improves the repulsiveness in multi-head attention and consequently strengthens model's expressiveness. Remarkably, our Bayesian interpretation provides theoretical inspirations on the not-well-understood questions: why and how one uses multi-head attention. Extensive experiments on various attention models and applications demonstrate that the proposed repulsive attention can improve the learned feature diversity, leading to more informative representations with consistent performance improvement on multiple tasks.
NOTE: Video may display a random order of authors.
Correct author list is at the top of this page.
Connected Papers in EMNLP2020
Similar Papers
Recall and Learn: Fine-tuning Deep Pretrained Language Models with Less Forgetting
Sanyuan Chen, Yutai Hou, Yiming Cui, Wanxiang Che, Ting Liu, Xiangzhan Yu,
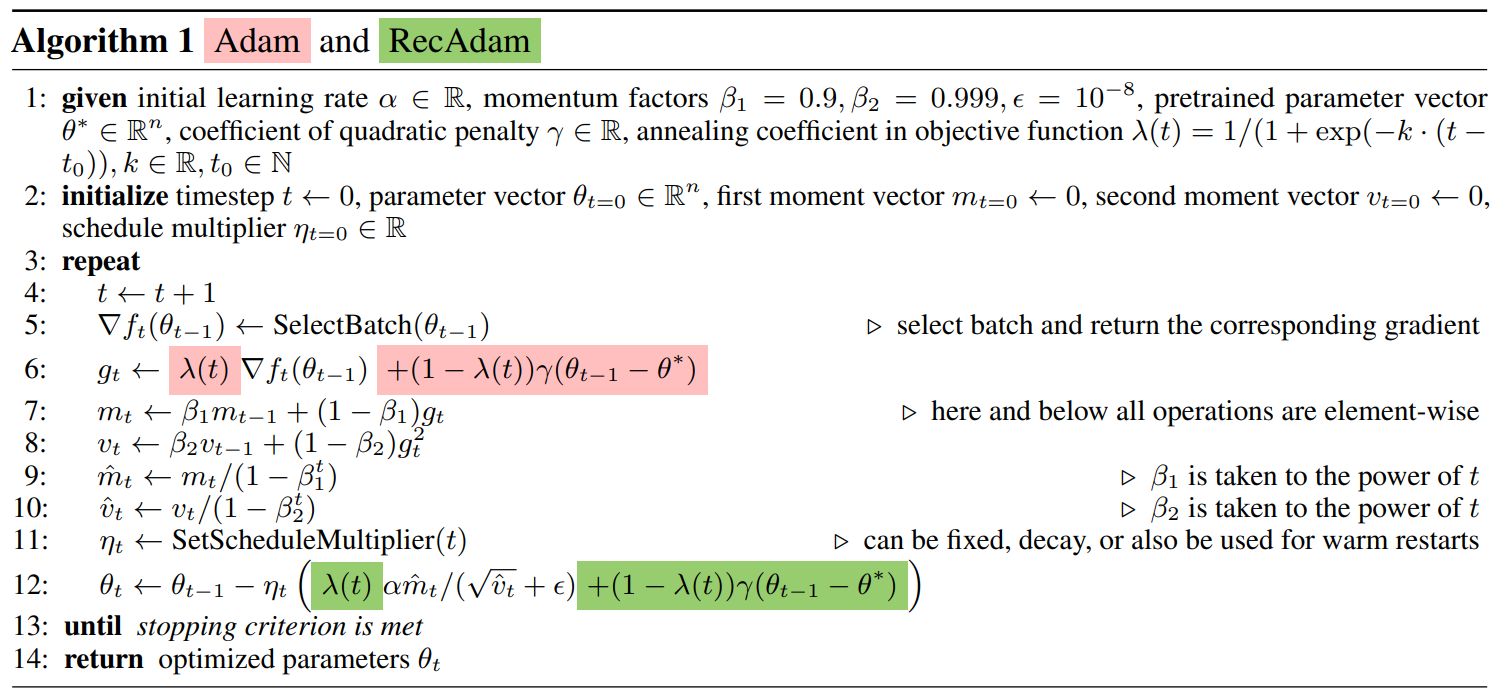
Does my multimodal model learn cross-modal interactions? It's harder to tell than you might think!
Jack Hessel, Lillian Lee,
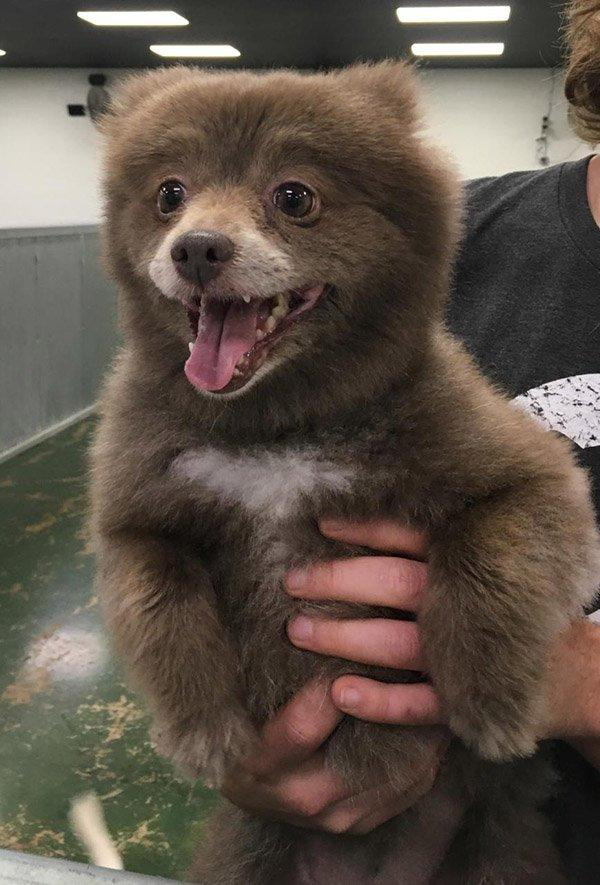
VD-BERT: A Unified Vision and Dialog Transformer with BERT
Yue Wang, Shafiq Joty, Michael Lyu, Irwin King, Caiming Xiong, Steven C.H. Hoi,
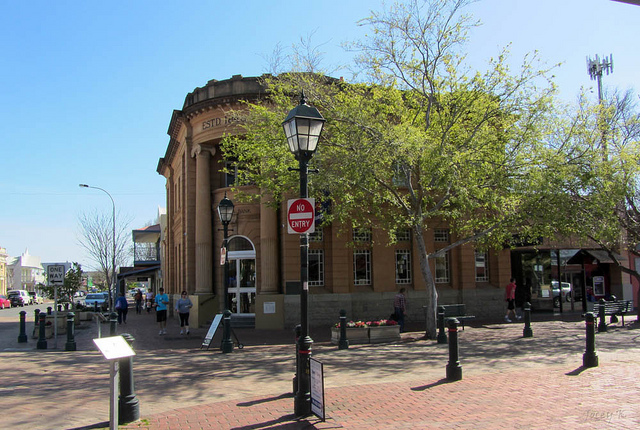
Understanding Neural Abstractive Summarization Models via Uncertainty
Jiacheng Xu, Shrey Desai, Greg Durrett,
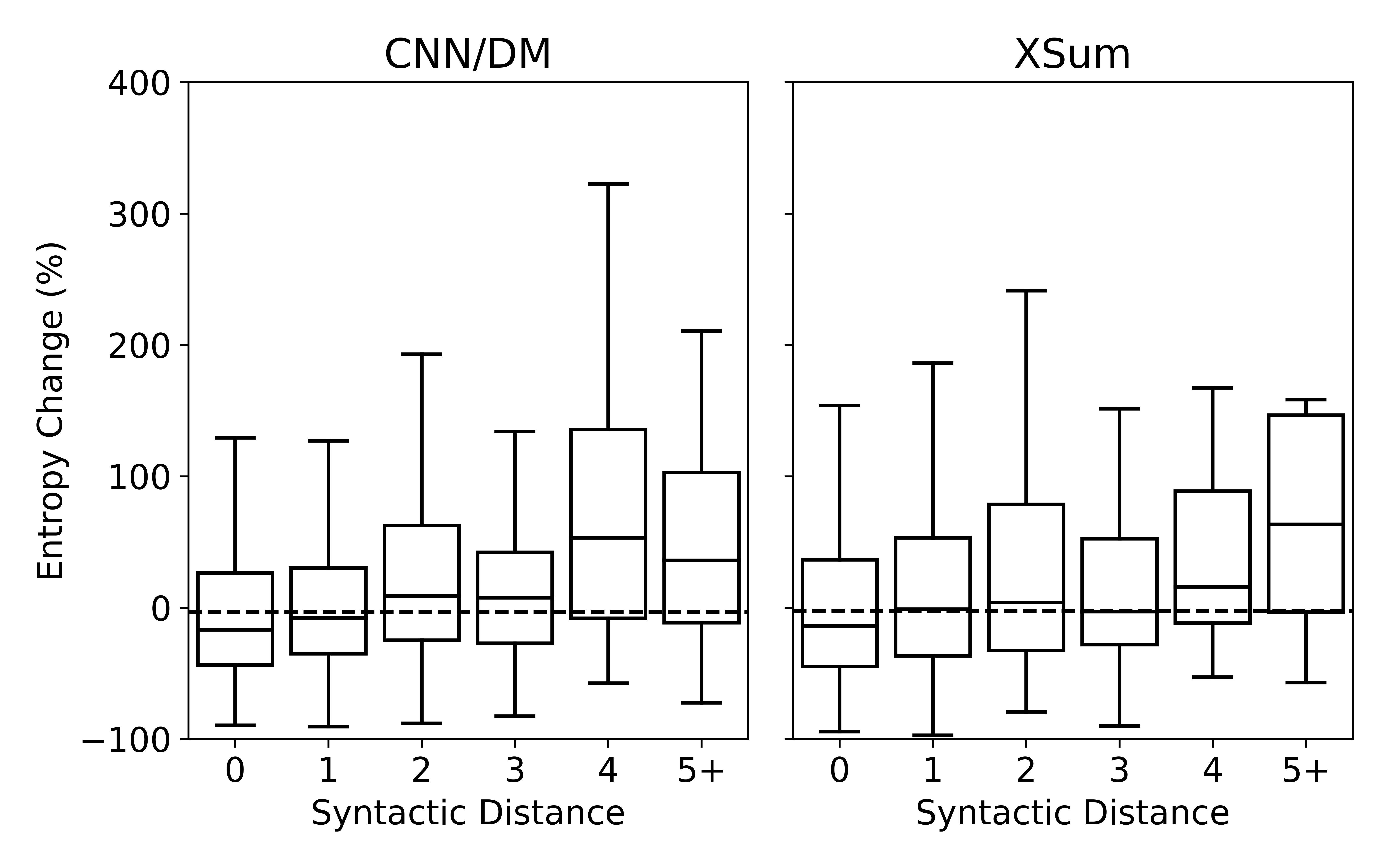