Does my multimodal model learn cross-modal interactions? It's harder to tell than you might think!
Jack Hessel, Lillian Lee
Language Grounding to Vision, Robotics and Beyond Long Paper
You can open the pre-recorded video in a separate window.
Abstract:
Modeling expressive cross-modal interactions seems crucial in multimodal tasks, such as visual question answering. However, sometimes high-performing black-box algorithms turn out to be mostly exploiting unimodal signals in the data. We propose a new diagnostic tool, empirical multimodally-additive function projection (EMAP), for isolating whether or not cross-modal interactions improve performance for a given model on a given task. This function projection modifies model predictions so that cross-modal interactions are eliminated, isolating the additive, unimodal structure. For seven image+text classification tasks (on each of which we set new state-of-the-art benchmarks), we find that, in many cases, removing cross-modal interactions results in little to no performance degradation. Surprisingly, this holds even when expressive models, with capacity to consider interactions, otherwise outperform less expressive models; thus, performance improvements, even when present, often cannot be attributed to consideration of cross-modal feature interactions. We hence recommend that researchers in multimodal machine learning report the performance not only of unimodal baselines, but also the EMAP of their best-performing model.
NOTE: Video may display a random order of authors.
Correct author list is at the top of this page.
Connected Papers in EMNLP2020
Similar Papers
X-LXMERT: Paint, Caption and Answer Questions with Multi-Modal Transformers
Jaemin Cho, Jiasen Lu, Dustin Schwenk, Hannaneh Hajishirzi, Aniruddha Kembhavi,
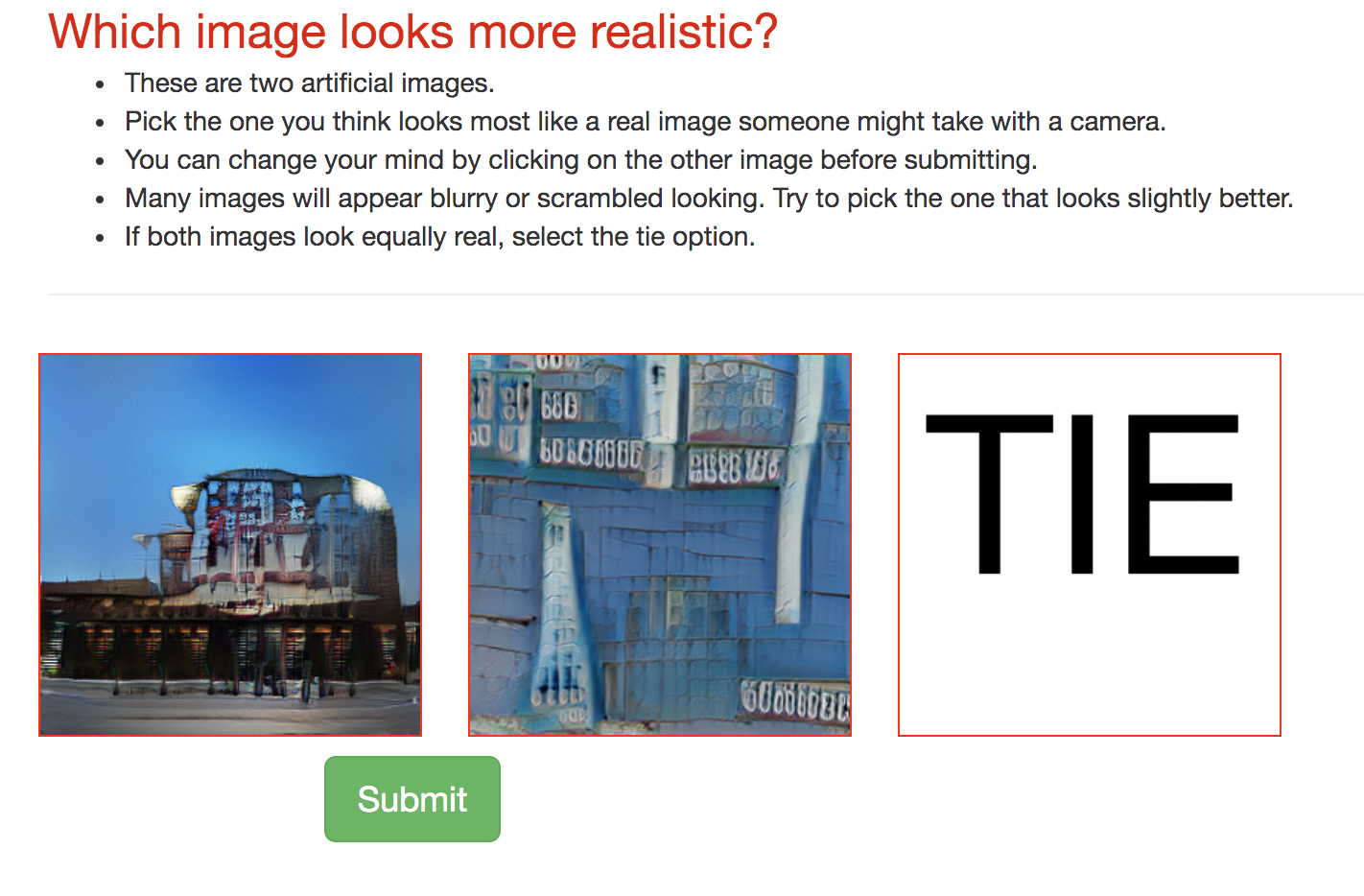
Multimodal Routing: Improving Local and Global Interpretability of Multimodal Language Analysis
Yao-Hung Hubert Tsai, Martin Ma, Muqiao Yang, Ruslan Salakhutdinov, Louis-Philippe Morency,
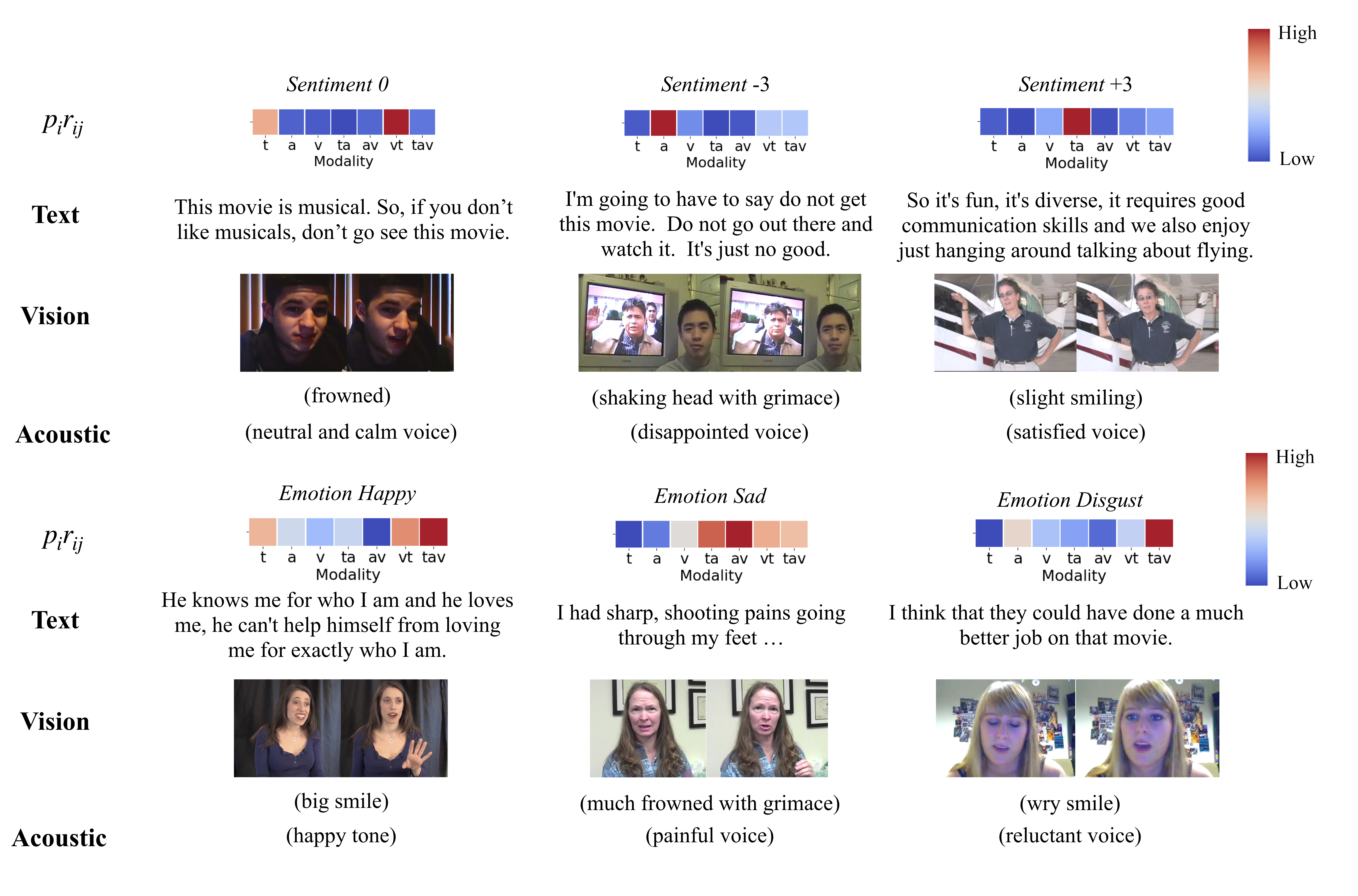
oLMpics - On what Language Model Pre-training Captures
Alon Talmor, Yanai Elazar, Yoav Goldberg, Jonathan Berant,

The World is Not Binary: Learning to Rank with Grayscale Data for Dialogue Response Selection
Zibo Lin, Deng Cai, Yan Wang, Xiaojiang Liu, Haitao Zheng, Shuming Shi,
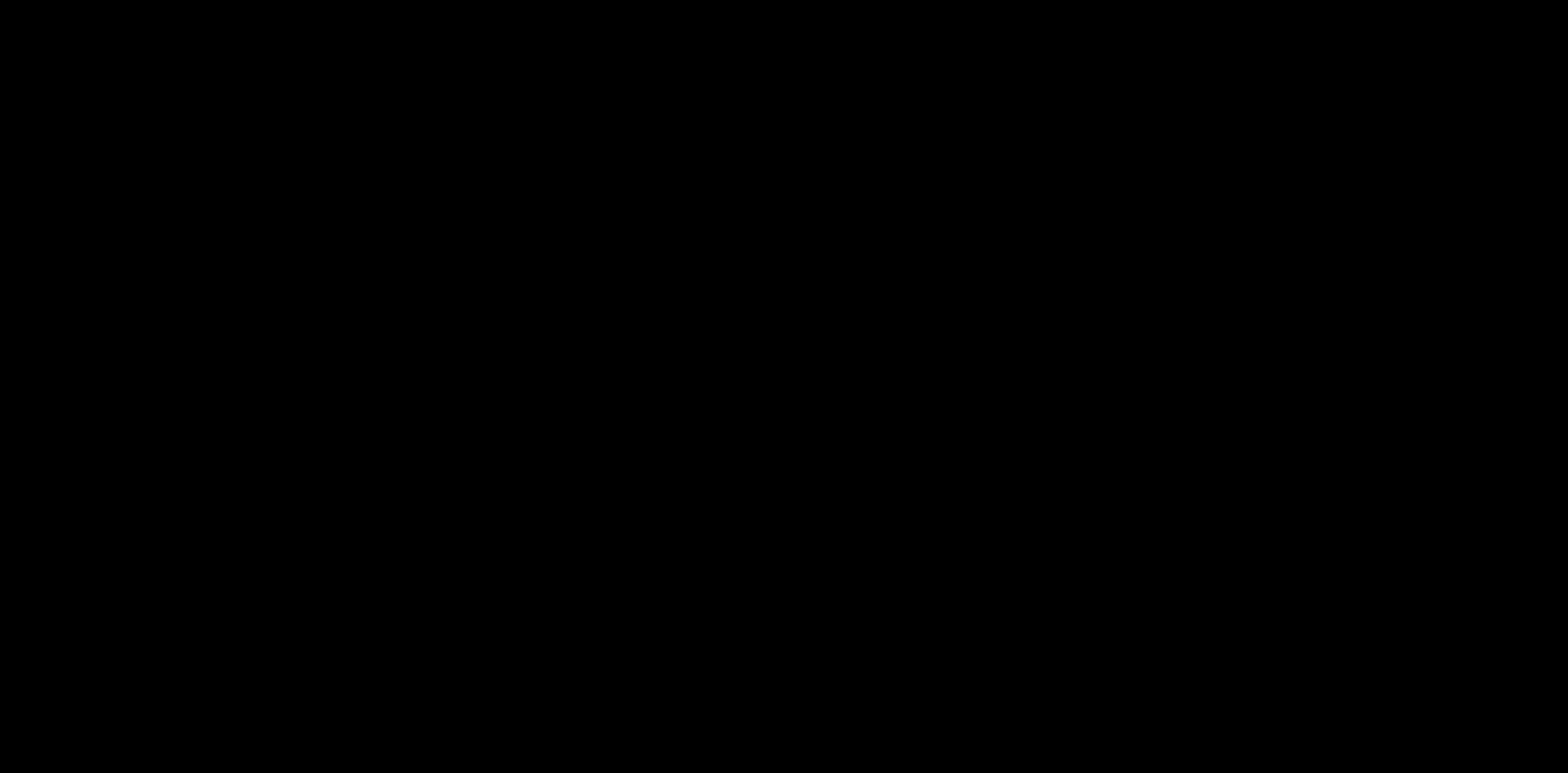