Uncertainty-Aware Semantic Augmentation for Neural Machine Translation
Xiangpeng Wei, Heng Yu, Yue Hu, Rongxiang Weng, Luxi Xing, Weihua Luo
Machine Translation and Multilinguality Long Paper
You can open the pre-recorded video in a separate window.
Abstract:
As a sequence-to-sequence generation task, neural machine translation (NMT) naturally contains intrinsic uncertainty, where a single sentence in one language has multiple valid counterparts in the other. However, the dominant methods for NMT only observe one of them from the parallel corpora for the model training but have to deal with adequate variations under the same meaning at inference. This leads to a discrepancy of the data distribution between the training and the inference phases. To address this problem, we propose uncertainty-aware semantic augmentation, which explicitly captures the universal semantic information among multiple semantically-equivalent source sentences and enhances the hidden representations with this information for better translations. Extensive experiments on various translation tasks reveal that our approach significantly outperforms the strong baselines and the existing methods.
NOTE: Video may display a random order of authors.
Correct author list is at the top of this page.
Connected Papers in EMNLP2020
Similar Papers
Language Model Prior for Low-Resource Neural Machine Translation
Christos Baziotis, Barry Haddow, Alexandra Birch,
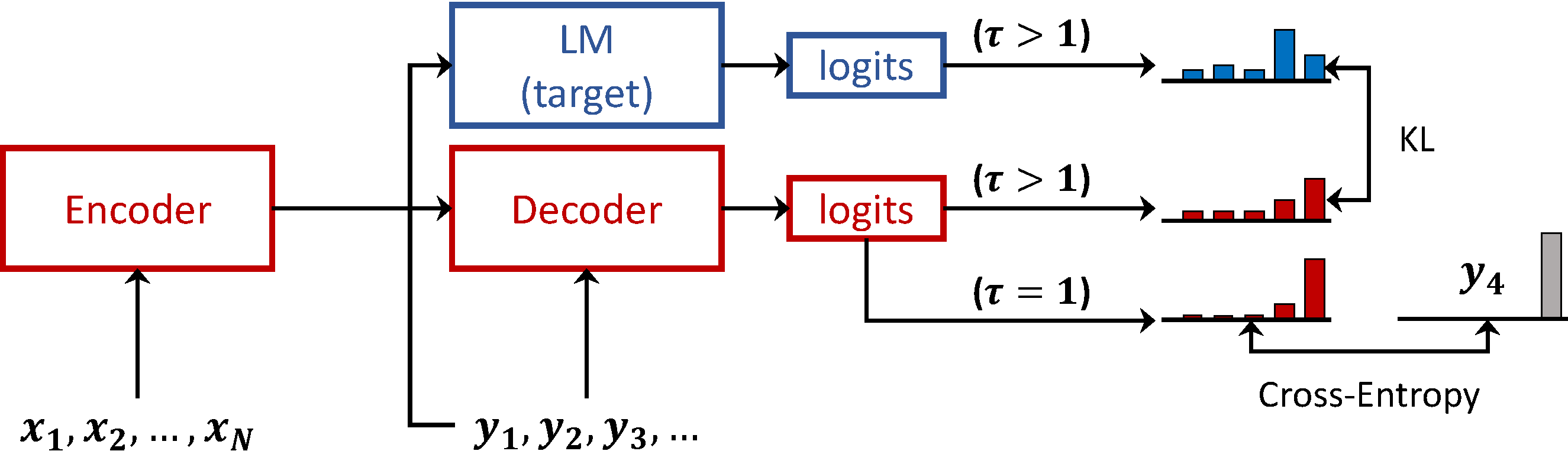
Simulated multiple reference training improves low-resource machine translation
Huda Khayrallah, Brian Thompson, Matt Post, Philipp Koehn,

Incomplete Utterance Rewriting as Semantic Segmentation
Qian Liu, Bei Chen, Jian-Guang Lou, Bin Zhou, Dongmei Zhang,
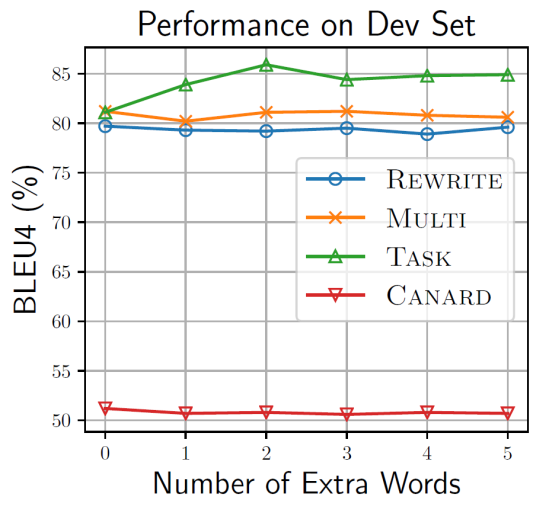
On the Sparsity of Neural Machine Translation Models
Yong Wang, Longyue Wang, Victor Li, Zhaopeng Tu,
