Making Monolingual Sentence Embeddings Multilingual using Knowledge Distillation
Nils Reimers, Iryna Gurevych
Machine Translation and Multilinguality Long Paper
You can open the pre-recorded video in a separate window.
Abstract:
We present an easy and efficient method to extend existing sentence embedding models to new languages. This allows to create multilingual versions from previously monolingual models. The training is based on the idea that a translated sentence should be mapped to the same location in the vector space as the original sentence. We use the original (monolingual) model to generate sentence embeddings for the source language and then train a new system on translated sentences to mimic the original model. Compared to other methods for training multilingual sentence embeddings, this approach has several advantages: It is easy to extend existing models with relatively few samples to new languages, it is easier to ensure desired properties for the vector space, and the hardware requirements for training are lower. We demonstrate the effectiveness of our approach for 50+ languages from various language families. Code to extend sentence embeddings models to more than 400 languages is publicly available.
NOTE: Video may display a random order of authors.
Correct author list is at the top of this page.
Connected Papers in EMNLP2020
Similar Papers
Pre-training Multilingual Neural Machine Translation by Leveraging Alignment Information
Zehui Lin, Xiao Pan, Mingxuan Wang, Xipeng Qiu, Jiangtao Feng, Hao Zhou, Lei Li,

Reusing a Pretrained Language Model on Languages with Limited Corpora for Unsupervised NMT
Alexandra Chronopoulou, Dario Stojanovski, Alexander Fraser,
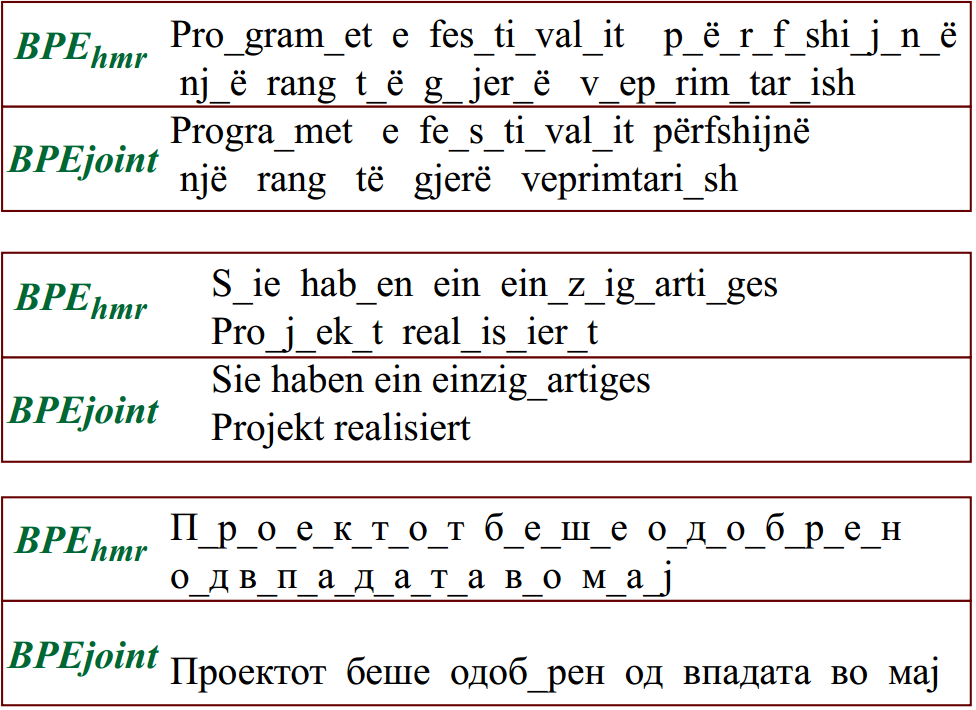
Improving Multilingual Models with Language-Clustered Vocabularies
Hyung Won Chung, Dan Garrette, Kiat Chuan Tan, Jason Riesa,
