Transformer Based Multi-Source Domain Adaptation
Dustin Wright, Isabelle Augenstein
Machine Learning for NLP Long Paper
You can open the pre-recorded video in a separate window.
Abstract:
In practical machine learning settings, the data on which a model must make predictions often come from a different distribution than the data it was trained on. Here, we investigate the problem of unsupervised multi-source domain adaptation, where a model is trained on labelled data from multiple source domains and must make predictions on a domain for which no labelled data has been seen. Prior work with CNNs and RNNs has demonstrated the benefit of mixture of experts, where the predictions of multiple domain expert classifiers are combined; as well as domain adversarial training, to induce a domain agnostic representation space. Inspired by this, we investigate how such methods can be effectively applied to large pretrained transformer models. We find that domain adversarial training has an effect on the learned representations of these models while having little effect on their performance, suggesting that large transformer-based models are already relatively robust across domains. Additionally, we show that mixture of experts leads to significant performance improvements by comparing several variants of mixing functions, including one novel metric based on attention. Finally, we demonstrate that the predictions of large pretrained transformer based domain experts are highly homogenous, making it challenging to learn effective metrics for mixing their predictions.
NOTE: Video may display a random order of authors.
Correct author list is at the top of this page.
Connected Papers in EMNLP2020
Similar Papers
SSMBA: Self-Supervised Manifold Based Data Augmentation for Improving Out-of-Domain Robustness
Nathan Ng, Kyunghyun Cho, Marzyeh Ghassemi,
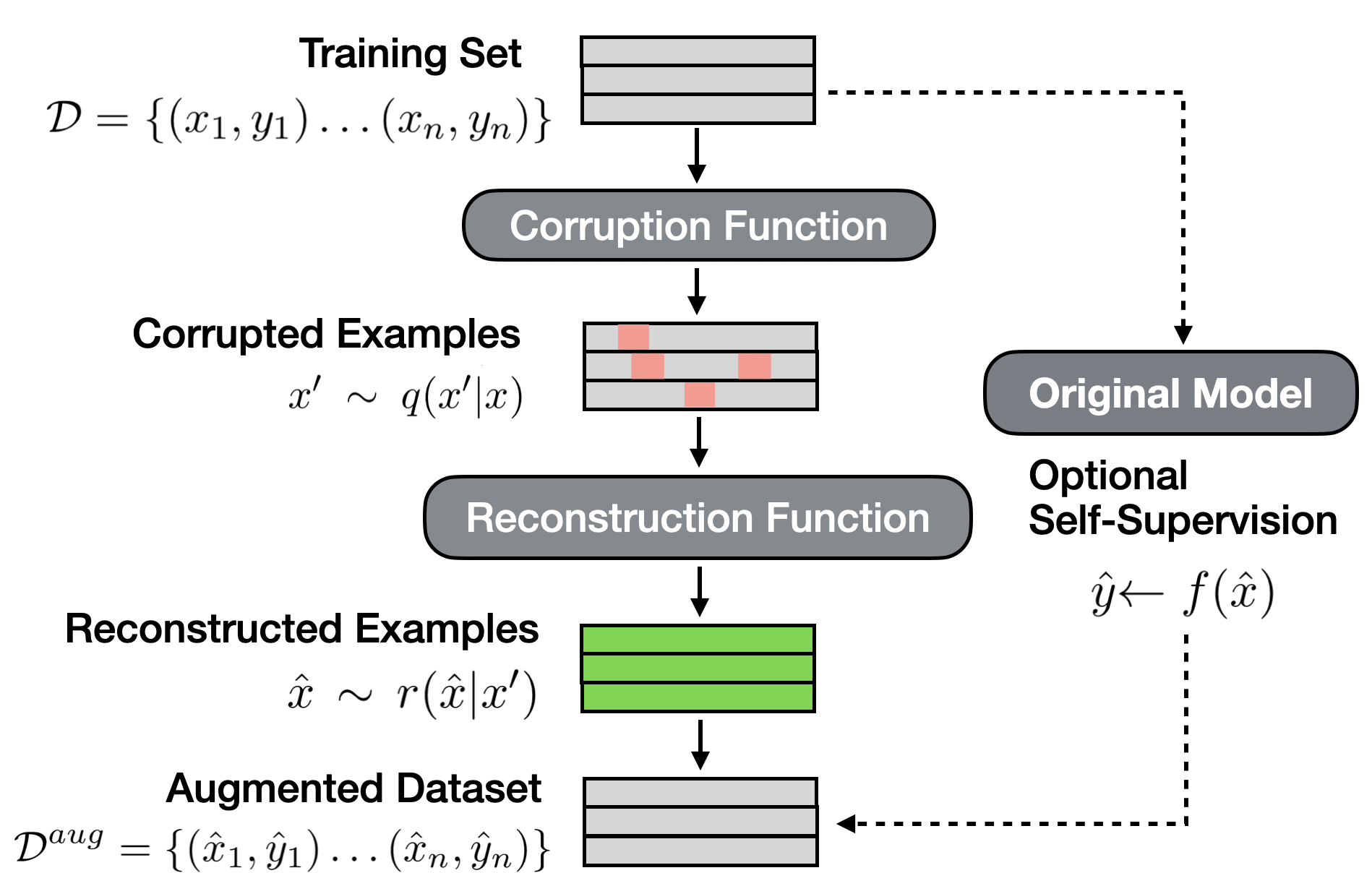
Improving Dialog Evaluation with a Multi-reference Adversarial Dataset and Large Scale Pretraining
Ananya Sai, Akash Mohan Kumar, Siddhartha Arora, Mitesh Khapra,

Feature Adaptation of Pre-Trained Language Models across Languages and Domains with Robust Self-Training
Hai Ye, Qingyu Tan, Ruidan He, Juntao Li, Hwee Tou Ng, Lidong Bing,

Active Learning for BERT: An Empirical Study
Liat Ein-Dor, Alon Halfon, Ariel Gera, Eyal Shnarch, Lena Dankin, Leshem Choshen, Marina Danilevsky, Ranit Aharonov, Yoav Katz, Noam Slonim,
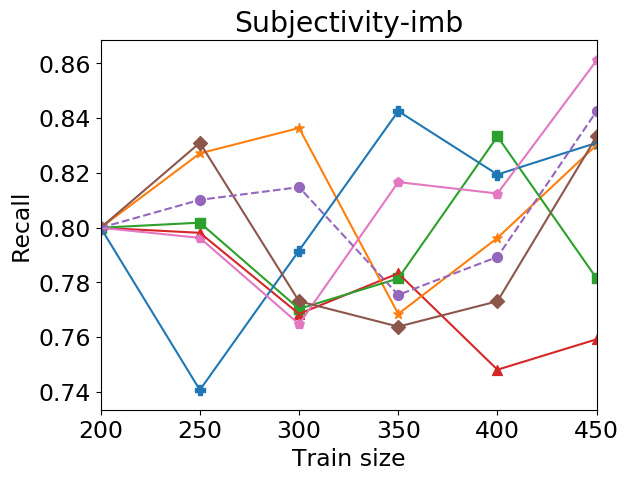