Feature Adaptation of Pre-Trained Language Models across Languages and Domains with Robust Self-Training
Hai Ye, Qingyu Tan, Ruidan He, Juntao Li, Hwee Tou Ng, Lidong Bing
Sentiment Analysis, Stylistic Analysis, and Argument Mining Long Paper
You can open the pre-recorded video in a separate window.
Abstract:
Adapting pre-trained language models (PrLMs) (e.g., BERT) to new domains has gained much attention recently. Instead of fine-tuning PrLMs as done in most previous work, we investigate how to adapt the features of PrLMs to new domains without fine-tuning. We explore unsupervised domain adaptation (UDA) in this paper. With the features from PrLMs, we adapt the models trained with labeled data from the source domain to the unlabeled target domain. Self-training is widely used for UDA, and it predicts pseudo labels on the target domain data for training. However, the predicted pseudo labels inevitably include noise, which will negatively affect training a robust model. To improve the robustness of self-training, in this paper we present class-aware feature self-distillation (CFd) to learn discriminative features from PrLMs, in which PrLM features are self-distilled into a feature adaptation module and the features from the same class are more tightly clustered. We further extend CFd to a cross-language setting, in which language discrepancy is studied. Experiments on two monolingual and multilingual Amazon review datasets show that CFd can consistently improve the performance of self-training in cross-domain and cross-language settings.
NOTE: Video may display a random order of authors.
Correct author list is at the top of this page.
Connected Papers in EMNLP2020
Similar Papers
Learning Which Features Matter: RoBERTa Acquires a Preference for Linguistic Generalizations (Eventually)
Alex Warstadt, Yian Zhang, Xiaocheng Li, Haokun Liu, Samuel R. Bowman,
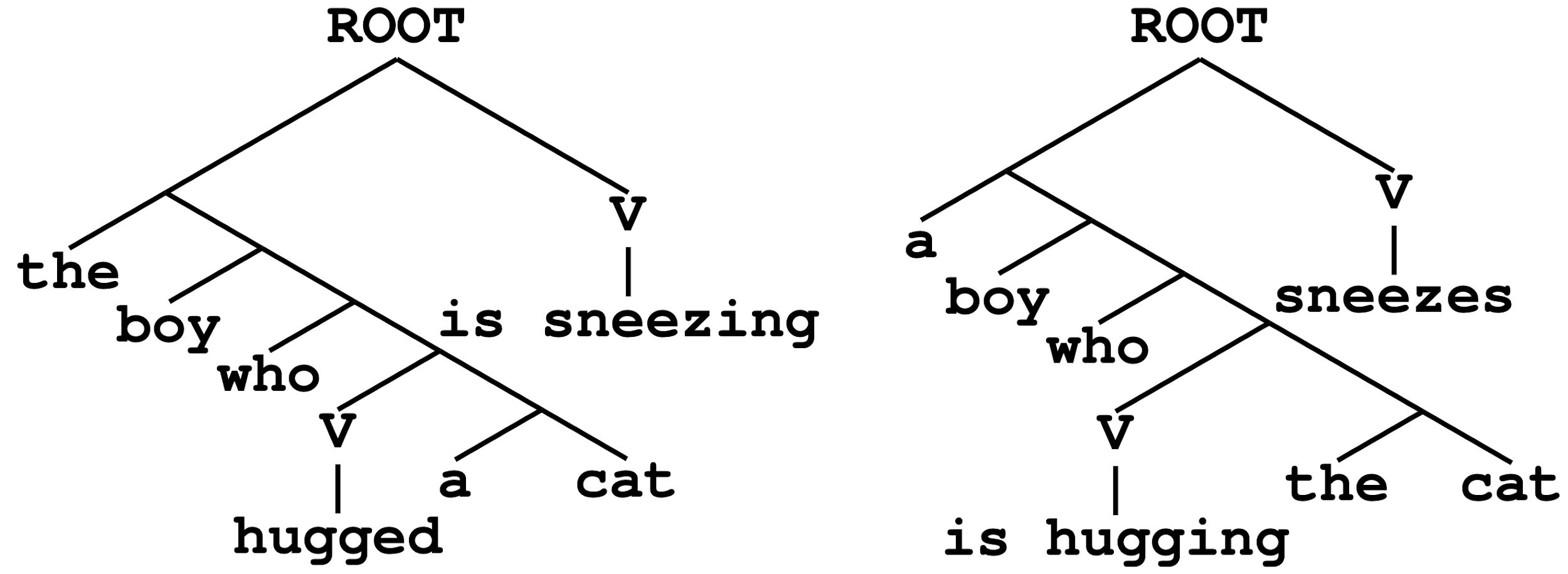
Reusing a Pretrained Language Model on Languages with Limited Corpora for Unsupervised NMT
Alexandra Chronopoulou, Dario Stojanovski, Alexander Fraser,
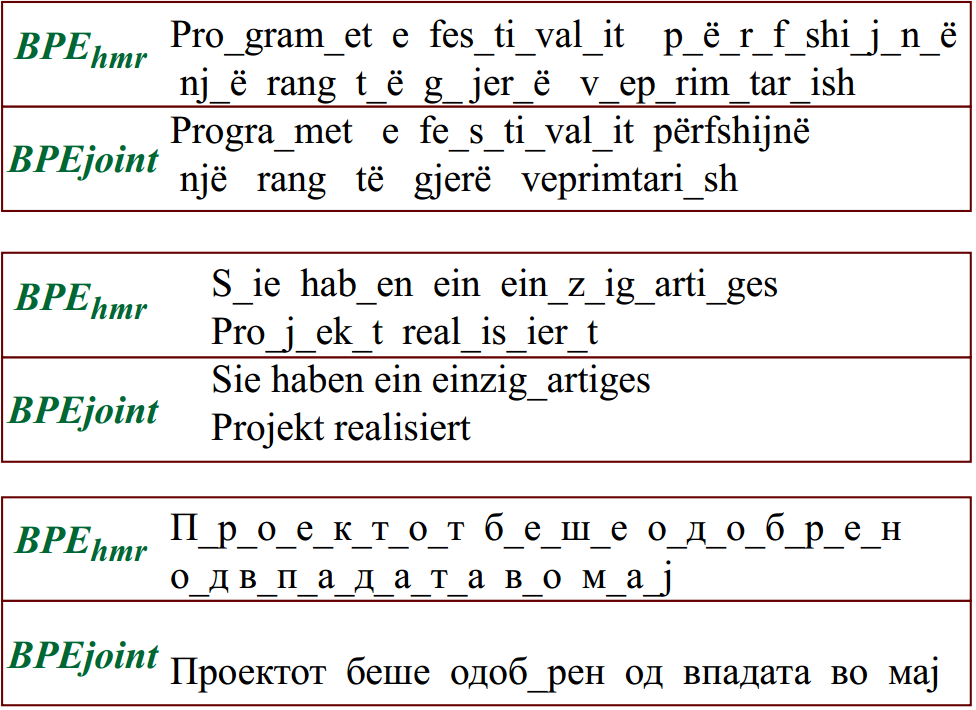
An Empirical Investigation Towards Efficient Multi-Domain Language Model Pre-training
Kristjan Arumae, Qing Sun, Parminder Bhatia,
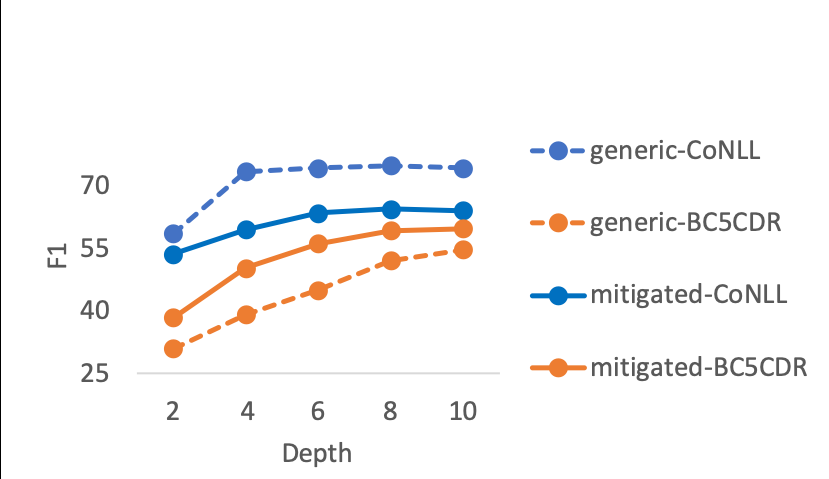